Misinformation Really Does Spread like a Virus, Epidemiology Shows
流行病学表明,错误信息确实像病毒一样传播
Author: Sander van der Linden
桑德·范德林登
Length: • 4 mins • 4 分钟
Annotated by howie.serious
注释者:howie.serious
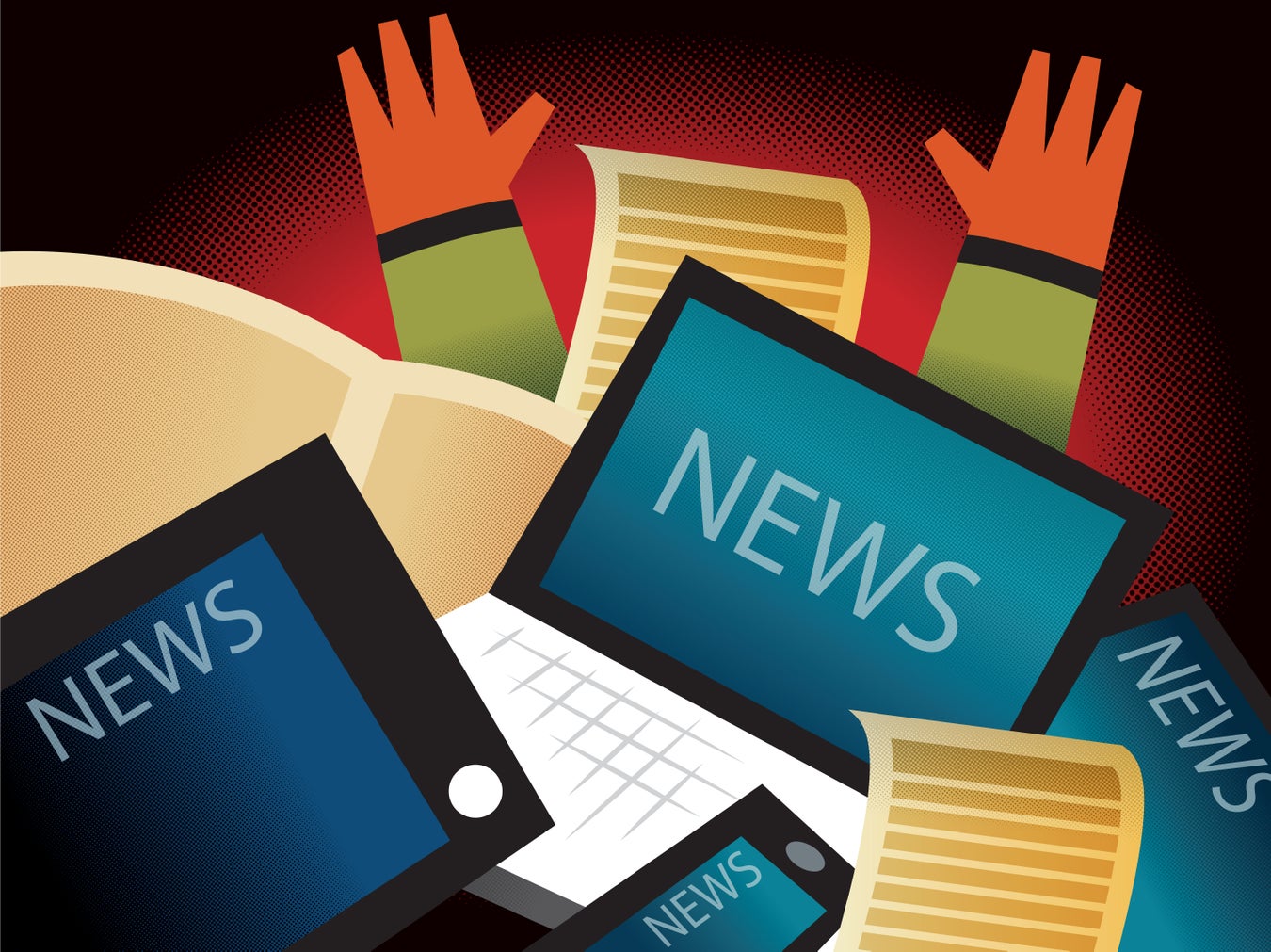
数学模型表明,错误信息确实像病毒一样传播。Paulo Buchinho/Getty Images
The following essay is reprinted with permission from The Conversation, an online publication covering the latest research.
以下文章经《对话》许可转载,这是一个涵盖最新研究的在线出版物。
We’re increasingly aware of how misinformation can influence elections. About 73% of Americans report seeing misleading election news, and about half struggle to discern what is true or false.
我们越来越意识到错误信息如何影响选举。大约 73%的美国人报告看到误导性的选举新闻,大约一半的人难以辨别真伪。
When it comes to misinformation, “going viral” appears to be more than a simple catchphrase. Scientists have found a close analogy between the spread of misinformation and the spread of viruses. In fact, how misinformation gets around can be effectively described using mathematical models designed to simulate the spread of pathogens.
当谈到错误信息时,“病毒式传播”似乎不仅仅是一个简单的流行语。科学家们发现错误信息的传播与病毒的传播有着密切的类比。事实上,错误信息的传播方式可以有效地用模拟病原体传播的数学模型来描述。
Concerns about misinformation are widely held, with a recent UN survey suggesting that 85% of people worldwide are worried about it.
人们普遍对错误信息表示担忧,最近的一项联合国调查显示,全球 85%的人对此感到担心。
These concerns are well founded. Foreign disinformation has grown in sophistication and scope since the 2016 US election. The 2024 election cycle has seen dangerous conspiracy theories about “weather manipulation” undermining proper management of hurricanes, fake news about immigrants eating pets inciting violence against the Haitian community, and misleading election conspiracy theories amplified by the world’s richest man, Elon Musk.
这些担忧是有道理的。自 2016 年美国大选以来,外国虚假信息在复杂性和范围上都有所增加。2024 年选举周期出现了关于“天气操控”的危险阴谋论,破坏了对飓风的正确管理,还有关于移民吃宠物的假新闻,引发了对海地社区的暴力行为,以及世界首富埃隆·马斯克放大的误导性选举阴谋论。
Recent studies have employed mathematical models drawn from epidemiology (the study of how diseases occur in the population and why). These models were originally developed to study the spread of viruses, but can be effectively used to study the diffusion of misinformation across social networks.
最近的研究采用了来自流行病学(研究疾病在人群中如何发生及其原因)的数学模型。这些模型最初是为研究病毒传播而开发的,但可以有效地用于研究社交网络上错误信息的传播。
One class of epidemiological models that works for misinformation is known as the susceptible-infectious-recovered (SIR) model. These simulate the dynamics between susceptible (S), infected (I), and recovered or resistant individuals (R).
一类适用于错误信息的流行病学模型被称为易感-感染-恢复(SIR)模型。这些模型模拟了易感(S)、感染(I)和恢复或抵抗个体(R)之间的动态。
These models are generated from a series of differential equations (which help mathematicians understand rates of change) and readily apply to the spread of misinformation. For instance, on social media, false information is propagated from individual to individual, some of whom become infected, some of whom remain immune. Others serve as asymptomatic vectors (carriers of disease), spreading misinformation without knowing or being adversely affected by it.
这些模型是由一系列微分方程生成的(帮助数学家理解变化率),并可以直接应用于错误信息的传播。例如,在社交媒体上,错误信息从个人传播到个人,其中一些人被感染,一些人保持免疫。其他人则作为无症状载体(疾病携带者),在不知情或不受不利影响的情况下传播错误信息。
These models are incredibly useful because they allow us to predict and simulate population dynamics and to come up with measures such as the basic reproduction (R0) number – the average number of cases generated by an “infected” individual.
这些模型非常有用,因为它们允许我们预测和模拟人口动态,并提出诸如基本再生数(R0)之类的措施——即由“感染”个体产生的平均病例数。
As a result, there has been growing interest in applying such epidemiological approaches to our information ecosystem. Most social media platforms have an estimated R0 greater than 1, indicating that the platforms have potential for the epidemic-like spread of misinformation.
因此,人们对将这种流行病学方法应用于我们的信息生态系统的兴趣日益浓厚。大多数社交媒体平台的估计 R0 大于 1,这表明这些平台具有类似流行病传播错误信息的潜力。
Looking for solutions 寻找解决方案
Mathematical modelling typically either involves what’s called phenomenological research (where researchers describe observed patterns) or mechanistic work (which involves making predictions based on known relationships). These models are especially useful because they allow us to explore how possible interventions may help reduce the spread of misinformation on social networks.
数学建模通常涉及所谓的现象学研究(研究人员描述观察到的模式)或机械工作(基于已知关系进行预测)。这些模型特别有用,因为它们使我们能够探索可能的干预措施如何帮助减少社交网络上错误信息的传播。
We can illustrate this basic process with a simple illustrative model shown in the graph below, which allows us to explore how a system might evolve under a variety of hypothetical assumptions, which can then be verified.
我们可以用下面图中显示的简单示例模型来说明这一基本过程,这使我们能够探索系统在各种假设条件下可能如何演变,然后可以进行验证。
Prominent social media figures with large followings can become “superspreaders” of election disinformation, blasting falsehoods to potentially hundreds of millions of people. This reflects the current situation where election officials report being outmatched in their attempts to fact-check minformation.
拥有大量追随者的知名社交媒体人物可能成为选举虚假信息的“超级传播者”,将虚假信息传播给可能数亿人。这反映了当前的情况,即选举官员在试图核实错误信息时感到力不从心。
In our model, if we conservatively assume that people just have a 10% chance of infection after exposure, debunking misinformation only has a small effect, according to studies. Under the 10% chance of infection scenario, the population infected by election misinformation grows rapidly (orange line, left panel).
在我们的模型中,如果我们保守地假设人们在接触后只有 10%的感染几率,根据研究,揭穿错误信息的效果很小。在 10%感染几率的情况下,选举错误信息感染的人群迅速增长(橙色线,左图)。
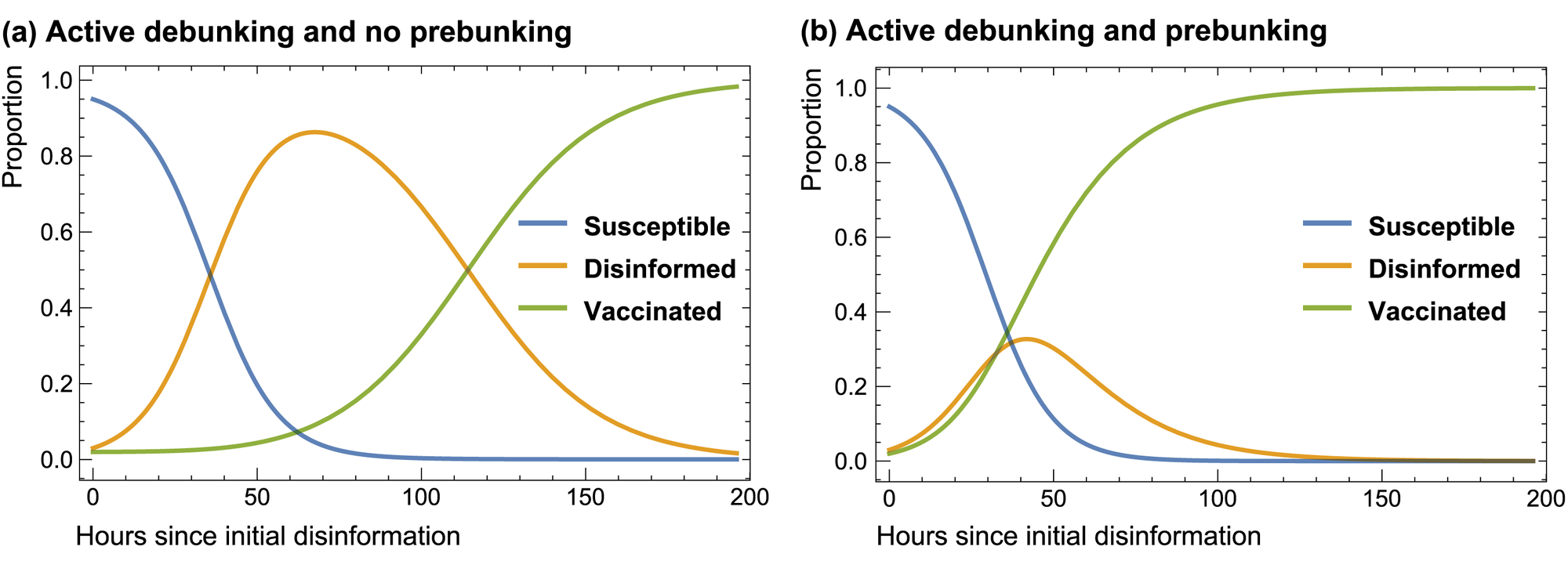
A ‘compartment’ model of disinformation spread over a week in a cohort of users, where disinformation has a 10% chance of infecting a susceptible unvaccinated individual upon exposure. Debunking is assumed to be 5% effective. If prebunking is introduced and is about twice as effective as debunking, the dynamics of disinformation infection change markedly.
一周内用户群体中错误信息传播的“隔离”模型,其中错误信息在接触后有 10%的几率感染易感的未接种个体。假设揭穿的效果为 5%。如果引入预揭穿,其效果大约是揭穿的两倍,错误信息感染的动态会显著改变。
Sander van der Linden/Robert David Grimes
桑德·范德林登/罗伯特·大卫·格里姆斯
Psychological ‘vaccination’
心理“疫苗接种”
The viral spread analogy for misinformation is fitting precisely because it allows scientists to simulate ways to counter its spread. These interventions include an approach called “psychological inoculation”, also known as prebunking.
错误信息的病毒传播类比非常贴切,因为它允许科学家模拟对抗其传播的方法。这些干预措施包括一种称为“心理免疫”的方法,也称为预揭穿。
This is where researchers preemptively introduce, and then refute, a falsehood so that people gain future immunity to misinformation. It’s similar to vaccination, where people are introduced to a (weakened) dose of the virus to prime their immune systems to future exposure.
这是研究人员预先引入并随后驳斥谬误的地方,以便人们在未来对错误信息产生免疫力。这类似于疫苗接种,人们接触到(减弱的)病毒剂量,以使他们的免疫系统对未来的暴露做好准备。
For example, a recent study used AI chatbots to come up with prebunks against common election fraud myths. This involved warning people in advance that political actors might manipulate their opinion with sensational stories, such as the false claim that “massive overnight vote dumps are flipping the election”, along with key tips on how to spot such misleading rumours. These ‘inoculations’ can be integrated into population models of the spread of misinformation.
例如,最近的一项研究使用人工智能聊天机器人来提出针对常见选举欺诈谣言的预防措施。这涉及提前警告人们,政治行为者可能会用耸人听闻的故事操纵他们的意见,例如“巨大的隔夜投票倾斜选举”的虚假说法,并提供识别此类误导性谣言的关键提示。这些“免疫接种”可以整合到错误信息传播的人口模型中。
You can see in our graph that if prebunking is not employed, it takes much longer for people to build up immunity to misinformation (left panel, orange line). The right panel illustrates how, if prebunking is deployed at scale, it can contain the number of people who are disinformed (orange line).
你可以在我们的图表中看到,如果不使用预防措施,人们需要更长的时间来建立对错误信息的免疫力(左图,橙色线)。右图说明了如果大规模部署预防措施,可以控制被误导的人数(橙色线)。
The point of these models is not to make the problem sound scary or suggest that people are gullible disease vectors. But there is clear evidence that some fake news stories do spread like a simple contagion, infecting users immediately.
这些模型的目的不是让问题听起来可怕或暗示人们是易受感染的疾病载体。但有明确的证据表明,一些假新闻故事确实像简单的传染病一样传播,立即感染用户。
Meanwhile, other stories behave more like a complex contagion, where people require repeated exposure to misleading sources of information before they become “infected”.
与此同时,其他故事的行为更像是复杂的传染病,人们需要反复接触误导性信息来源后才会“感染”。
The fact that individual susceptibility to misinformation can vary does not detract from the usefulness of approaches drawn from epidemiology. For example, the models can be adjusted depending on how hard or difficult it is for misinformation to “infect” different sub-populations.
个人对错误信息的易感性可能有所不同,但这并不影响从流行病学中借鉴的方法的有效性。例如,可以根据错误信息“感染”不同子群体的难易程度来调整模型。
Although thinking of people in this way might be psychologically uncomfortable for some, most misinformation is diffused by small numbers of influential superspreaders, just as happens with viruses.
尽管以这种方式看待人们可能会让一些人感到心理不适,但大多数错误信息是由少数有影响力的超级传播者传播的,就像病毒一样。
Taking an epidemiological approach to the study of fake news allows us to predict its spread and model the effectiveness of interventions such as prebunking.
采用流行病学方法研究假新闻可以让我们预测其传播,并模拟干预措施(如预防性反驳)的有效性。
Some recent work validated the viral approach using social media dynamics from the 2020 US presidential election. The study found that a combination of interventions can be effective in reducing the spread of misinformation.
最近的一些研究使用 2020 年美国总统大选的社交媒体动态验证了病毒传播的方法。研究发现,结合多种干预措施可以有效减少错误信息的传播。
Models are never perfect. But if we want to stop the spread of misinformation, we need to understand it in order to effectively counter its societal harms.
模型从来都不完美。但如果我们想要阻止错误信息的传播,我们需要理解它,以便有效地对抗其对社会的危害。
This article was originally published on The Conversation. Read the original article.
本文最初发表在 The Conversation 上。阅读原文。