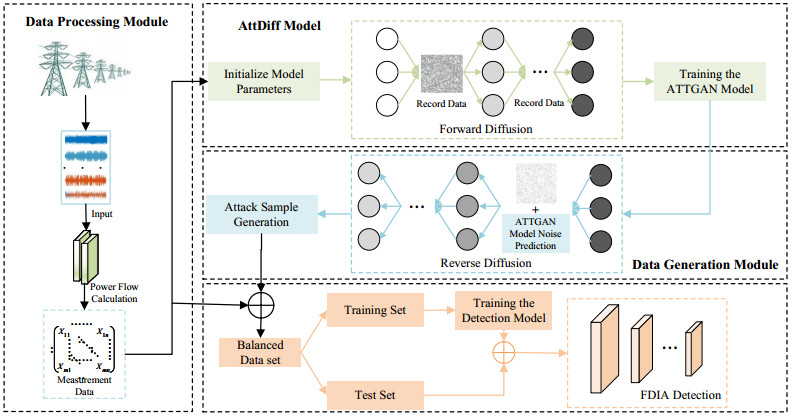
A false data injection attack (FDIA) indicates that attackers mislead system decisions by inputting false or tampered data into the system, which seriously threatens the security of power cyber-physical systems. Considering the scarcity of FDIA attack samples, the traditional FDIA detection models based on neural networks are always limited in their detection capabilities due to imbalanced training samples. To address this problem, this paper proposes an efficient FDIA attack sample generation method by an adversarial attention-diffusion model. The proposed scheme consists of a diffusion model and a GAN model with an attention mechanism (ATTGAN). First, the forward diffusion of the diffusion model was used to add noise to the real data while injecting the attack vector. Then, the ATTGAN model was trained to effectively focus on the information of power grid measurements and topological nodes, while weakening irrelevant information. In the reverse diffusion process, the trained ATTGAN model was combined to predict the noise, and it was further iterated forward step by step and denoised in this process. Finally, a large number of efficient FDIA attack samples can were generated. Extensive experiments have been carried out on IEEE 14, IEEE 39, and IEEE 118 bus systems. The experimental results indicate that the generated attack samples outperform existing state-of-the-art schemes in terms of evasion detection capability, robustness, and attack strength.
虚假数据注入攻击(FDIA)是指攻击者通过向系统输入虚假或篡改的数据,误导系统决策,严重威胁电力信息物理系统的安全。考虑到FDIA攻击样本的稀缺性,传统的基于神经网络的FDIA检测模型总是受到训练样本不平衡的影响,导致检测能力受限。针对该问题,本文提出了一种基于对抗注意力扩散模型的高效FDIA攻击样本生成方法。该方案由一个扩散模型和一个带注意力机制的GAN模型(ATTGAN)组成。首先利用扩散模型的前向扩散,在注入攻击向量的同时对真实数据添加噪声;然后训练ATTGAN模型,使其有效聚焦电网量测信息和拓扑节点信息,同时弱化无关信息;在反向扩散过程中,结合训练好的ATTGAN模型对噪声进行预测,并在此过程中逐步向前迭代并进行去噪;最后生成大量高效的FDIA攻击样本。在IEEE 14、IEEE 39和IEEE 118总线系统上进行了大量的实验,实验结果表明,生成的攻击样本在逃避检测能力、鲁棒性和攻击强度方面均优于现有的最优方案。
Citation: Kunzhan Li, Fengyong Li, Baonan Wang, Meijing Shan. False data injection attack sample generation using an adversarial attention-diffusion model in smart grids[J]. AIMS Energy, 2024, 12(6): 1271-1293. doi: 10.3934/energy.2024058
引用:李昆战,李凤勇,王宝南,单梅静。智能电网中基于对抗性注意力扩散模型的虚假数据注入攻击样本生成[J]。AIMS Energy,2024, 12(6): 1271-1293。doi: 10.3934/energy.2024058
[1] |
Stefania Conti, Santi A. Rizzo, Nunzio Salerno, Giuseppe M. Tina .
Distribution network topology identification based on synchrophasor. AIMS Energy, 2018, 6(2): 245-260.
doi: 10.3934/energy.2018.2.245
斯特凡尼亚·康蒂、桑蒂·A·里佐、农齐奥·萨勒诺、朱塞佩·M·蒂娜。基于同步相量的配电网拓扑识别。 AIMS能源,2018,6(2):245-260。 doi:10.3934/energy.2018.2.245 |
[2] |
Kangli Xiang, Keren Chen, Simin Chen, Wanqing Chen, Jinyu Chen .
Model for sustainable carbon emission reduction energy development and smart grid technology strategy. AIMS Energy, 2024, 12(6): 1206-1224.
doi: 10.3934/energy.2024055
项康丽, 陈可仁, 陈思敏, 陈万清, 陈金宇 . 可持续碳减排能源发展模型与智能电网技术战略. AIMS Energy, 2024, 12(6): 1206-1224. doi: 10.3934/energy.2024055 |
[3] |
Hassan Shirzeh, Fazel Naghdy, Philip Ciufo, Montserrat Ros .
Stochastic energy balancing in substation energy management. AIMS Energy, 2015, 3(4): 810-837.
doi: 10.3934/energy.2015.4.810
Hassan Shirzeh、Fazel Naghdy、Philip Ciufo、Montserrat Ros。变电站能源管理中的随机能源平衡。AIMS Energy,2015 年,3(4): 810-837。doi: 10.3934/energy.2015.4.810 |
[4] |
HVV Priyadarshana, MA Kalhan Sandaru, KTMU Hemapala, WDAS Wijayapala .
A review on Multi-Agent system based energy management systems for micro grids. AIMS Energy, 2019, 7(6): 924-943.
doi: 10.3934/energy.2019.6.924
HVV Priyadarshana、MA Kalhan Sandaru、KTMU Hemapala、WDAS Wijayapala。基于多代理系统的微电网能源管理系统的回顾。 AIMS能源,2019,7(6):924-943。 doi: 10.3934/energy.2019.6.924 |
[5] |
Syed Sabir Hussain Rizvi, Krishna Teerth Chaturvedi, Mohan Lal Kolhe .
A review on peak shaving techniques for smart grids. AIMS Energy, 2023, 11(4): 723-752.
doi: 10.3934/energy.2023036
Syed Sabir Hussain Rizvi、Krishna Teerth Chaturvedi、Mohan Lal Kolhe。智能电网调峰技术综述。 AIMS能源,2023,11(4):723-752。 doi:10.3934/energy.2023036 |
[6] |
Wesly Jean, Antonio C. P. Brasil Junior, Eugênia Cornils Monteiro da Silva .
Smart grid systems infrastructures and distributed solar power generation in urban slums–A case study and energy policy in Rio de Janeiro. AIMS Energy, 2023, 11(3): 486-502.
doi: 10.3934/energy.2023025
Wesly Jean、Antonio CP Brasil Junior、Eugênia Cornils Monteiro da Silva。城市贫民窟的智能电网系统基础设施和分布式太阳能发电——里约热内卢的案例研究和能源政策。AIMS Energy,2023 年,11(3):486-502。doi:10.3934/energy.2023025 |
[7] |
Ricardo Echeverri-Martínez, Wilfredo Alfonso-Morales, Eduardo F. Caicedo-Bravo .
A methodological Decision-Making support for the planning, design and operation of smart grid projects. AIMS Energy, 2020, 8(4): 627-651.
doi: 10.3934/energy.2020.4.627
Ricardo Echeverri-Martínez, Wilfredo Alfonso-Morales, Eduardo F. Caicedo-Bravo . 为智能电网项目的规划、设计和运营提供决策支持的方法。AIMS Energy,2020 年,8(4): 627-651。doi: 10.3934/energy.2020.4.627 |
[8] |
Kushan Tharuka Lulbadda, K. T. M. U. Hemapala .
The additional functions of smart inverters. AIMS Energy, 2019, 7(6): 971-988.
doi: 10.3934/energy.2019.6.971
Kushan Tharuka Lulbadda、KTMU Hemapala。智能逆变器的附加功能。AIMS Energy,2019 年,7(6): 971-988。doi: 10.3934/energy.2019.6.971 |
[9] |
Baseem Khan, Hassan Haes Alhelou, Fsaha Mebrahtu .
A holistic analysis of distribution system reliability assessment methods with conventional and renewable energy sources. AIMS Energy, 2019, 7(4): 413-429.
doi: 10.3934/energy.2019.4.413
Baseem Khan、Hassan Haes Alhelou、Fsaha Mebrahtu。传统和可再生能源配电系统可靠性评估方法的整体分析。AIMS Energy,2019 年,7(4): 413-429。doi: 10.3934/energy.2019.4.413 |
[10] |
M. Ebrahim Adabi, Juan A. Martinez-Velasco .
Solid state transformer technologies and applications: A bibliographical survey. AIMS Energy, 2018, 6(2): 291-338.
doi: 10.3934/energy.2018.2.291
M. Ebrahim Adabi, Juan A. Martinez-Velasco . 固态变压器技术与应用:文献综述。AIMS Energy,2018,6(2): 291-338。doi: 10.3934/energy.2018.2.291 |
A false data injection attack (FDIA) indicates that attackers mislead system decisions by inputting false or tampered data into the system, which seriously threatens the security of power cyber-physical systems. Considering the scarcity of FDIA attack samples, the traditional FDIA detection models based on neural networks are always limited in their detection capabilities due to imbalanced training samples. To address this problem, this paper proposes an efficient FDIA attack sample generation method by an adversarial attention-diffusion model. The proposed scheme consists of a diffusion model and a GAN model with an attention mechanism (ATTGAN). First, the forward diffusion of the diffusion model was used to add noise to the real data while injecting the attack vector. Then, the ATTGAN model was trained to effectively focus on the information of power grid measurements and topological nodes, while weakening irrelevant information. In the reverse diffusion process, the trained ATTGAN model was combined to predict the noise, and it was further iterated forward step by step and denoised in this process. Finally, a large number of efficient FDIA attack samples can were generated. Extensive experiments have been carried out on IEEE 14, IEEE 39, and IEEE 118 bus systems. The experimental results indicate that the generated attack samples outperform existing state-of-the-art schemes in terms of evasion detection capability, robustness, and attack strength.
虚假数据注入攻击(FDIA)是指攻击者通过向系统输入虚假或篡改的数据,误导系统决策,严重威胁电力信息物理系统的安全。考虑到FDIA攻击样本的稀缺性,传统的基于神经网络的FDIA检测模型总是受到训练样本不平衡的影响,导致检测能力受限。针对该问题,本文提出了一种基于对抗注意力扩散模型的高效FDIA攻击样本生成方法。该方案由一个扩散模型和一个带注意力机制的GAN模型(ATTGAN)组成。首先利用扩散模型的前向扩散,在注入攻击向量的同时对真实数据添加噪声;然后训练ATTGAN模型,使其有效聚焦电网量测信息和拓扑节点信息,同时弱化无关信息;在反向扩散过程中,结合训练好的ATTGAN模型对噪声进行预测,并在此过程中逐步向前迭代并进行去噪;最后生成大量高效的FDIA攻击样本。在IEEE 14、IEEE 39和IEEE 118总线系统上进行了大量的实验,实验结果表明,生成的攻击样本在逃避检测能力、鲁棒性和攻击强度方面均优于现有的最优方案。
As a key component of modern power systems, smart grids are designed to achieve efficient, flexible, and safe energy distribution. By integrating advanced sensor technology, communication networks, and intelligent control strategies, smart grids have gradually evolved into a highly integrated cyber-physical system (CPS) [1], and can respond to various operating conditions and external events in real time, optimize resource allocation, and improve the system's adaptability and anti-interference capabilities. However, this progress has also brought new security challenges, especially in the face of increasingly complex cyber attacks. A false data injection attack (FDIA) is one of the main security threats facing smart grids. By manipulating the measurement data of the power grid, attackers can mislead the system state estimation and decision-making process, thereby posing a serious threat to the stability and security of the power grid [2]. The stealth and complexity of such attacks make it difficult for traditional security measures to effectively deal with it. It is urgent to develop new defense mechanisms to ensure the data integrity and system security of smart grids.
智能电网是现代电力系统的重要组成部分,旨在实现高效、灵活、安全的能源分配。智能电网通过集成先进的传感器技术、通信网络和智能控制策略,逐渐发展成为高度集成的信息物理系统 (CPS) [1] 并能实时响应各种运行状况和外部事件,优化资源配置,提高系统的适应性和抗干扰能力。然而,这种进步也带来了新的安全挑战,特别是面对日益复杂的网络攻击。虚假数据注入攻击(FDIA)是智能电网面临的主要安全威胁之一。攻击者通过操纵电网的测量数据,可以误导系统状态估计和决策过程,从而对电网的稳定性和安全性构成严重威胁 [2] 此类攻击的隐蔽性、复杂性使得传统安全手段难以有效应对,亟待开发新的防御机制,保障智能电网的数据完整性和系统安全。
In recent years, large-scale grid security incidents in which criminals use data tampering mechanisms to attack occur frequently. Take the attack on Ukrenergo as an example. From 2015 to 2016, Ukrenergo suffered two cyber attacks. The attackers injected false data into the monitoring data acquisition system and deleted the original data, causing huge economic losses and social unrest [3]. This has also attracted the attention of many researchers to FDIA attacks. Since false data can be cleverly designed by attackers to evade detection by detection mechanisms, the purpose of intrusion can be achieved, causing irreversible damage to the power system. Therefore, society has carried out a lot of research work to identify and defend against an FDIA. For example, Kumar et al. [4] explored the effectiveness of machine learning algorithms in detecting false data injection attacks (FDIA) in critical infrastructure. Cheng et al. [5] introduced a highly discriminative FDIA detector called k-minimum residual similarity (kSRS) detection. This method achieves a high detection rate and low false alarm rate for an FDIA by characterizing the statistical consistency of measurement residuals in AC state estimation. Qu et al. [6] proposed a novel approach to improve the accuracy of DDIA detection and localization by synthesizing topological correlations in non-Euclidean spatial attributes of grid data. However, the above research is based on traditional machine learning to design FDIA detection solutions, which are limited by the complexity of manual feature extraction, dimensionality disaster, and insufficient generalization ability when detecting FDIA attacks. Therefore, traditional FDIA detection methods have gradually lost their effectiveness in defending against these network attacks.
近年来,犯罪分子利用数据篡改手段进行攻击的大规模电网安全事件频频发生。以乌克兰国家电网公司遭受攻击为例,2015年至2016年,乌克兰国家电网公司遭受了两次网络攻击,攻击者向监控数据采集系统注入虚假数据,并删除原始数据,造成巨大的经济损失和社会动荡 [3] 这也引起了众多研究者对FDIA攻击的关注,由于攻击者可以巧妙设计虚假数据以逃避检测机制的检测,从而达到入侵的目的,对电力系统造成不可逆的破坏,因此社会上开展了大量对FDIA的识别和防御研究工作,例如Kumar等[1]对FDIA进行了研究。 [4] 探索了机器学习算法在检测关键基础设施中的虚假数据注入攻击 (FDIA) 方面的有效性。Cheng 等人 [5] 介绍了一种高辨别性的 FDIA 检测器,称为 k 最小残差相似度 (kSRS) 检测。该方法通过表征 AC 状态估计中测量残差的统计一致性,实现了 FDIA 的高检测率和低误报率。Qu 等人 [6] 提出了一种通过综合栅格数据非欧几里德空间属性中的拓扑相关性来提高DDIA检测与定位准确率的新方法。但上述研究都是基于传统机器学习来设计FDIA检测方案,在检测FDIA攻击时受到人工特征提取的复杂性、维数灾难、泛化能力不足等限制。 因此,传统的FDIA检测方法在防御这些网络攻击方面已逐渐失去效力。
With the update and iteration of technology, some FDIA detection methods based on machine learning and deep learning have also been developed. In order to correctly detect false data injection attacks in power grids, Fand et al. [7] proposed a one-dimensional convolutional neural network (1DCNN) and long short-term memory (LSTM) network multi-channel fusion network model based on the residual neural network (ResNet) structure, referred to as the channel-fused Res-CNN-LSTM network model. In order to make the detection results more credible, Su et al. [8] proposed an interpretable deep learning FDIA detection method, namely the dual attention multi-head graph attention network (DAMGAT). Takiddin et al. [9] proposed an anomaly detector based on generalized graph neural networks (GNN), which is robust to an FDIA and data poisoning. Li et al. [10] proposed a false data detection method based on graph neural networks, extracting the spatial features of power grid topology information and operation data through gated graph neural networks (GGNN). Qu et al. [11] proposed an improved adaptive Kalman filter (AKF) and convolutional neural network (CNN) hybrid detection method for power information physical system (CPS) FDIA. Wang et al. [12] proposed a deep learning-based location detection architecture (DLLD) to detect the exact location of an FDIA in real time. Xie et al. [13] proposed a Bayesian deep learning-based method to detect network attacks and maintain the security of smart grids. Although these methods show great advantages in FDIA attack detection, they also have great limitations. Most of the methods rely on large-scale training attack samples, but in the actual scenario, FDIA attack samples are difficult to obtain, and samples are scarce, resulting in extremely unbalanced sample data sets. Although they have strong detection performance, if there are not enough attack samples for model training, the efficiency of the FDIA attack detection model in smart grids will also be reduced. Therefore, the construction of FDIA attack samples and the acquisition of balanced data sets become the first problems to be solved.
随着技术的更新迭代,一些基于机器学习、深度学习的FDIA检测方法也得到了发展。为了正确检测电网中的虚假数据注入攻击,Fand等[9]提出了一种基于FDIA的检测方法,用于检测电网中的虚假数据注入攻击。 [7] 提出了一种基于残差神经网络(ResNet)结构的一维卷积神经网络(1DCNN)与长短期记忆网络(LSTM)多通道融合网络模型,简称通道融合的Res-CNN-LSTM网络模型。为了使检测结果更加可信,苏等人。 [8] 提出了一种可解释的深度学习FDIA检测方法,即双重注意多头图注意网络(DAMGAT)。Takiddin等人 [9] 提出了一种基于广义图神经网络(GNN)的异常检测器,该检测器对 FDIA 和数据中毒具有很强的鲁棒性。Li 等人 [10] 提出了一种基于图神经网络的虚假数据检测方法,通过门控图神经网络(GGNN)提取电网拓扑信息和运行数据的空间特征。 [11] 提出了一种针对电力信息物理系统(CPS)FDIA的改进自适应卡尔曼滤波(AKF)和卷积神经网络(CNN)混合检测方法。 [12] 提出了一种基于深度学习的位置检测架构 (DLLD),用于实时检测 FDIA 的精确位置。Xie 等人 [13] 提出了基于贝叶斯深度学习的方法来检测网络攻击,维护智能电网的安全。虽然这些方法在FDIA攻击检测中表现出很大的优势,但也存在很大的局限性。 大部分方法都依赖于大规模训练攻击样本,但在实际场景中,FDIA攻击样本获取困难,样本稀缺,导致样本数据集极不平衡。虽然具有较强的检测性能,但如果没有足够的攻击样本进行模型训练,智能电网中FDIA攻击检测模型的效率也会降低。因此,FDIA攻击样本的构建和均衡数据集的获取成为首先要解决的问题。
Several researchers have conducted extensive research on the construction of FDIA attack vectors. Yan et al. [14] proposed the DoS-WGAN architecture, which uses a Wasserstein generative adversarial network (WGAN) with gradient penalty technology to evade network traffic classifiers. WGAN is used to automatically synthesize attack vectors that can be disguised as normal network traffic in the case of network attacks and bypass the detection of network intrusion detection systems. To address the problem of highly unbalanced data samples, Kumar et al. [15] proposed a Wasserstein conditional generative adversarial network (WCGAN) combined with an XGBoost classifier. The gradient penalty is used together with WCGAN for stable learning of the model. Tian et al. [16] introduced the joint adversarial examples and FDIA (AFDIA) to explore various attack scenarios on power system state estimation. Considering that disturbances directly added to the measurement are likely to be detected by the BDD, a method of adding disturbances to the state variables is proposed to ensure that the attack is invisible to the BDD. Bhattacharjee et al. [17] proposed a scheme for constructing invisible attack vectors based on independent component analysis to identify mixing matrices. Wu et al. [18] proposed a new FDIA sample generation method to construct large-scale attack samples by introducing a mixed Laplace model that can accurately fit the distribution of data changes. Li et al. [19] designed an efficient adversarial model for generating FDIA attack samples at the edge of public and private networks that can effectively bypass detection models and threaten grid systems. Through interactive adversarial learning, efficient FDIA attack samples can be continuously generated. Although the existing sample generation method can appropriately expand the FDIA attack sample library, its generation method is too simple, resulting in low concealment and weak attack capability of the generated samples, which is not conducive to the training of the detection model and the improvement of performance.
许多研究人员对 FDIA 攻击向量的构建进行了广泛的研究。Yan 等人。 [14] 提出了 DoS-WGAN 架构,利用 Wasserstein 生成对抗网络 (WGAN) 结合梯度惩罚技术来逃避网络流量分类器。WGAN 用于在网络攻击发生时自动合成可以伪装成正常网络流量的攻击向量,绕过网络入侵检测系统的检测。针对数据样本高度不平衡的问题,Kumar 等人提出了一种基于 WGAN 的 DoS-WGAN 架构。 [15] 提出了一种结合XGBoost分类器的Wasserstein条件生成对抗网络(WCGAN),并结合梯度惩罚和WCGAN实现模型的稳定学习。Tian等 [16] 引入联合对抗样本和 FDIA(AFDIA)来探索针对电力系统状态估计的各种攻击场景。考虑到直接添加到测量中的扰动很可能被 BDD 检测到,提出了一种向状态变量添加扰动的方法,以确保攻击对 BDD 不可见。Bhattacharjee 等人。 [17] 提出了一种基于独立成分分析识别混合矩阵的隐形攻击向量构建方案。吴等人。 [18] 通过引入能够准确拟合数据变化分布的混合拉普拉斯模型,提出了一种新的FDIA样本生成方法来构造大规模攻击样本。 [19] 设计了一种有效的对抗模型,用于在公共和私有网络边缘生成 FDIA 攻击样本,可以有效绕过检测模型并威胁电网系统。 通过交互式对抗学习,可以不断生成高效的FDIA攻击样本。现有的样本生成方法虽然可以适当扩充FDIA攻击样本库,但是其生成方法过于简单,导致生成的样本隐蔽性不高、攻击能力弱,不利于检测模型的训练和性能的提升。
With the rise of generative AI technology, a new perspective has been brought to the detection of various network attacks. Among them, the diffusion model has gradually become a new and most advanced model among the deep generation models in recent years, showing superior performance in the research of image generation and multi-modal generation. Compared with traditional deep learning methods, it can generate new attack samples with better concealment and attack capabilities by learning the distribution of data, which provides a new way to understand the strategies that attackers may adopt. Therefore, in response to the above problems, considering the diversity, concealment, and scalability of the data generated by the diffusion model [20] and its powerful generation ability, this paper presents a method to generate FDIA attack samples using an adversarial attention diffusion model. The proposed AttDiff model consists of a diffusion model and a GAN (ATTGAN) model with an attention mechanism. First, as the time step increases, in the forward diffusion process, the original input data is gradually denoised, the attack vector is injected, and the data state corresponding to each time step and the added noise are recorded. The recorded data is then used to train generative adversarial network (ATTGAN) models with attention mechanisms. The purpose is to allow the ATTGAN model to effectively focus on the information of power grid measurements and topological nodes through adversarial training, while weakening irrelevant information and fully learning the characteristics of real sample data. Afterward, in the reverse diffusion process, the noise-added data processed in the forward diffusion process should be denoised from back to front in combination with the size of the time step, and the trained ATTGAN model should be combined to predict the noise value to be removed. In this process, the purpose is to build the attack sample data closest to the real sample from the noise. The FDIA sample generated by the method in this paper is more diversified, more hidden, and can effectively simulate the behavior of the real attacker, and is less likely to be detected by the detector.
随着生成式人工智能技术的兴起,为各类网络攻击的检测带来了新的视角。其中,扩散模型近年来逐渐成为深度生成模型中较新的、最先进的模型,在图像生成、多模态生成的研究中表现出优越的性能。与传统的深度学习方法相比,它可以通过学习数据的分布来生成隐蔽性和攻击能力更强的新型攻击样本,为理解攻击者可能采取的策略提供了新的思路。因此,针对上述问题,考虑扩散模型生成数据的多样性、隐蔽性和可扩展性 [20] 及其强大的生成能力,本文提出一种利用对抗性注意扩散模型生成FDIA攻击样本的方法。提出的AttDiff模型由扩散模型和带有注意机制的GAN(ATTGAN)模型组成。首先,随着时间步长的增加,在前向扩散过程中,对原始输入数据逐渐去噪,注入攻击向量,并记录每个时间步长对应的数据状态和添加的噪声。然后利用记录的数据来训练带有注意机制的生成对抗网络(ATTGAN)模型。目的是让ATTGAN模型通过对抗性训练有效地聚焦电网测量值和拓扑节点信息,同时弱化无关信息,充分学习真实样本数据的特点。 随后在反向扩散过程中,需要结合时间步长的大小,从后向前对正向扩散过程中处理好的加噪数据进行去噪,并结合训练好的ATTGAN模型预测需要去除的噪声值。此过程的目的是从噪声中构建出最接近真实样本的攻击样本数据。本文方法生成的FDIA样本更加多样化,隐蔽性更强,能够有效模拟真实攻击者的行为,且被检测器检测到的概率更小。
Comparing with the existing schemes, we make the following novel contributions in FDIA attack sample generation:
与现有方案相比,我们在FDIA攻击样本生成方面做出了以下新颖的贡献:
● We propose a novel and efficient sample generation method. Our proposed scheme introduces an adversarial attention-diffusion model to generate a large number of attack samples, which can solve the problem of scarce attack samples in FDIA detection. According to the investigation, this is the first time that the diffusion model has been applied to the study of FDIA attack sample generation.
● 提出了一种新颖、高效的样本生成方法。该方案引入了对抗性注意力扩散模型来生成大量攻击样本,可以解决FDIA检测中攻击样本稀缺的问题。经调查,这是首次将扩散模型应用于FDIA攻击样本生成的研究中。
● We use the forward diffusion of the diffusion model to add noise to the real data while injecting the attack vector. Subsequently, the ATTGAN model is trained to effectively focus on the information of power grid measurements and topological nodes, while weakening irrelevant information. In the reverse diffusion process, we combine the trained ATTGAN model to predict the noise, and gradually iterate forward step by step and denoise in this process. A large number of efficient FDIA attack samples can be generated.
● 利用扩散模型的前向扩散,在注入攻击向量的同时,对真实数据添加噪声,随后训练ATTGAN模型,有效聚焦电网量测信息和拓扑节点信息,同时弱化无关信息;在反向扩散过程中,结合训练好的ATTGAN模型对噪声进行预测,并在此过程中逐步向前迭代,不断去噪,可以生成大量高效的FDIA攻击样本。
● Comprehensive experiments are performed with classical data sets. The experimental results demonstrate that the proposed method can significantly improve the overall performance against FDIA detection, and outperform the existing FDIA attack sample construction methods in terms of attack strength, concealment, and decline of FDIA detection accuracy.
● 使用经典数据集进行了全面的实验,实验结果表明,所提方法能够显著提高针对FDIA检测的整体性能,并且在攻击强度、隐蔽性、FDIA检测准确率下降等方面优于现有的FDIA攻击样本构造方法。
The rest of this paper is organized as follows. In Section 2, related works on FDIAs and diffusion models are introduced. In Section 3, the framework of the proposed attack sample generation method based on the adversarial attention diffusion model and the specific structure of the AttDiff model are introduced in detail. In Section 4, a large number of experiments and corresponding comparative experiments are carried out, and the experimental results are presented. Finally, in Section 5, the work of this paper is summarized and prospected.
本文其余部分安排如下。第2节介绍了FDIA和扩散模型的相关工作。第3节详细介绍了本文提出的基于对抗注意力扩散模型的攻击样本生成方法的框架和AttDiff模型的具体结构。第4节进行了大量的实验和相应的对比实验,并给出了实验结果。最后,第5节对本文的工作进行了总结和展望。
A fake data injection attack is a kind of network attack against CPS. Its core is to construct and inject fake data to bypass system detection, so as to achieve the purpose of attack. This type of attack is particularly common in critical infrastructure such as smart grids and can have a serious impact on the operational stability, supply reliability, and data accuracy of the power system.
虚假数据注入攻击是针对CPS的一种网络攻击,其核心是通过构造和注入虚假数据来绕过系统检测,从而达到攻击目的。此类攻击在智能电网等关键基础设施中尤为常见,会对电力系统的运行稳定性、供电可靠性、数据准确性造成严重影响。
The core principle of constructing an FDIA is to manipulate the key measurement data in the AC power system in order to change the state estimation results of the system. By tampering with the active power on any bus node, an attacker generates a set of attack vectors designed to interfere with the normal operation of the system. These attack vectors are injected into the measured data of the system, which causes the calculated state estimation to deviate from the real operating state of the power grid. In this way, attackers are able to mislead the system's decision-making process for their potential sabotage or manipulation purposes. In DC power flow, the relationship between system state and measurement data can be expressed as:
构建FDIA的核心原理是操纵交流电力系统中的关键测量数据,以改变系统的状态估计结果。通过篡改任意总线节点的有功功率,攻击者可以生成一组旨在干扰系统正常运行的攻击向量。这些攻击向量被注入到系统的测量数据中,导致计算出的状态估计偏离电网的实际运行状态。通过这种方式,攻击者能够误导系统的决策过程,以实现潜在的破坏或操纵目的。在直流潮流中,系统状态与系统状态之间的关系 和测量数据 可以表示为:
(1) |
where represents the measurement data, represents the state vector, represents the measurement error vector, and represent the number of measurements and the number of state data, respectively, and represents the measurement Jacobian matrix, which is a matrix representing the grid topology information. The measurement data includes the flow and injection power of different buses.
在哪里 表示测量数据, 表示状态向量, 表示测量误差向量, 和 分别表示测量次数和状态数据次数, 表示测量雅可比矩阵,是表示电网拓扑信息的矩阵,测量数据包括不同母线的潮流和注入功率。
For DC state estimation, assuming that the per unit voltage of each node in the system is 1, ignoring the influence of line resistance and ground branch, the power between bus and bus is:
对于直流状态估计,假设系统各节点标幺电压为1,忽略线路电阻和接地支路的影响,母线间功率 和公交车 是:
(2) |
where represents the reactance of the transmission line between bus and bus . and are the voltage phase angles of bus and bus . The minimized objective function is estimated by linear weighted least squares:
在哪里 表示总线之间的传输线的电抗 和公交车 。 和 是母线电压相位角 和公交车 最小化目标函数通过线性加权最小二乘法估计:
(3) |
where is the error covariance matrix of the measurement, and of the minimized objective function is:
在哪里 是测量的误差协方差矩阵,并且 最小化目标函数为:
(4) |
The principle of residual detection has been widely used in the traditional bad data detection mechanism (BDD). Residual is the difference between the measurement value and the measurement estimate , i.e.:
残差检测的原理在传统的坏数据检测机制(BDD)中得到了广泛的应用。残差 是测量值之间的差异 和测量估计 , IE:
(5) |
BDD can then detect bad data by comparing the Euclidean norm of the measured residuals to a predefined threshold , as follows:
然后,BDD 可以通过将测量残差的欧几里德范数与预定义阈值进行比较来检测坏数据 ,如下所示:
(6) |
In order to make the injected attack vector invisible, we assume that the measurement error follows an ideal normal distribution, and use to represent the FDIA vector injected by the attacker into the measured value. Then the actual measurement data is , and the error vector of the state variable caused by the FDIA is . At this time, the estimated state variable , and the residual error after attack can be expressed as:
为了使注入的攻击向量不可见,我们假设测量误差 遵循理想的正态分布,并使用 表示攻击者注入到测量值的FDIA向量。则实际测量数据为 ,FDIA引起的状态变量的误差向量为 此时估计的状态变量 ,攻击后的残差误差可表示为:
(7) |
When , the following formula holds:
什么时候 ,以下公式成立:
(8) |
It can be seen that when the above conditions are met, an FDIA can successfully bypass BDD, causing changes and losses to the state estimation of the power system. However, it is worth noting that the basic assumption of an FDIA is that both the attacker and the defender (that is, the power system operator) have complete information about the parameters and topology of the power system, that is, in Eq (1), and it is also necessary to find highly sparse attack vectors that meet certain conditions to launch attacks. For this, the cost is high.
可以看出,当满足上述条件时,FDIA 可以成功绕过 BDD,给电力系统的状态估计带来变化和损失。但值得注意的是,FDIA 的基本假设是攻击者和防御者(即电力系统运营商)都拥有关于电力系统参数和拓扑的完整信息,即 在式(1)中,还需要找到满足一定条件的高度稀疏的攻击向量才能发动攻击,这样做的成本较高。
Diffusion models have surpassed the previous leading generative adversarial network (GAN) technology in the field of image creation and have shown great application potential in multiple fields such as computer vision, natural language processing, signal processing, multimodal learning, molecular structure modeling, time series analysis, and adversarial sample purification. In the field of computer vision, diffusion models are used to perform various image restoration tasks, such as image resolution enhancement, image painting, and image translation [21–23]. In natural language processing, diffusion models can generate character level text through a technique called the discrete denoising diffusion probability model (D3PM) [24]. In addition, in terms of time series data generation, diffusion models are also used to fill in the blanks in time series data, as shown in the literature [25,26]. In the field of data generation, due to the similar structure of time series data and intrusion detection data, both of them are one-dimensional data composed of multiple features, which stimulates the idea of generating FDIA attack sample data in this experiment. According to the survey, there is no literature that uses diffusion models for FDIA attack sample generation to generate a balanced data set.
The existing sample generation methods are too simple, resulting in low concealment and weak attack ability of the generated samples, which is not conducive to the training and performance improvement of the detection model. The use of a diffusion model can construct more robust, more threatening, and more hidden attack samples, so that the training of the detection model can be further optimized and improved, and finally achieve the purpose of enhancing the defense capability of the power system, making its operation safer and more stable.
The proposed framework mainly consists of three parts: the data processing module, AttDiff model, and data generation module. In the data processing module, various interactive data from the virtual power grid and power plant are first collected and processed, and then the flow calculation is performed on these data. Then, the measurement data in the power system is further simulated through simulation. Then, the processed measurement data is input into the AttDiff model and the sample data is normalized to adapt to network training. After initializing the model parameters, the forward diffusion process of the diffusion model is entered. During this process, as the time step increases, noise and attack vectors are gradually added to the original data, and the data in the forward diffusion process is recorded. After this process is completed, the ATTGAN model is trained with the recorded data. In addition, in backpropagation, noise prediction is combined with a trained ATTGAN model, and noise is iteratively removed step by step with time steps. Finally, new attack samples are generated based on the features of the original data to obtain a balanced data set. The complete framework of the proposed attack sample generation method is shown in Figure 1.
In a cyber-physical system, data comes from a variety of sources and may include multiple sensors. These data have significant differences in structure, accuracy, transmission delay, and update frequency, so it is necessary to properly preprocess these diverse data. Each column of data in the data set represents a feature of the sensor, and different features correspond to different units of measurement and magnitude, which may lead to the uneven influence of certain attributes in data analysis and model training. In order to balance this dimensional difference and ensure that each attribute is given equal consideration during analysis and training, the minimum-maximum normalization technique is used to linearly transform the data into a specified interval while preserving the original relative order and distribution characteristics of the data. The specific normalization formula is as follows:
(9) |
where is the eigenvalue of the original data set, is the normalized data value, is the minimum eigenvalue, and is the maximum eigenvalue. In this way, each feature value is converted into a range of 0 to 1, which helps ensure that different features have the same importance in model training, and reduces the impact of dimension and magnitude.
In this section, the diffusion model is combined with the ATTGAN model to complete the forward diffusion and reverse diffusion process of the diffusion model, and finally generate more hidden FDIA attack samples. In the reverse diffusion process, the ATTGAN model is used for noise prediction. The specific structure of the AttDiff model is shown in Figure 2.
Diffusion models [20] usually contain two key stages: the forward diffusion process and reverse diffusion process. In this model, it is necessary to select a data point from the real data distribution as the starting point , and gradually add Gaussian noise and attack vectors to as the time step increases in the forward diffusion process, we finally turn into standard Gaussian noise . For each forward step , the noise disturbance is controlled by the following formula:
(10) |
where is a different variance scale. After the forward diffusion ends, the reverse diffusion process gradually reconstructs the original data by sampling and learning the diffusion model .
In addition, the parameter plays a crucial role in the diffusion model, and can directly affect its performance. Therefore, choosing the right value in our study is crucial to balance the concealment and quality of the generated attack samples. If is too large, the model may overfit the noise rather than the true distribution of the data, causing the generated attack sample to lose its correlation to the real data. Conversely, if is too small, the model may not be trained enough to efficiently generate high-quality attack samples. In the experimental part of this study, we find the optimal value by adjusting the value of and observing its effect on the quality of the generated sample to ensure that the generated attack sample is both highly concealed and able to effectively challenge the detection model.
For a specific data point extracted from the actual data distribution , this experiment constructs a forward diffusion process. The process involves gradually adding a small amount of Gaussian noise to the sample in steps along with varying degrees of attack vectors to generate a series of noisy data points . The noise variance at each step is controlled by . As the step size increases, the original data will have fewer and fewer recognizable features. When , the final data point will tend to be an isotropic Gaussian distribution.
In addition, in the forward diffusion process, this experiment extracted data points from normal sample data, and recorded each time step corresponding to the added random noise and the data value before the current state was noised. The purpose is to train the ATTGAN model with the recorded data after the forward diffusion is over. In the process of inverse diffusion, the added noise corresponding to each time step can be predicted and denoised more accurately, so that the attack sample is closer to the real original normal data, and the invisibility of the attack sample is increased.
One benefit of the above process is that we can use the re-parameterization technique. We can sample in closed form at any time point . Here we define , and , which means can be expressed as . In this way, we can directly use the initial data point to represent .
(11) |
By reversing the above forward diffusion process and sampling at the same time, the real sample data can be recovered from the Gaussian noise input . If the variance at each step is small enough, then will also take on the characteristics of a Gaussian distribution. However, it is important to note that directly estimating is challenging because it often relies on the entire data set. Therefore, the ATTGAN model trained after the forward diffusion process mentioned above is used in this experiment to predict a noise value by inputting the corresponding to the current time step to approximate these conditional probabilities, so as to perform the denoising process of reverse diffusion. According to the nature of the Markov chain, the reverse diffusion process at the current time step depends only on its previous time step , which means that we have the following formula:
(12) |
From this, we can know the overall process of generating samples by the diffusion model. The sample generation process of the diffusion model involves gradually adding noise to the data from front to back until the data is completely transformed into standard Gaussian noise. This process is called forward diffusion. Subsequently, in the reverse diffusion process, by learning how to reverse the operation of adding noise, the noise is gradually removed from the back to the front to restore the original sample data. In this way, new data points can be generated from a limited sample data set, and these newly generated sample data are exactly the data needed at the moment.
The ATTGAN model is mainly composed of generators and discriminators. In this experiment, in order to learn different features of the input data more effectively and improve the sensitivity of the model to specific parts of the input data, an attention mechanism layer is added to the generator, thereby improving the performance of the model.
The model's generator consists of six fully connected layers and is equipped with an appropriate activation function at each layer, such as LeakyReLU, to introduce nonlinear properties. In addition, an attention layer is introduced into the structure of the generator, which consists of three fully connected layers and focuses on the salient parts of the input features through a learnable weight vector . The output of the attention mechanism is combined with the raw output of the generator to enhance the detail and quality of the data. Note that the design of the attention layer is based on the following formula:
(13) |
where is the output of the previous layer, and are the learnable weights and biases, and softmax functions are used to generate a normalized weight vector .
The discriminator corresponds to the generator, again consisting of six fully connected layers, each of which is followed by an activation function. The goal of a discriminator is to distinguish between real data and data generated by a generator.
The training of the model follows the standard adversarial training process. In the training process, this experiment adopts binary cross entropy loss (BCELoss) to optimize the model. Generators and discriminators are optimized with the following loss functions:
(14) |
where and are the loss functions of the generator and discriminator, respectively, and represent the generator and discriminator, respectively, is the noise vector sampled from the prior distribution , and is the data point from the real data distribution . In this experiment, an Adam optimizer was used to train the model, the learning rate was 0.0001, and the batch size was 64. In the training process, the quality of the generated data and the performance of the discriminator are monitored in real time, and the hyperparameters are carefully adjusted to ensure the effectiveness and stability of the model.
After the forward diffusion of the diffusion model ends, the reverse diffusion process must be followed. Before the reverse diffusion, the ATTGAN model needs to be trained with data recorded during the forward diffusion process described above, which is used for noise prediction in the reverse diffusion. After the model is trained, it officially enters the process of reverse diffusion. Combined with the trained ATTGAN model, it iteratively denoises step by step from the back, and the noise value removed is predicted by the ATTGAN model. In the end, large-scale samples close to the real data will be generated, and these samples are the required attack samples, so that the rare sample library can be expanded to form a balanced data set. Finally, the purpose of improving the detection performance of the detector can be achieved, and then the defense capability of the power system can be enhanced. The overall process of FDIA attack sample generation based on the AttDiff model is shown in Algorithm 1.
Algorithm 1: FDIA Sample Generation Process based on the AttDiff Model | |
Input: | Original measurement data , number of time steps |
Output: | Generated FDIA attack samples |
1 | Initialization the number of time steps , batch size, and other related parameters |
2 | Forward Diffusion Process |
3 | for each time step = 1 to do |
4 | Generate noise and attack vectors of different intensities |
5 | Perform iterative calculation of forward diffusion according to Eq 11; |
6 | Record noisy data and current time step |
7 | Store the current and corresponding to for subsequent ATTGAN model training |
8 | end for |
9 | Train ATTGAN model using data recorded during forward diffusion process |
10 | Reverse Diffusion Process |
11 | for each time step = to 1 do |
12 | Predict noise for using the trained ATTGAN model |
13 | Denoise the data |
14 | Perform iterative calculation of reverse diffusion according to formula 12; |
15 | Update the data state for the next iteration |
16 | end for |
17 | Output the final denoised data after the last iteration of the reverse diffusion process. |
18 | Return the generated FDIA attack samples . |
Among them, steps 1–8 are the forward diffusion stage of the diffusion model. According to the size of the time step, noise and attack vectors are added to the original data by iterating from front to back, and the data state and added noise corresponding to each time step are recorded. Step 9 is the training stage of the ATTGAN model. The data recorded in the process of forward diffusion is used for training. The purpose is to enable the ATTGAN model to effectively focus on power grid measurement values and topological node information, while weakening irrelevant information and fully learning the characteristics of real sample data, so as to play a better role in reverse diffusion. Steps 10–18 are the reverse diffusion stage of the diffusion model. According to the size of the time step, the noisy data is denoised by iterating from back to front. In this process, the diffusion model is combined with the trained ATTGAN model to predict the noise value to be removed, so as to build the attack sample data that is closest to the real sample. Finally, a large number of FDIA attack samples with high quality and high concealment can be obtained.
In this study, three well-known power system test platforms, namely the IEEE 14 bus, IEEE 39 bus, and IEEE 118 bus systems [27,28], were used to evaluate and verify the performance of the proposed method. These bus systems are widely used benchmark models in power system analysis and research. They provide a simplified representation of the power system network, including bus configuration, electrical parameters, generator and load data, etc. Real load data from January 1, 2020, to May 1, 2022, were collected from the New York Independent System Operator (NYISO) as test data. These data cover a time range of more than two years, including seasonal changes throughout the year and load patterns under different weather conditions. By applying these actual load data to the above bus system, we can simulate the measurement data of the power system, and further obtain the load information of each bus state variable and each node, providing real data support for model evaluation.
In this experiment, the above test data are all the data under normal measurement conditions. In order to simulate the real attack behavior, the proposed method is used to attack the test data. A traditional FDIA is a classical method with a certain degree of invisibility and flexibility. The proposed method in this experiment combines the traditional FDIA method to construct an attack sample generation method based on adversarial attention diffusion. By combining the proposed adversarial generation network, the attack sample mixed with normal data features can be generated through reverse diffusion. In order to test the influence of different attack levels, the attack vector is divided into three different attack strengths according to the method in [29]: a) Weak attack: the ratio of the mean power injection deviation in to is less than 10; b) Strong attack: the ratio of the mean power injection deviation in to is greater than 30; c) Medium attack: FDIA samples that do not fall under either of the above attacks.
Since the three bus systems all contain 15,000 pieces of data, the data sets are divided into training sets, verification sets, and test sets according to the ratio of 5:3:2, and experimental comparison tests were carried out under different attack intensity scenarios.
In addition, the experiment uses four indicators, namely precision, recall, accuracy, and score, as the evaluation criteria for the output results, which are defined as follows:
(15) |
(16) |
(17) |
(18) |
where true positive (TP) indicates the amount of incorrect data correctly detected, true negative (TN) indicates the amount of normal data detected as normal, false positive (FP) indicates the amount of normal data incorrectly detected as incorrect, and false negative (FN) indicates the amount of incorrect data incorrectly detected as normal. The higher the accuracy and precision, the worse the concealment of the attack sample and the easier it is to be detected.
All experimental simulations in this study were performed on devices with an Intel Core i7-11390H CPU, NVIDIA GeForce MX450, and 16 GB RAM. MATPOWER is used to calculate the power flow and estimate the state of the data in MATLAB, and the proposed AttDiff model is used to generate FDIA attack samples in Python. The noise error of power flow calculation simulation measurement data is set to 0.25 and Gaussian noise with mean zero and standard deviation 1 is adopted. The time step of the diffusion model is set to 4.
In this study, large-scale experiments are carried out on IEEE 14, IEEE 39, and IEEE 118 bus systems to verify the performance of the attack samples generated by the proposed method. Two advanced deep learning detection models are used to detect the generated samples, namely the CNN-based detection model [12] and the LSTM-based detection model [30]. The three types of high, medium, and low attack intensity are subdivided into 9 attack intensities with low intensity is subdivided into 2, 5, and 10, medium intensity is subdivided into 15, 20, and 25, and high intensity is subdivided into 30, 40, and 50 for comparison. The corresponding experimental results are shown in Tables 1-6, in which Tables 1 and 2 are the test results of the IEEE 14 bus system, Tables 3 and 4 are the test results of the IEEE 39 bus system, and Tables 5 and 6 are the test results of the IEEE 118 bus system.
Attack Level | Attack Strength | Traditional FDIA [8] | LMM-FDIA [18] | AttDiff-FDIA | ||||||||
Precision | Recall | -Score | Precision | Recall | -Score | Precision | Recall | -Score | ||||
Weak | 2 | 0.6883 | 0.6992 | 0.6819 | 0.6740 | 0.6958 | 0.6730 | 0.6469 | 0.7548 | 0.6892 | ||
5 | 0.7289 | 0.7233 | 0.7130 | 0.6831 | 0.7482 | 0.7045 | 0.6664 | 0.7173 | 0.6755 | |||
10 | 0.7601 | 0.7829 | 0.7602 | 0.7079 | 0.6778 | 0.6768 | 0.6937 | 0.7017 | 0.6860 | |||
Medium | 15 | 0.8205 | 0.8101 | 0.8062 | 0.7351 | 0.6716 | 0.6867 | 0.7081 | 0.6757 | 0.6777 | ||
20 | 0.8469 | 0.8647 | 0.8483 | 0.7514 | 0.7095 | 0.7146 | 0.7129 | 0.6529 | 0.6661 | |||
25 | 0.8772 | 0.8817 | 0.8731 | 0.7656 | 0.6992 | 0.7159 | 0.7209 | 0.6941 | 0.6958 | |||
Strong | 30 | 0.9092 | 0.9111 | 0.9050 | 0.7857 | 0.7062 | 0.7314 | 0.7324 | 0.7014 | 0.7037 | ||
40 | 0.9223 | 0.9423 | 0.9289 | 0.7995 | 0.7286 | 0.7503 | 0.7561 | 0.7280 | 0.7297 | |||
50 | 0.9313 | 0.9490 | 0.9371 | 0.8117 | 0.7547 | 0.7702 | 0.7789 | 0.7156 | 0.7339 |
Attack Level | Attac Strength | Traditional FDIA [8] | LMM-FDIA [18] | AttDiff-FDIA | ||||||||
Precision | Recall | -Score | Precision | Recall | -Score | Precision | Recall | -Score | ||||
Weak | 2 | 0.7038 | 0.7208 | 0.7117 | 0.6859 | 0.7404 | 0.7119 | 0.6669 | 0.7540 | 0.7064 | ||
5 | 0.7167 | 0.7437 | 0.7298 | 0.6927 | 0.7707 | 0.7293 | 0.6828 | 0.7456 | 0.7127 | |||
10 | 0.7671 | 0.7915 | 0.7602 | 0.7235 | 0.7583 | 0.7404 | 0.6972 | 0.7551 | 0.7248 | |||
Medium | 15 | 0.7958 | 0.8235 | 0.8062 | 0.7431 | 0.7351 | 0.7383 | 0.7203 | 0.6961 | 0.7060 | ||
20 | 0.8277 | 0.8976 | 0.8610 | 0.7547 | 0.6959 | 0.7240 | 0.7220 | 0.6695 | 0.6935 | |||
25 | 0.8679 | 0.9059 | 0.8864 | 0.7565 | 0.7253 | 0.7402 | 0.7334 | 0.6325 | 0.6787 | |||
Strong | 30 | 0.9075 | 0.9317 | 0.9194 | 0.7715 | 0.7086 | 0.7379 | 0.743 | 0.6395 | 0.6862 | ||
40 | 0.9484 | 0.9361 | 0.9421 | 0.8039 | 0.7286 | 0.7641 | 0.7651 | 0.6792 | 0.7190 | |||
50 | 0.9601 | 0.8864 | 0.9213 | 0.8248 | 0.6981 | 0.7558 | 0.7957 | 0.7144 | 0.7527 |
Attack Level | Attack Strength | Traditional FDIA [8] | LMM-FDIA [18] | AttDiff-FDIA | ||||||||
Precision | Recall | -Score | Precision | Recall | -Score | Precision | Recall | -Score | ||||
Weak | 2 | 0.7187 | 0.7181 | 0.7078 | 0.7199 | 0.7223 | 0.7074 | 0.6934 | 0.6977 | 0.6815 | ||
5 | 0.7664 | 0.7799 | 0.7650 | 0.7200 | 0.7521 | 0.7242 | 0.7042 | 0.7200 | 0.6990 | |||
10 | 0.8594 | 0.8605 | 0.8549 | 0.7404 | 0.7263 | 0.7215 | 0.7208 | 0.7177 | 0.7074 | |||
Medium | 15 | 0.9094 | 0.9250 | 0.9141 | 0.7549 | 0.7109 | 0.7159 | 0.7119 | 0.7214 | 0.7034 | ||
20 | 0.9507 | 0.9437 | 0.9452 | 0.7672 | 0.7272 | 0.7342 | 0.7205 | 0.7132 | 0.7052 | |||
25 | 0.9737 | 0.9609 | 0.9660 | 0.7743 | 0.7122 | 0.7285 | 0.7309 | 0.7130 | 0.7082 | |||
Strong | 30 | 0.9801 | 0.9789 | 0.9787 | 0.7850 | 0.7346 | 0.7485 | 0.7439 | 0.7227 | 0.7211 | ||
40 | 0.9922 | 0.9817 | 0.9864 | 0.7836 | 0.7219 | 0.7385 | 0.7629 | 0.7115 | 0.7247 | |||
50 | 0.9919 | 0.9900 | 0.9906 | 0.8051 | 0.7451 | 0.7639 | 0.7943 | 0.7208 | 0.7456 |
Attack Level | Attack Strength | Traditional FDIA [8] | LMM-FDIA [18] | AttDiff-FDIA | ||||||||
Precision | Recall | -Score | Precision | Recall | -Score | Precision | Recall | -Score | ||||
Weak | 2 | 0.7414 | 0.7723 | 0.7555 | 0.7737 | 0.7234 | 0.7472 | 0.7180 | 0.7508 | 0.7328 | ||
5 | 0.8171 | 0.9069 | 0.8593 | 0.7976 | 0.8309 | 0.8138 | 0.7277 | 0.6972 | 0.7112 | |||
10 | 0.8814 | 0.9246 | 0.9011 | 0.8270 | 0.7857 | 0.8054 | 0.7461 | 0.7098 | 0.7273 | |||
Medium | 15 | 0.9326 | 0.9334 | 0.9325 | 0.8317 | 0.8007 | 0.8157 | 0.7526 | 0.7548 | 0.7533 | ||
20 | 0.9562 | 0.9651 | 0.9605 | 0.8549 | 0.7868 | 0.8194 | 0.7721 | 0.7269 | 0.7485 | |||
25 | 0.9835 | 0.9819 | 0.9826 | 0.8705 | 0.8009 | 0.8340 | 0.7866 | 0.7460 | 0.7656 | |||
Strong | 30 | 0.9867 | 0.9881 | 0.9874 | 0.8828 | 0.8098 | 0.8442 | 0.8135 | 0.7936 | 0.8024 | ||
40 | 0.9993 | 0.9954 | 0.9973 | 0.9039 | 0.7797 | 0.8371 | 0.8417 | 0.7673 | 0.8024 | |||
50 | 1.0000 | 0.9987 | 0.9993 | 0.9234 | 0.7419 | 0.8220 | 0.8860 | 0.7651 | 0.8195 |
Attack Level | Attack Strength | Traditional FDIA [8] | LMM-FDIA [18] | AttDiff-FDIA | ||||||||
Precision | Recall | -Score | Precision | Recall | -Score | Precision | Recall | -Score | ||||
Weak | 2 | 0.7134 | 0.7293 | 0.710 | 0.6996 | 0.7330 | 0.7039 | 0.6880 | 0.7415 | 0.7043 | ||
5 | 0.7462 | 0.7644 | 0.7455 | 0.7174 | 0.7304 | 0.7107 | 0.7035 | 0.7280 | 0.7039 | |||
10 | 0.8235 | 0.8068 | 0.8072 | 0.7407 | 0.7309 | 0.7208 | 0.7106 | 0.7240 | 0.7049 | |||
Medium | 15 | 0.8652 | 0.8707 | 0.8628 | 0.7644 | 0.7457 | 0.7439 | 0.7106 | 0.7357 | 0.7118 | ||
20 | 0.9282 | 0.9162 | 0.9190 | 0.7856 | 0.7318 | 0.7461 | 0.7329 | 0.7134 | 0.7118 | |||
25 | 0.9493 | 0.9322 | 0.9382 | 0.7990 | 0.7221 | 0.7467 | 0.7424 | 0.7187 | 0.7194 | |||
Strong | 30 | 0.9571 | 0.9603 | 0.9571 | 0.8110 | 0.7554 | 0.7733 | 0.7513 | 0.7469 | 0.7381 | ||
40 | 0.9746 | 0.9741 | 0.9734 | 0.8227 | 0.7357 | 0.7691 | 0.7991 | 0.7441 | 0.7601 | |||
50 | 0.9836 | 0.9773 | 0.9797 | 0.8422 | 0.7668 | 0.7925 | 0.8364 | 0.7577 | 0.7861 |
Attack Level | Attack Strength | Traditional FDIA [8] | LMM-FDIA [18] | AttDiff-FDIA | ||||||||
Precision | Recall | -Score | Precision | Recall | -Score | Precision | Recall | -Score | ||||
Weak | 2 | 0.7263 | 0.6764 | 0.6960 | 0.7159 | 0.8198 | 0.7640 | 0.6963 | 0.7457 | 0.7192 | ||
5 | 0.7541 | 0.7241 | 0.7382 | 0.7353 | 0.8149 | 0.7730 | 0.7179 | 0.6841 | 0.6994 | |||
10 | 0.8359 | 0.8269 | 0.8305 | 0.7637 | 0.8015 | 0.7819 | 0.7204 | 0.6691 | 0.6928 | |||
Medium | 15 | 0.8815 | 0.8458 | 0.8630 | 0.7829 | 0.7701 | 0.7761 | 0.7219 | 0.6527 | 0.6853 | ||
20 | 0.9153 | 0.9364 | 0.9255 | 0.7971 | 0.7372 | 0.7657 | 0.7323 | 0.6584 | 0.6920 | |||
25 | 0.9341 | 0.9515 | 0.9427 | 0.8090 | 0.7355 | 0.7704 | 0.7456 | 0.7242 | 0.7327 | |||
Strong | 30 | 0.9538 | 0.9624 | 0.9580 | 0.8270 | 0.7113 | 0.7648 | 0.7608 | 0.7020 | 0.7272 | ||
40 | 0.9776 | 0.9731 | 0.9753 | 0.8409 | 0.7082 | 0.7688 | 0.8002 | 0.7195 | 0.7574 | |||
50 | 0.9873 | 0.9737 | 0.9804 | 0.8630 | 0.7151 | 0.7812 | 0.8269 | 0.7501 | 0.7861 |
As can be seen from the table above, the attack sample generation method proposed in this experiment can obtain the lowest accuracy and lower score under different attack intensifications. When the CNN-based FDIA detection on the IEEE 14 bus system is used for low-intensity attacks of 5, the proposed method has a precision reduction of 6.256 and 1.676 compared with the traditional FDIA [8] method and the hybrid Laplace method [18], and a score reduction of 3.744 and 2.896 in , respectively. For medium-intensity attacks of 20, the proposed method has a precision reduction of 13.4 and 3.854 compared with the traditional FDIA [8] method and the mixed Laplacian method [18], and a score reduction of 18.22 and 4.852 in , respectively. For high-intensity attacks of 40, the proposed method has a precision reduction of 16.63 and 4.344 compared with the traditional FDIA [8] method and hybrid Laplacian method [18], and a score reduction of 19.91 and 2.06 in , respectively.
In addition, on the IEEE 39 and IEEE 118 bus systems, both the accuracy and score achieved the lowest values under the three attack intensities. This is enough to show that the samples generated by the method proposed in this experiment have a stronger ability to evade detection, and have better concealment and attack capabilities. In the comparison of the three methods, the reason why the method proposed in this experiment can achieve better performance results mainly depends on the following two reasons: First, this experiment introduces an attention mechanism in the application of the diffusion model, which makes the model itself pay more attention to the node features that have a greater impact on the results, thereby promoting the model's efficient learning of node data features; and second, by combining adversarial training in the entire diffusion process, it helps the model learn more robust feature representations, improves the generalization ability of the model, and then generates high-quality, indistinguishable attack sample data.
The main framework adopted in this experiment is the diffusion model. As we all know, the diffusion model is closely related to the time step, and the performance of the samples generated by the diffusion model is also closely related to the time step. In order to verify that the time step used is the most cost-effective value, a series of experiments were conducted to observe the effect of different time steps on the detection results of the final generated attack samples. Comparative experiments were carried out on IEEE 14, IEEE 39, and IEEE 118 bus systems using detection models based on CNN and LSTM. The result is shown in Figure 3.
This comparative experiment discusses the cases where the time step of the diffusion model is set to 2, 4, 6, 8, and 10, respectively. From the above experimental results, it can be seen that the detection accuracy on the three bus systems will decrease slightly with the increase of the time step. On the IEEE 14 bus test system, the detection accuracy of the CNN detector at different time steps is 0.6953, 0.6715, 0.6751, 0.673, and 0.6679, respectively. The detection accuracy of the LSTM detector at different time steps is 0.7051, 0.6788, 0.6744, 0.6727, and 0.6681, respectively. When the time step is 2, the detection accuracy based on the two detectors is the highest, and when the time step is 10, the detection accuracy is the lowest. However, when the time steps are 4, 6, 8, and 10, the accuracy only decreases in a small range, and there is not much difference.
In addition, for the comparison of the IEEE 39 and IEEE 118 bus systems, the detection accuracy based on the two detectors eventually showed a downward trend. For the diffusion model, the time step has a direct impact on the final detection result. Within a reasonable range, the larger the time step, the more detailed control the model will have, and the more opportunities to learn and approximate the true distribution of the data, and better capture and recover the complex patterns and structures in the data, so as to improve the diversity and authenticity of the generated samples. However, a larger the time step is not the better, because the increase of the time step will lead to a large increase in the amount of computation and training time, and too high of a time step may also cause the model to overfit the training data, and can not be well generalized to new data. In order to guarantee the quality of attack samples, the calculation cost is reduced as much as possible by analyzing the comparative experiments on three bus systems with different time steps. Therefore, the most cost-effective time step setting was chosen for this experiment, that is, the time step was set to 4. This setting can not only effectively control the time overhead, but also ensure the high quality of the generated samples.
In order to verify the reliability of the proposed sample generation method, a series of experiments were carried out to study the influence of different noise disturbances on the measured data of the power system. Because the actual power system measurement data is often disturbed by noise, it is crucial to understand the impact of these disturbances on the proposed approach. This experiment simulates this kind of interference by adding Gaussian noise with different variance to the data of each node in the power system. In addition, on the IEEE 14, IEEE 39, and IEEE 118 bus systems, the detection models based on CNN and LSTM were used to conduct comparative tests on the attack samples generated by the traditional FDIA method, the mixed Laplacian method, and the method proposed in this experiment. The test results are shown in the figure below. Figures 4, 5, and 6 are the tests based on the IEEE 14 bus system, the IEEE 39 bus system, and the IEEE 118 bus system, respectively.
In this comparative experiment, seven different noise variance levels were set for the measurement data: 0.25, 0.30, 0.35, 0.40, 0.45, 0.50, and 0.55. By observing the above experimental results, it can be found that the precision of all models shows a decreasing trend with the increase of the noise variance level. Therefore, the noise variance level in the simulated power grid environment in this study is set at 0.25. This decrease in detection accuracy is mainly due to the fact that the increase in noise variance makes it more difficult to distinguish between normal and attack samples. Noise interference makes it easier for pure data to be masked by it. In addition, the experimental data also show that under the two detection models, the attack sample generation method proposed in this experiment performs better than the traditional FDIA sample generation method and the mixed Laplacian sample generation method under various noise levels. This proves that the sample generation method proposed in this study has more obvious advantages over the existing technology in terms of robustness and adaptability. In general, the method proposed in this paper can generate more stable and anti-jamming FDIA attack samples, and has stronger ability to evade detection. This provides a large number of efficient attack sample resources for constructing and training FDIA detection models based on deep learning and AI.
The existing FDIA detection methods have been limited by the problem of sample scarcity and data set imbalance. This paper presents a method to generate FDIA attack samples using an adversarial attention diffusion model. The overall model of the method consists of two parts: the diffusion model and GAN model with an attention mechanism (ATTGAN). First, the original power flow data is noised by the forward diffusion of the diffusion model combined with the time step. In this process, the original measurement data and added noise corresponding to each time step are recorded for the subsequent model training. After the forward diffusion is completed, the data recorded during the forward diffusion process is used to train the proposed ATTGAN model, which is used for noise prediction during the reverse diffusion process. Finally, in the reverse diffusion process, the trained ATTGAN model is combined with the diffusion model, and according to the size of the time step, the iterative denoising is done step by step from back to front to generate FDIA attack samples with stronger concealment and attack capabilities. This experiment was conducted on a large scale on the IEEE 14, IEEE 39, and IEEE 118 bus systems. The experimental results show that the attack samples generated by the proposed sample generation method have better ability to evade detection after detection by two kinds of detectors, thus effectively reducing the detection precision and accuracy.
Although our proposed attention-diffusion method can generate more covert and disguised attack samples, thereby expanding the attack sample base, it also has some limitations. The main limitation is the relatively large time overhead of the model, which may affect its efficiency in real-time applications. In addition, model performance is sensitive to parameter selection and requires careful tuning to achieve optimal performance, potentially adding complexity to model deployment. To overcome these limitations, future work could explore algorithmic optimization and hardware acceleration to improve the computational efficiency of the model, as well as the application of hyperparameter optimization techniques to automate the parameter selection process to reduce the effort of manual adjustment and potentially discover a better combination of parameters. Future work could also further explore the explainability of the model, improve user trust in the model output, and extend the model's application to support broader smart grid security research.
The authors declare they have not used Artificial Intelligence (AI) tools in the creation of this article.
This research is supported by grants from the Engineering Research Center of Offshore Wind Technology Ministry of Education (Shanghai University of Electric Power).
The authors declare no conflicts of interest.
Conceptualization, Kunzhan Li; methodology, Kunzhan Li and Fengyong Li; validation, Kunzhan Li; formal analysis, Fengyong Li and Baonan Wang; writing—original draft preparation, Kunzhan Li; writing—review and editing, Fengyong Li and Baonan Wang; supervision, Meijing Shan. All authors have read and agreed to the published version of the manuscript.
[1] | Baheti R, Gill H (2011) Cyber-physical systems. The Tmpact of Control Technology 12: 161–166. Available from: www.ieeecss.org. |
[2] |
Aoufi S, Derhab A, Guerroumi M (2020) Survey of false data injection in smart power grid: Attacks, countermeasures and challenges. J Inf Secur Appl 54: 102518. https://doi.org/10.1016/j.jisa.2020.102518 doi: 10.1016/j.jisa.2020.102518
![]() |
[3] |
Li Z, Tong W, Jin X (2016) Construction of cyber security defense hierarchy and cyber security testing system of smart grid: Thinking and enlightenment for network attack events to national power grid of Ukraine and Israel. Autom Electr Power Syst 40: 147–151. https://doi.org/10.7500/AEPS20160313005 doi: 10.7500/AEPS20160313005
![]() |
[4] |
Kumar A, Saxena N, Jung S, et al. (2021) Improving detection of false data injection attacks using machine learning with feature selection and oversampling. Energies 15: 212. https://doi.org/10.3390/en15010212 doi: 10.3390/en15010212
![]() |
[5] |
Cheng G, Lin Y, Zhao J, et al. (2022) A highly discriminative detector against false data injection attacks in AC state estimation. IEEE Trans Smart Grid 13: 2318–2330. https://doi.org/10.1109/TSG.2022.3141803 doi: 10.1109/TSG.2022.3141803
![]() |
[6] |
Qu Z, Dong Y, Li Y, et al. (2024) Localization of dummy data injection attacks in power systems considering incomplete topological information: A spatio-temporal graph wavelet convolutional neural network approach. Appl Energy 360: 122736. https://doi.org/10.1016/j.apenergy.2024.122736 doi: 10.1016/j.apenergy.2024.122736
![]() |
[7] | Fang Z (2024) Detection of false data injection attacks in power grid based on Res-CNN-LSTM with channel fusion. Electr Eng 25: 11–17. Available from: www.cesmedia.cn. |
[8] |
Su X, Deng C, Yang J, et al. (2024) Damgat based interpretable detection of false data injection attacks in smart grids. IEEE Trans Smart Grid 15: 4182–4195. https://doi.org/10.1109/TSG.2024.3364665 doi: 10.1109/TSG.2024.3364665
![]() |
[9] |
Takiddin A, Ismail M, Atat R, et al. (2023) Robust graph autoencoder-based detection of false data injection attacks against data poisoning in smart grids. IEEE Trans Artif Intell 5: 1287–1301. https://doi.org/10.1109/TAI.2023.3286831 doi: 10.1109/TAI.2023.3286831
![]() |
[10] |
Li X, Wang Y, Lu Z (2023) Graph-based detection for false data injection attacks in power grid. Energy 263: 125865. https://doi.org/10.1016/j.energy.2022.125865 doi: 10.1016/j.energy.2022.125865
![]() |
[11] |
Qu Z, Bo X, Yu T, et al. (2022) Active and passive hybrid detection method for power CPS false data injection attacks with improved AKF and GRU‐CNN. IET Renewable Power Gener 16: 1490–1508. https://doi.org/10.1049/rpg2.12432 doi: 10.1049/rpg2.12432
![]() |
[12] |
Wang S, Bi S, Zhang YJA (2020) Locational detection of the false data injection attack in a smart grid: A multilabel classification approach. IEEE Int Things J 7: 8218–8227. https://doi.org/10.1109/JIOT.2020.2983911 doi: 10.1109/JIOT.2020.2983911
![]() |
[13] |
Xie J, Rahman A, Sun W (2024) Bayesian gan-based false data injection attack detection in active distribution grids with DERs. IEEE Trans Smart Grid 15: 3223–3234. https://doi.org/10.1109/TSG.2023.3337340 doi: 10.1109/TSG.2023.3337340
![]() |
[14] |
Yan Q, Wang M, Huang W, et al. (2019) Automatically synthesizing DoS attack traces using generative adversarial networks. Int J Mach Learn Cybern 10: 3387–3396. https://doi.org/10.1007/s13042-019-00925-6 doi: 10.1007/s13042-019-00925-6
![]() |
[15] |
Kumar V, Sinha D (2023) Synthetic attack data generation model applying generative adversarial network for intrusion detection. Comput Secur 125: 103054. https://doi.org/10.1016/j.cose.2022.103054 doi: 10.1016/j.cose.2022.103054
![]() |
[16] |
Tian J, Wang B, Wang Z, et al. (2021) Joint adversarial example and false data injection attacks for state estimation in power systems. IEEE Trans Cybern 52: 13699–13713. https://doi.org/10.1109/TCYB.2021.3125345 doi: 10.1109/TCYB.2021.3125345
![]() |
[17] |
Bhattacharjee A, Mondal AK, Verma A, et al. (2022) Deep latent space clustering for detection of stealthy false data injection attacks against AC state estimation in power systems. IEEE Trans Smart Grid 14: 2338–2351. https://doi.org/10.1109/TSG.2022.3216625 doi: 10.1109/TSG.2022.3216625
![]() |
[18] |
Wu Y, Zu T, Guo N, et al. (2023) Laplace-domain hybrid distribution model based FDIA attack sample generation in smart grids. Symmetry 15: 1669. https://doi.org/10.3390/sym15091669 doi: 10.3390/sym15091669
![]() |
[19] | Li F, Shen W, Bi Z, et al. (2024) Sparse adversarial learning for FDIA attack sample generation in distributed smart grids. CMES-Comput Model Eng Sci 139. https://doi.org/10.32604/cmes.2023.044431 |
[20] |
Tang B, Lu Y, Li Q, et al. (2023) A diffusion model based on network intrusion detection method for industrial cyber-physical systems. Sensors 23: 1141. https://doi.org/10.3390/s23031141 doi: 10.3390/s23031141
![]() |
[21] | Batzolis G, Stanczuk J, Schönlieb CB, et al. (2021) Conditional image generation with score-based diffusion models. arXiv preprint arXiv:2111, 13606. https://doi.org/10.48550/arXiv.2111.13606 |
[22] | Deng J, Dong W, Socher R, et al. (2009) Imagenet: A large-scale hierarchical image database. 2009 IEEE Conference on Computer Vision and Pattern Recognition, Miami, FL, USA, 248–255. https://doi.org/10.1109/CVPR.2009.5206848 |
[23] | Esser P, Rombach R, Ommer B (2021) Taming transformers for high-resolution image synthesis. Proceedings of the IEEE/CVF Conference on Computer Vision and Pattern Recognition, 12873–12883. https://doi.org/10.1109/CVPR46437.2021.01268 |
[24] | Austin J, Johnson DD, Ho J, et al. (2021) Structured denoising diffusion models in discrete state-spaces. Adv Neural Inf Process Syst 34: 17981–17993. Available from: www.proceedings.neurips.cc. |
[25] | Park SW, Lee K, Kwon J (2021) Neural markov controlled SDE: Stochastic optimization for continuous-time data. International Conference on Learning Representations. Available from: https://openreview.net/pdf?id = 7DI6op61AY. |
[26] | Tashiro Y, Song J, Song Y, et al. (2021) Csdi: Conditional score-based diffusion models for probabilistic time series imputation. Adv Neural Inf Process Syst 34: 24804–24816. Available from: www.proceedings.neurips.cc |
[27] |
Wu Y, Wang Q, Guo N, et al. (2023) Efficient multi-source self-attention data fusion for FDIA detection in smart grid. Symmetry 15: 1019. https://doi.org/10.3390/sym15051019 doi: 10.3390/sym15051019
![]() |
[28] | Wu Y, Sheng Y, Guo N, et al. (2022) Hybrid deep network based multi-source sensing data fusion for fdia detection in smart grid. 2022 Asia Power and Electrical Technology Conference (APET), 310–315. https://doi.org/10.1109/APET56294.2022.10072807 |
[29] |
Li Y, Wei X, Li Y, et al. (2022) Detection of false data injection attacks in smart grid: A secure federated deep learning approach. IEEE Trans Smart Grid 13: 4862–4872. https://doi.org/10.1109/TSG.2022.3204796 doi: 10.1109/TSG.2022.3204796
![]() |
[30] |
Musleh A, Chen G, Dong Z, et al. (2023) Attack detection in automatic generation control systems using LSTM-based stacked autoencoders. IEEE Trans Ind Inf 19: 153–165. https://doi.org/10.1109/TII.2022.3178418 doi: 10.1109/TII.2022.3178418
![]() |
Attack Level | Attack Strength | Traditional FDIA [8] | LMM-FDIA [18] | AttDiff-FDIA | ||||||||
Precision | Recall | -Score | Precision | Recall | -Score | Precision | Recall | -Score | ||||
Weak | 2 | 0.6883 | 0.6992 | 0.6819 | 0.6740 | 0.6958 | 0.6730 | 0.6469 | 0.7548 | 0.6892 | ||
5 | 0.7289 | 0.7233 | 0.7130 | 0.6831 | 0.7482 | 0.7045 | 0.6664 | 0.7173 | 0.6755 | |||
10 | 0.7601 | 0.7829 | 0.7602 | 0.7079 | 0.6778 | 0.6768 | 0.6937 | 0.7017 | 0.6860 | |||
Medium | 15 | 0.8205 | 0.8101 | 0.8062 | 0.7351 | 0.6716 | 0.6867 | 0.7081 | 0.6757 | 0.6777 | ||
20 | 0.8469 | 0.8647 | 0.8483 | 0.7514 | 0.7095 | 0.7146 | 0.7129 | 0.6529 | 0.6661 | |||
25 | 0.8772 | 0.8817 | 0.8731 | 0.7656 | 0.6992 | 0.7159 | 0.7209 | 0.6941 | 0.6958 | |||
Strong | 30 | 0.9092 | 0.9111 | 0.9050 | 0.7857 | 0.7062 | 0.7314 | 0.7324 | 0.7014 | 0.7037 | ||
40 | 0.9223 | 0.9423 | 0.9289 | 0.7995 | 0.7286 | 0.7503 | 0.7561 | 0.7280 | 0.7297 | |||
50 | 0.9313 | 0.9490 | 0.9371 | 0.8117 | 0.7547 | 0.7702 | 0.7789 | 0.7156 | 0.7339 |
Attack Level | Attac Strength | Traditional FDIA [8] | LMM-FDIA [18] | AttDiff-FDIA | ||||||||
Precision | Recall | -Score | Precision | Recall | -Score | Precision | Recall | -Score | ||||
Weak | 2 | 0.7038 | 0.7208 | 0.7117 | 0.6859 | 0.7404 | 0.7119 | 0.6669 | 0.7540 | 0.7064 | ||
5 | 0.7167 | 0.7437 | 0.7298 | 0.6927 | 0.7707 | 0.7293 | 0.6828 | 0.7456 | 0.7127 | |||
10 | 0.7671 | 0.7915 | 0.7602 | 0.7235 | 0.7583 | 0.7404 | 0.6972 | 0.7551 | 0.7248 | |||
Medium | 15 | 0.7958 | 0.8235 | 0.8062 | 0.7431 | 0.7351 | 0.7383 | 0.7203 | 0.6961 | 0.7060 | ||
20 | 0.8277 | 0.8976 | 0.8610 | 0.7547 | 0.6959 | 0.7240 | 0.7220 | 0.6695 | 0.6935 | |||
25 | 0.8679 | 0.9059 | 0.8864 | 0.7565 | 0.7253 | 0.7402 | 0.7334 | 0.6325 | 0.6787 | |||
Strong | 30 | 0.9075 | 0.9317 | 0.9194 | 0.7715 | 0.7086 | 0.7379 | 0.743 | 0.6395 | 0.6862 | ||
40 | 0.9484 | 0.9361 | 0.9421 | 0.8039 | 0.7286 | 0.7641 | 0.7651 | 0.6792 | 0.7190 | |||
50 | 0.9601 | 0.8864 | 0.9213 | 0.8248 | 0.6981 | 0.7558 | 0.7957 | 0.7144 | 0.7527 |
Attack Level | Attack Strength | Traditional FDIA [8] | LMM-FDIA [18] | AttDiff-FDIA | ||||||||
Precision | Recall | -Score | Precision | Recall | -Score | Precision | Recall | -Score | ||||
Weak | 2 | 0.7187 | 0.7181 | 0.7078 | 0.7199 | 0.7223 | 0.7074 | 0.6934 | 0.6977 | 0.6815 | ||
5 | 0.7664 | 0.7799 | 0.7650 | 0.7200 | 0.7521 | 0.7242 | 0.7042 | 0.7200 | 0.6990 | |||
10 | 0.8594 | 0.8605 | 0.8549 | 0.7404 | 0.7263 | 0.7215 | 0.7208 | 0.7177 | 0.7074 | |||
Medium | 15 | 0.9094 | 0.9250 | 0.9141 | 0.7549 | 0.7109 | 0.7159 | 0.7119 | 0.7214 | 0.7034 | ||
20 | 0.9507 | 0.9437 | 0.9452 | 0.7672 | 0.7272 | 0.7342 | 0.7205 | 0.7132 | 0.7052 | |||
25 | 0.9737 | 0.9609 | 0.9660 | 0.7743 | 0.7122 | 0.7285 | 0.7309 | 0.7130 | 0.7082 | |||
Strong | 30 | 0.9801 | 0.9789 | 0.9787 | 0.7850 | 0.7346 | 0.7485 | 0.7439 | 0.7227 | 0.7211 | ||
40 | 0.9922 | 0.9817 | 0.9864 | 0.7836 | 0.7219 | 0.7385 | 0.7629 | 0.7115 | 0.7247 | |||
50 | 0.9919 | 0.9900 | 0.9906 | 0.8051 | 0.7451 | 0.7639 | 0.7943 | 0.7208 | 0.7456 |
Attack Level | Attack Strength | Traditional FDIA [8] | LMM-FDIA [18] | AttDiff-FDIA | ||||||||
Precision | Recall | -Score | Precision | Recall | -Score | Precision | Recall | -Score | ||||
Weak | 2 | 0.7414 | 0.7723 | 0.7555 | 0.7737 | 0.7234 | 0.7472 | 0.7180 | 0.7508 | 0.7328 | ||
5 | 0.8171 | 0.9069 | 0.8593 | 0.7976 | 0.8309 | 0.8138 | 0.7277 | 0.6972 | 0.7112 | |||
10 | 0.8814 | 0.9246 | 0.9011 | 0.8270 | 0.7857 | 0.8054 | 0.7461 | 0.7098 | 0.7273 | |||
Medium | 15 | 0.9326 | 0.9334 | 0.9325 | 0.8317 | 0.8007 | 0.8157 | 0.7526 | 0.7548 | 0.7533 | ||
20 | 0.9562 | 0.9651 | 0.9605 | 0.8549 | 0.7868 | 0.8194 | 0.7721 | 0.7269 | 0.7485 | |||
25 | 0.9835 | 0.9819 | 0.9826 | 0.8705 | 0.8009 | 0.8340 | 0.7866 | 0.7460 | 0.7656 | |||
Strong | 30 | 0.9867 | 0.9881 | 0.9874 | 0.8828 | 0.8098 | 0.8442 | 0.8135 | 0.7936 | 0.8024 | ||
40 | 0.9993 | 0.9954 | 0.9973 | 0.9039 | 0.7797 | 0.8371 | 0.8417 | 0.7673 | 0.8024 | |||
50 | 1.0000 | 0.9987 | 0.9993 | 0.9234 | 0.7419 | 0.8220 | 0.8860 | 0.7651 | 0.8195 |
Attack Level | Attack Strength | Traditional FDIA [8] | LMM-FDIA [18] | AttDiff-FDIA | ||||||||
Precision | Recall | -Score | Precision | Recall | -Score | Precision | Recall | -Score | ||||
Weak | 2 | 0.7134 | 0.7293 | 0.710 | 0.6996 | 0.7330 | 0.7039 | 0.6880 | 0.7415 | 0.7043 | ||
5 | 0.7462 | 0.7644 | 0.7455 | 0.7174 | 0.7304 | 0.7107 | 0.7035 | 0.7280 | 0.7039 | |||
10 | 0.8235 | 0.8068 | 0.8072 | 0.7407 | 0.7309 | 0.7208 | 0.7106 | 0.7240 | 0.7049 | |||
Medium | 15 | 0.8652 | 0.8707 | 0.8628 | 0.7644 | 0.7457 | 0.7439 | 0.7106 | 0.7357 | 0.7118 | ||
20 | 0.9282 | 0.9162 | 0.9190 | 0.7856 | 0.7318 | 0.7461 | 0.7329 | 0.7134 | 0.7118 | |||
25 | 0.9493 | 0.9322 | 0.9382 | 0.7990 | 0.7221 | 0.7467 | 0.7424 | 0.7187 | 0.7194 | |||
Strong | 30 | 0.9571 | 0.9603 | 0.9571 | 0.8110 | 0.7554 | 0.7733 | 0.7513 | 0.7469 | 0.7381 | ||
40 | 0.9746 | 0.9741 | 0.9734 | 0.8227 | 0.7357 | 0.7691 | 0.7991 | 0.7441 | 0.7601 | |||
50 | 0.9836 | 0.9773 | 0.9797 | 0.8422 | 0.7668 | 0.7925 | 0.8364 | 0.7577 | 0.7861 |
Attack Level | Attack Strength | Traditional FDIA [8] | LMM-FDIA [18] | AttDiff-FDIA | ||||||||
Precision | Recall | -Score | Precision | Recall | -Score | Precision | Recall | -Score | ||||
Weak | 2 | 0.7263 | 0.6764 | 0.6960 | 0.7159 | 0.8198 | 0.7640 | 0.6963 | 0.7457 | 0.7192 | ||
5 | 0.7541 | 0.7241 | 0.7382 | 0.7353 | 0.8149 | 0.7730 | 0.7179 | 0.6841 | 0.6994 | |||
10 | 0.8359 | 0.8269 | 0.8305 | 0.7637 | 0.8015 | 0.7819 | 0.7204 | 0.6691 | 0.6928 | |||
Medium | 15 | 0.8815 | 0.8458 | 0.8630 | 0.7829 | 0.7701 | 0.7761 | 0.7219 | 0.6527 | 0.6853 | ||
20 | 0.9153 | 0.9364 | 0.9255 | 0.7971 | 0.7372 | 0.7657 | 0.7323 | 0.6584 | 0.6920 | |||
25 | 0.9341 | 0.9515 | 0.9427 | 0.8090 | 0.7355 | 0.7704 | 0.7456 | 0.7242 | 0.7327 | |||
Strong | 30 | 0.9538 | 0.9624 | 0.9580 | 0.8270 | 0.7113 | 0.7648 | 0.7608 | 0.7020 | 0.7272 | ||
40 | 0.9776 | 0.9731 | 0.9753 | 0.8409 | 0.7082 | 0.7688 | 0.8002 | 0.7195 | 0.7574 | |||
50 | 0.9873 | 0.9737 | 0.9804 | 0.8630 | 0.7151 | 0.7812 | 0.8269 | 0.7501 | 0.7861 |
Attack Level | Attack Strength | Traditional FDIA [8] | LMM-FDIA [18] | AttDiff-FDIA | ||||||||
Precision | Recall | -Score | Precision | Recall | -Score | Precision | Recall | -Score | ||||
Weak | 2 | 0.6883 | 0.6992 | 0.6819 | 0.6740 | 0.6958 | 0.6730 | 0.6469 | 0.7548 | 0.6892 | ||
5 | 0.7289 | 0.7233 | 0.7130 | 0.6831 | 0.7482 | 0.7045 | 0.6664 | 0.7173 | 0.6755 | |||
10 | 0.7601 | 0.7829 | 0.7602 | 0.7079 | 0.6778 | 0.6768 | 0.6937 | 0.7017 | 0.6860 | |||
Medium | 15 | 0.8205 | 0.8101 | 0.8062 | 0.7351 | 0.6716 | 0.6867 | 0.7081 | 0.6757 | 0.6777 | ||
20 | 0.8469 | 0.8647 | 0.8483 | 0.7514 | 0.7095 | 0.7146 | 0.7129 | 0.6529 | 0.6661 | |||
25 | 0.8772 | 0.8817 | 0.8731 | 0.7656 | 0.6992 | 0.7159 | 0.7209 | 0.6941 | 0.6958 | |||
Strong | 30 | 0.9092 | 0.9111 | 0.9050 | 0.7857 | 0.7062 | 0.7314 | 0.7324 | 0.7014 | 0.7037 | ||
40 | 0.9223 | 0.9423 | 0.9289 | 0.7995 | 0.7286 | 0.7503 | 0.7561 | 0.7280 | 0.7297 | |||
50 | 0.9313 | 0.9490 | 0.9371 | 0.8117 | 0.7547 | 0.7702 | 0.7789 | 0.7156 | 0.7339 |
Attack Level | Attac Strength | Traditional FDIA [8] | LMM-FDIA [18] | AttDiff-FDIA | ||||||||
Precision | Recall | -Score | Precision | Recall | -Score | Precision | Recall | -Score | ||||
Weak | 2 | 0.7038 | 0.7208 | 0.7117 | 0.6859 | 0.7404 | 0.7119 | 0.6669 | 0.7540 | 0.7064 | ||
5 | 0.7167 | 0.7437 | 0.7298 | 0.6927 | 0.7707 | 0.7293 | 0.6828 | 0.7456 | 0.7127 | |||
10 | 0.7671 | 0.7915 | 0.7602 | 0.7235 | 0.7583 | 0.7404 | 0.6972 | 0.7551 | 0.7248 | |||
Medium | 15 | 0.7958 | 0.8235 | 0.8062 | 0.7431 | 0.7351 | 0.7383 | 0.7203 | 0.6961 | 0.7060 | ||
20 | 0.8277 | 0.8976 | 0.8610 | 0.7547 | 0.6959 | 0.7240 | 0.7220 | 0.6695 | 0.6935 | |||
25 | 0.8679 | 0.9059 | 0.8864 | 0.7565 | 0.7253 | 0.7402 | 0.7334 | 0.6325 | 0.6787 | |||
Strong | 30 | 0.9075 | 0.9317 | 0.9194 | 0.7715 | 0.7086 | 0.7379 | 0.743 | 0.6395 | 0.6862 | ||
40 | 0.9484 | 0.9361 | 0.9421 | 0.8039 | 0.7286 | 0.7641 | 0.7651 | 0.6792 | 0.7190 | |||
50 | 0.9601 | 0.8864 | 0.9213 | 0.8248 | 0.6981 | 0.7558 | 0.7957 | 0.7144 | 0.7527 |
Attack Level | Attack Strength | Traditional FDIA [8] | LMM-FDIA [18] | AttDiff-FDIA | ||||||||
Precision | Recall | -Score | Precision | Recall | -Score | Precision | Recall | -Score | ||||
Weak | 2 | 0.7187 | 0.7181 | 0.7078 | 0.7199 | 0.7223 | 0.7074 | 0.6934 | 0.6977 | 0.6815 | ||
5 | 0.7664 | 0.7799 | 0.7650 | 0.7200 | 0.7521 | 0.7242 | 0.7042 | 0.7200 | 0.6990 | |||
10 | 0.8594 | 0.8605 | 0.8549 | 0.7404 | 0.7263 | 0.7215 | 0.7208 | 0.7177 | 0.7074 | |||
Medium | 15 | 0.9094 | 0.9250 | 0.9141 | 0.7549 | 0.7109 | 0.7159 | 0.7119 | 0.7214 | 0.7034 | ||
20 | 0.9507 | 0.9437 | 0.9452 | 0.7672 | 0.7272 | 0.7342 | 0.7205 | 0.7132 | 0.7052 | |||
25 | 0.9737 | 0.9609 | 0.9660 | 0.7743 | 0.7122 | 0.7285 | 0.7309 | 0.7130 | 0.7082 | |||
Strong | 30 | 0.9801 | 0.9789 | 0.9787 | 0.7850 | 0.7346 | 0.7485 | 0.7439 | 0.7227 | 0.7211 | ||
40 | 0.9922 | 0.9817 | 0.9864 | 0.7836 | 0.7219 | 0.7385 | 0.7629 | 0.7115 | 0.7247 | |||
50 | 0.9919 | 0.9900 | 0.9906 | 0.8051 | 0.7451 | 0.7639 | 0.7943 | 0.7208 | 0.7456 |
Attack Level | Attack Strength | Traditional FDIA [8] | LMM-FDIA [18] | AttDiff-FDIA | ||||||||
Precision | Recall | -Score | Precision | Recall | -Score | Precision | Recall | -Score | ||||
Weak | 2 | 0.7414 | 0.7723 | 0.7555 | 0.7737 | 0.7234 | 0.7472 | 0.7180 | 0.7508 | 0.7328 | ||
5 | 0.8171 | 0.9069 | 0.8593 | 0.7976 | 0.8309 | 0.8138 | 0.7277 | 0.6972 | 0.7112 | |||
10 | 0.8814 | 0.9246 | 0.9011 | 0.8270 | 0.7857 | 0.8054 | 0.7461 | 0.7098 | 0.7273 | |||
Medium | 15 | 0.9326 | 0.9334 | 0.9325 | 0.8317 | 0.8007 | 0.8157 | 0.7526 | 0.7548 | 0.7533 | ||
20 | 0.9562 | 0.9651 | 0.9605 | 0.8549 | 0.7868 | 0.8194 | 0.7721 | 0.7269 | 0.7485 | |||
25 | 0.9835 | 0.9819 | 0.9826 | 0.8705 | 0.8009 | 0.8340 | 0.7866 | 0.7460 | 0.7656 | |||
Strong | 30 | 0.9867 | 0.9881 | 0.9874 | 0.8828 | 0.8098 | 0.8442 | 0.8135 | 0.7936 | 0.8024 | ||
40 | 0.9993 | 0.9954 | 0.9973 | 0.9039 | 0.7797 | 0.8371 | 0.8417 | 0.7673 | 0.8024 | |||
50 | 1.0000 | 0.9987 | 0.9993 | 0.9234 | 0.7419 | 0.8220 | 0.8860 | 0.7651 | 0.8195 |
Attack Level | Attack Strength | Traditional FDIA [8] | LMM-FDIA [18] | AttDiff-FDIA | ||||||||
Precision | Recall | -Score | Precision | Recall | -Score | Precision | Recall | -Score | ||||
Weak | 2 | 0.7134 | 0.7293 | 0.710 | 0.6996 | 0.7330 | 0.7039 | 0.6880 | 0.7415 | 0.7043 | ||
5 | 0.7462 | 0.7644 | 0.7455 | 0.7174 | 0.7304 | 0.7107 | 0.7035 | 0.7280 | 0.7039 | |||
10 | 0.8235 | 0.8068 | 0.8072 | 0.7407 | 0.7309 | 0.7208 | 0.7106 | 0.7240 | 0.7049 | |||
Medium | 15 | 0.8652 | 0.8707 | 0.8628 | 0.7644 | 0.7457 | 0.7439 | 0.7106 | 0.7357 | 0.7118 | ||
20 | 0.9282 | 0.9162 | 0.9190 | 0.7856 | 0.7318 | 0.7461 | 0.7329 | 0.7134 | 0.7118 | |||
25 | 0.9493 | 0.9322 | 0.9382 | 0.7990 | 0.7221 | 0.7467 | 0.7424 | 0.7187 | 0.7194 | |||
Strong | 30 | 0.9571 | 0.9603 | 0.9571 | 0.8110 | 0.7554 | 0.7733 | 0.7513 | 0.7469 | 0.7381 | ||
40 | 0.9746 | 0.9741 | 0.9734 | 0.8227 | 0.7357 | 0.7691 | 0.7991 | 0.7441 | 0.7601 | |||
50 | 0.9836 | 0.9773 | 0.9797 | 0.8422 | 0.7668 | 0.7925 | 0.8364 | 0.7577 | 0.7861 |
Attack Level | Attack Strength | Traditional FDIA [8] | LMM-FDIA [18] | AttDiff-FDIA | ||||||||
Precision | Recall | -Score | Precision | Recall | -Score | Precision | Recall | -Score | ||||
Weak | 2 | 0.7263 | 0.6764 | 0.6960 | 0.7159 | 0.8198 | 0.7640 | 0.6963 | 0.7457 | 0.7192 | ||
5 | 0.7541 | 0.7241 | 0.7382 | 0.7353 | 0.8149 | 0.7730 | 0.7179 | 0.6841 | 0.6994 | |||
10 | 0.8359 | 0.8269 | 0.8305 | 0.7637 | 0.8015 | 0.7819 | 0.7204 | 0.6691 | 0.6928 | |||
Medium | 15 | 0.8815 | 0.8458 | 0.8630 | 0.7829 | 0.7701 | 0.7761 | 0.7219 | 0.6527 | 0.6853 | ||
20 | 0.9153 | 0.9364 | 0.9255 | 0.7971 | 0.7372 | 0.7657 | 0.7323 | 0.6584 | 0.6920 | |||
25 | 0.9341 | 0.9515 | 0.9427 | 0.8090 | 0.7355 | 0.7704 | 0.7456 | 0.7242 | 0.7327 | |||
Strong | 30 | 0.9538 | 0.9624 | 0.9580 | 0.8270 | 0.7113 | 0.7648 | 0.7608 | 0.7020 | 0.7272 | ||
40 | 0.9776 | 0.9731 | 0.9753 | 0.8409 | 0.7082 | 0.7688 | 0.8002 | 0.7195 | 0.7574 | |||
50 | 0.9873 | 0.9737 | 0.9804 | 0.8630 | 0.7151 | 0.7812 | 0.8269 | 0.7501 | 0.7861 |