DeepSeek, Huawei, Export Controls, and the Future of the U.S.-China AI Race
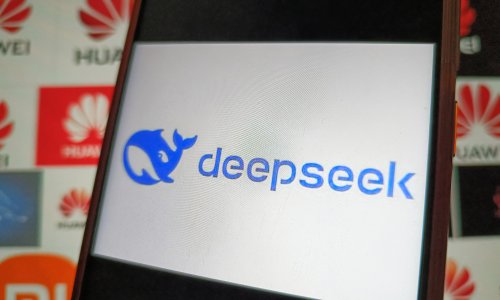
Photo: CFOTO/Future Publishing/Getty Images
Available Downloads
Introduction
Six months ago, few in the West aside from obsessive AI professionals had heard of DeepSeek, a Chinese AI research lab founded barely more than a year and a half ago. Today, DeepSeek is a global sensation attracting the attention of heads of state, global CEOs, top investors, and the general public.
With the release of its R1 model on January 20, 2025—the same day as President Trump’s second inauguration—DeepSeek has cemented its reputation as the top frontier AI research lab in China and caused a reassessment of assumptions about the landscape of global AI competition. By January 27, DeepSeek’s iPhone app had overtaken OpenAI’s ChatGPT as the most-downloaded free app on Apple’s U.S. App Store. The stock prices of some U.S. tech companies briefly tumbled, including the AI chip designer Nvidia, which lost more than $600 billion off its valuation in a single day. (AI chips are also known as graphics processing units, or GPUs, and the terms are used interchangeably in this report.)
ChatGPT has again overtaken DeepSeek in app store rankings, and Nvidia’s stock price has since mostly recovered. However, investor interest in Chinese tech companies has grown significantly and remains elevated. DeepSeek is now even reportedly seeking investment from venture capital firms.
As a sector, AI is prone to overreactions and wild swings in perception. One might think that the story of DeepSeek is just another overblown AI hype cycle. However, the extraordinary attention focused on DeepSeek is justified, even if the conclusions some have drawn from its success are not. It would be a great mistake for U.S. policymakers to ignore DeepSeek or to suggest that its accomplishments are merely a combination of intellectual property theft and misleading Chinese propaganda. Policymakers need to understand that—even while DeepSeek has in some cases simply implemented innovations already known to U.S. AI companies—DeepSeek has also demonstrated genuine technological breakthroughs of its own. These facts deserve careful consideration as the second Trump administration sets its AI policy agenda.
This paper provides an overview of DeepSeek’s origins and achievements, their geopolitical implications, and the key challenges facing U.S. and allied policymaking as a result. The paper pays particular attention to DeepSeek’s implications for the future of AI and semiconductor export control policy. It concludes that—while DeepSeek’s success does partly reflect failures of earlier implementations of U.S. export controls—these controls can continue to play a critical role in supporting the United States’ strategy for winning the AI race against China. Success is not guaranteed and will require two things:
(1) Preventing large-scale AI chip smuggling and
(2) Preventing the team of Huawei and SMIC from providing a viable Chinese AI chip alternative to the leading incumbent international team of Nvidia and TSMC.
Both will be exceedingly difficult, and in both cases export controls can at best slow and disrupt Chinese efforts, not stop them.
Regarding the second point, Chinese sources and leaders are expressing renewed optimism. At a meeting on February 17 between Chinese Community Party (CCP) Chairman Xi Jinping and Chinese technology executives (including DeepSeek CEO Liang Wenfeng), Huawei founder Ren Zhengfei told Xi that his previous concerns about the lack of domestic advanced semiconductor production and the damaging impacts of U.S. export controls had eased because of recent breakthroughs by Huawei and its partners. Ren further said that he is leading a network of more than 2,000 Chinese companies who are collectively working to ensure that China achieves self-sufficiency of more than 70 percent across the entire semiconductor value chain by 2028. These predictions should be taken seriously. While China’s economy faces many challenges, Gerard DiPippo of RAND is correct to argue,
Even if overall economic growth remains comparatively weak and many Chinese firms continue to struggle, China’s central and local governments will keep supporting high-tech industries and emerging stars like DeepSeek. . . . Western policymakers shouldn’t make the mistake of believing China is down and out, and they shouldn’t be surprised when China continues to catch up or make breakthroughs in critical and emerging technologies.
What follows are a series of 21 key judgments regarding DeepSeek, Huawei, export controls, and the future of U.S.-China AI competition.
Readers are advised to pay special attention to the discussion of Huawei and SMIC, as this report contains a great deal of new and previously private information regarding Huawei’s progress toward indigenizing China’s AI and semiconductor technology stack.
- DeepSeek did not come out of nowhere. Its parent company, High-Flyer Capital Management, has roots in AI-enabled high frequency trading that provided a strong technical foundation in terms of both computing infrastructure and workforce talent.
DeepSeek is a subsidiary of High-Flyer Capital Management, a Chinese quantitative hedge fund focused on algorithmic trading powered by deep learning. Deep learning is the core technology paradigm underlying the past two decades of rapid AI advancements. Cofounded in 2015 by Liang Wenfeng, who is also the founder and CEO of DeepSeek, High-Flyer was already ranked as the top Chinese hedge fund in 2019.
DeepSeek’s heritage via High-Flyer in the algorithmic trading world helps to explain its success in frontier AI research and especially its success with its most recent model releases. Algorithmic trading firms frequently own and operate their own data center infrastructure, which they use to develop proprietary investment strategies powered by machine learning artificial intelligence.
High-Flyer has admitted that its activities include “High-Frequency Trading,” though High-Flyer de-emphasizes this work since it is politically controversial (and was for a time banned) in China. High-frequency trading firms race against each other to be the fastest asset traders reacting to new market developments and are obsessively focused on optimizing and accelerating their computing infrastructure. These efforts routinely include intense research on developing proprietary and unorthodox data center engineering methods for improved speed and efficiency. Firms can justify making these expensive investments in computing and network infrastructure optimization because the success or failure of trades collectively worth billions of dollars depends upon reducing the time between learning of a fact and making a stock trade based on that new fact by mere nanoseconds (billionths of a second).
High-Flyer is among the leaders of such firms in China and has long been among the most focused on both AI algorithms for trading and data center engineering. The Financial Times reported that, by mid-2022, High-Flyer had already acquired more than 10,000 Nvidia A100 chips (which at the time were the most advanced AI chips in the world) and spent more than 1.2 billion RMB (roughly $180 million at the time) to build two data centers. These infrastructure investments distinguished High-Flyer from most other Chinese companies: Only four other companies in China possessed such a large quantity of chips at the time, and all were major tech companies, not finance companies. In a 2023 interview, Liang said:
We always wanted to carry out larger-scale experiments, so we’ve always aimed to deploy as much computational power as possible. . . . We wanted to find a paradigm that can fully describe the entire financial market.
In another 2023 interview with a Chinese media outlet, Liang provided a timeline for High-Flyer’s AI chip compute reserves as a gradual “progression from one GPU in the beginning, to 100 GPUs in 2015, 1,000 GPUs in 2019.” He stated that, from the day of DeepSeek’s 2023 founding onward, High-Flyer had both the computational power and “ample R&D budgets” to support DeepSeek’s mission of “research and exploration” in pursuit of artificial general intelligence (AGI).
DeepSeek’s heritage from High-Flyer’s machine learning research and intensive computing optimization techniques are evident in some of the strategies that it has pursued to develop its AI models. More broadly, the synergy of technical skills between the world of algorithmic trading and AI research is well known. For example, industry sources at leading U.S. AI labs told CSIS that they have also focused on recruiting talent, especially compute infrastructure engineers, with experience in the high-frequency trading industry. Both sectors pay such talented individuals handsomely.
- DeepSeek’s technological achievements in terms of performance and cost were not surprising. They reflected a continuation of longstanding trends. However, the fact that such achievements came from a Chinese lab was a surprise.
DeepSeek’s recent technological achievements (reflected in the DeepSeek-V2, DeepSeek-V3, DeepSeek-R1, DeepSeek Math, and Native Sparse Attention technical papers) can most easily be understood through two basic facts:
First, DeepSeek has demonstrated a suite of algorithmic and architectural improvements that significantly reduce the amount of computing power (and therefore financial investment) required for any AI model to reach and operate at a given level of performance. This is true for both the training stage (in which AI models are created) and the inference stage (in which their capabilities are used to serve internal or external customers).
Second, DeepSeek has now developed AI models in China that are broadly comparable to the best U.S.-developed AI models introduced in mid-2024 (e.g., OpenAI’s o1 or Anthropic’s Claude 3.5 Sonnet).
Of the two demonstrations, the fact that these advances came from China is more surprising than the fact that they happened at all. DeepSeek’s improvements in technical performance were consistent with preexisting trend lines of AI performance improvements. They represent expected performance breakthroughs, not shocking ones.
To understand why, it is helpful to consider an analogy in the form of Moore’s Law. In economic terms, Moore’s Law essentially means that computer chips improve to be roughly twice as good in performance for the same amount of money roughly every two years. Though the nature of that performance improvement has changed, this trend has been consistently observed for almost six decades (with some caveats).
As Moore’s Law has been repeatedly validated since the 1960s, it has become steadily easier to confidently predict that computing performance per dollar will continue to double every two years. But, as the fortunes of Intel vividly demonstrate, that is not synonymous with being able to state with confidence which specific company or which specific technical innovations will deliver that improved performance. The same is true of AI today: It is easier to forecast the degree of AI performance growth than to forecast who will invent that performance growth or how.
AI has its own versions of Moore’s Law that are driving exponential growth in both absolute performance and performance available at a given cost. At a high level, these sources of improvement can be grouped into three types:
- Increasing available computing resources (either by using better computer chips, more chips, or both);
- Improving algorithms and architectures (for example, to deliver more “intelligence” for a given compute budget); and
- Improving the quality and/or quantity of available training data.
Epoch AI, an AI-focused nonprofit research institute, has estimated that, while the amount of human-generated training data will likely plateau in 2028, algorithmic and architectural improvements and increased computing resources have been improving at 3x per year and 4.6x per year respectively—for a combined multiplier effect of 13.8x per year. In short, AI performance is increasing at a rate far faster than the familiar (though still extraordinary) pace of exponential progress described by Moore’s Law. Even ignoring the possible remaining improvements from harvesting additional training data, a (highly oversimplified) back-of-the-envelope estimate of future performance based on the compute trends and algorithmic architectural trends would suggest that AI performance at the end of 2028 will be more than 36,000 times better than at the beginning of 2025, rather than just the four or eight times better that would be true if improvement were only occurring at the familiar pace of Moore’s Law.
For those who only began paying attention to AI with the late 2022 launch of ChatGPT, this pace of progress can be both breathtaking and difficult to believe. However, for those familiar with the AI industry’s history over the past two decades, the fact that an AI model that requires a massive data center one year can run on a laptop or a single AI chip a few years later is well-known as a recurring, though still quite incredible, phenomenon. The dramatic reduction in cost-per-performance DeepSeek demonstrated is not a surprise to Western AI researchers. They have been leaders in driving—and benefitting from—the exact same trend for more than a decade. One might ask: “If that is the case, why are U.S. AI labs still building ever larger and more powerful data centers?” Put simply, such companies are reinvesting all efficiency gains toward improved overall performance. Dario Amodei, CEO of the U.S. AI lab Anthropic, put it this way:
Because the value of having a more intelligent system is so high, this [reduced cost] shifting of the curve typically causes companies to spend more, not less, on training models: the gains in cost efficiency end up entirely devoted to training smarter models, limited only by the company’s financial resources.
Moreover, AI researchers are clearly nowhere near the absolute ceiling of computational performance and efficiency. The human brain is a real-world existence proof of human-level intelligence that requires only 20 watts of electricity, compared to the megawatts or gigawatts contemplated for future AI data centers. Despite the many differences between human brains and machine intelligence, the existence of the brain hints at future intelligence efficiency possibilities in much the same way that bird wings inspired the Wright brothers.
Thus, it is not at all surprising that somewhere on earth, an AI research lab managed to deliver the improved AI performance-per-dollar that DeepSeek’s technical papers demonstrate. The only surprise is that the research lab that discovered some of the relevant technical innovations is a Chinese one, and notably one whose technical team appears to have been educated and trained almost entirely in China, rather than at Western universities or Western companies.
While Chinese researchers have been at or close to world class in many domains of AI research for many years, DeepSeek represents the first time that a Chinese AI lab has demonstrated breakthroughs at or near the absolute frontier of foundational AI research. Many of the techniques that DeepSeek demonstrated are now the new state-of-the-art. Of note, this is exactly what the Chinese government set as a goal in China’s 2017 official AI strategy:
By 2025, China will achieve major breakthroughs in basic theories for AI, such that some technologies and applications achieve a world-leading level and AI becomes the main driving force for China’s industrial upgrading and economic transformation.
- DeepSeek’s technological innovations are real, not propaganda. They have been in all cases proven to work by Western researchers who replicated DeepSeek’s approach.
DeepSeek R1论文发表后的第一周,一些评论家认为DeepSeek的结果是编造的,是中国宣传努力或 "心理战 "的一部分。这是完全错误的。美国大学的研究人员已经复制了 DeepSeek V3 和 R1 论文中描述的部分研究成果,而美国的人工智能公司也已经在努力调整 DeepSeek 的新技术,将其用于自己的人工智能工作中。
正如本文将进一步讨论的那样,DeepSeek 的所有技术创新基本上都与算法和架构改进有关。DeepSeek并不具备卓越的计算硬件,也无法访问可能比西方研究人员更具优势的独特重要数据集(不包括合成数据)。DeepSeek 在 DeepSeek-V2(2024 年 5 月)、DeepSeek-V3(2024 年 12 月)、DeepSeek-R1(2025 年 1 月)和其他研究论文中展示的关键技术创新意义重大。其中一些创新已被美国公司独立发现(但未公开披露),但另一些则是真正的新技术。
DeepSeek 的成功在很大程度上反映了 2022 年 10 月美国第一套人工智能芯片出口管制措施的缺陷所产生的滞后影响。美国政府在 2023 年 10 月的更新中承认并部分弥补了这些缺陷。
美国和中国的许多媒体和政策评论家都认为,DeepSeek 的效率提升证明了美国的出口管制未能限制中国的人工智能行业,DeepSeek 证明了开发和服务高性能人工智能能力并不需要大量的美国尖端人工智能芯片。第一种说法有一定道理,但第二种说法是错误的。
首先,DeepSeek 的 V3 研究论文指出,他们使用 Nvidia H800 芯片对模型进行了 278.8 万 GPU 小时的训练,按每个 GPU 小时 2 美元的成本估算,相当于 557.6 万美元。
H800 是 H100 芯片的降级版,是 Nvidia 为中国市场专门开发的,以符合 2022 年 10 月 7 日实施的美国出口管制。特别是,这些管制措施限制销售超过总处理能力和互连速度这两个指标性能阈值的芯片。
政府消息人士告诉 CSIS,美国政府知道这些限制将阻止 Nvidia 运输其(当时)市场领先的 A100 芯片和即将推出的 H100 芯片。他们推断,中国客户将被限制使用 V100,该芯片于 2017 年首次推出,性能明显低于其后续产品。此外,美国政府还假设,如果 Nvidia 专门为中国市场开发一款新芯片,该芯片在一项指标上超过出口管制性能阈值,但在另一项指标上未超过阈值,这将需要典型的多年 AI 芯片开发时间表。
然而,制定 2022 年 10 月出口管制政策的美国政府设计者没有意识到的是,Nvidia 拥有对其现有芯片产品进行制造后修改的机制。具体来说,Nvidia 的每款芯片都经过冗余、可恢复和缺陷容忍度设计,以最大限度地减少制造缺陷的影响。这种缺陷是先进节点半导体行业的一个重大问题,而设计芯片以减轻其潜在影响几乎是普遍现象。Nvidia 可以烧断芯片上一个或多个战略性放置的电气保险丝,因此制造缺陷通常只会降低芯片性能或停用冗余组件,而不会导致整个芯片失效。行业消息来源向 CSIS 证实,Nvidia 熔断了 A100 芯片上的保险丝,使其互连速度(而非处理能力)低于出口管制性能阈值,从而形成了 A800 产品线。
从本质上讲,A100 诞生时就具备了在必要时通过熔断器设置变为 A800 的能力。相比之下,面对出口管制,Nvidia 对 H800 进行了一些小的重新设计和设计优化。这使得 Nvidia 可以在不违反出口管制的情况下,继续向中国大量出货先进的 AI 芯片(性能接近全球最先进水平),而无需花费数年时间专门为中国市场设计新芯片。根据 The Information 的报道,A800 和 H800 芯片的性能与原版芯片的性能非常接近,因此在 A800 和 H800 上市期间,实际上不存在向中国大规模走私 AI 芯片的需求。既然合法购买的芯片已经足够好,为什么还要走私呢?
拜登政府最终意识到,A800 和 H800 的持续销售意味着他们的政策不会对中国的人工智能生态系统产生预期影响。前政府官员告诉 CSIS,到 2022 年 12 月,这一点在内部已经很清楚了。然而,美国政府在最初的一揽子出口管制措施出台 12 个月后才采取行动。美国政府于 2023 年 10 月修改了出口管制的性能阈值,阻止向中国出口 A800、H800 以及任何性能相当的非英伟达芯片。
在 A800 和 H800 芯片对华出口合法的一年时间里,有大量此类芯片销往中国。虽然没有精确的销售数字,但据 Nvidia 披露,在 2022 年 10 月 31 日至 2023 年 10 月 31 日期间,其对华销售额超过了 90 亿美元。不过,这其中确实包括了未广泛用于人工智能训练或推理的芯片收入。Nvidia 按计费地点划分的季度收入见表 1。
如果 DeepSeek 承认在其数据中心使用 H100 训练其模型,那么根据美国法律,DeepSeek 将承认其活动是非法的,因为 H100 从未在中国合法上市,甚至中国公司在中国境外的子公司也无法合法购买。因此,如果 DeepSeek 确实从事了非法活动,它就有动机隐瞒这一事实。这就提出了一个问题:DeepSeek 在其出版物中声称只使用 H800 芯片时是否撒了谎。
行业分析师本-汤普森(Ben Thompson)指出,有确凿证据表明 DeepSeek 确实如其宣称的那样使用了 H800:DeepSeek 的许多算法和架构改进都非常适合在互联带宽有限的条件下最大限度地有效利用计算资源。这至少有力地表明,DeepSeek 在其计算基础设施中使用了许多 H800,而且具有 V3 性能的模型确实可以完全在 H800 上进行训练。
不过,这并不能证明 DeepSeek 在其整体计算基础设施中只使用 H800。事实上,中国新闻媒体的一些报道称,DeepSeek确实在英伟达H100芯片上训练了其稍新的R1模型,而根据美国出口管制,英伟达H100芯片是禁止从中国出口的。半导体咨询公司 SemiAnalysis 最近援引匿名行业消息人士的话写道,DeepSeek 总共拥有 50,000 个 Hopper 代 GPU,其中包括 H100、H800 和 H20。SemiAnalysis特别声称,它有证据表明,High-Flyer/DeepSeek的计算基础设施在其总计算堆栈中至少包括1万个H100、1万个H800、3万个H20和1万个A100。SemiAnalysis 还估计,High-Flyer/DeepSeek 仅在 GPU 服务器资本支出方面就花费了 16.3 亿美元(即不包括其他数据中心建设和运营成本)。
艾伦人工智能研究所的机器学习研究员内森-兰伯特正确地指出
仅仅通过最终的预训练运行来跟踪一个项目所使用的计算资源,是估算实际成本的一种非常无益的方法。对于了解计算的实际利用率和底层学习的效率来说,这是一个非常有用的衡量标准,但根据最终运行所用 GPU 的市场价格为模型分配成本则具有欺骗性。
然而,在这种情况下,并不是 DeepSeek 在欺骗,因为他们的论文非常清楚地说明了 560 万美元的数字中包含了什么和不包含什么。这只是最后一次成功的预训练运行。它不包括为产生最终运行所需的洞察力而事先进行的数百次实验的计算成本,也不包括与训练后微调或推理计算工作量相关的计算成本。
虽然没有公开证据表明在 2023 年 10 月之前发生过大规模的 Nvidia 芯片走私活动,但业内人士告诉 CSIS,到 2024 年初,大规模的 H100 芯片走私活动已经开始。2024 年年中,The Information 的记者采访了八个不同的 H100 芯片走私网络的参与者,每个网络都提供了证据,证明他们完成了价值超过 1 亿美元的中国交易。这些网络继续活跃,躲避侦查的技术也越来越高超。例如,《信息》在 2024 年 12 月的调查中发现
在接到即将接受检查的通知后,走私分子复制了他们从超微公司购买的装有 Nvidia 芯片(已走私到中国)的服务器的序列号,并将其安装到他们能接触到的其他服务器上。
有证据表明,各国政府越来越重视打击人工智能芯片走私。在最近结束的财年中,英伟达 18% 的营收来自新加坡,但只有 2% 的营收来自新加坡。新加坡官员最近逮捕了三名被控协助向中国走私人工智能芯片的人。新加坡政府更加重视打击走私活动,这可能反映了其对在拜登政府的人工智能扩散框架下被降级为二级国家的担忧。
然而,DeepSeek 所面临的挑战也反映了人工智能芯片出口管制政策所取得的成功,DeepSeek 首席执行官将其描述为公司和更广泛的中国人工智能生态系统所面临的最大挑战。
与 2023 年 8 月华为推出 Mate60 Pro 5G 智能手机一样,许多评论家迅速强调 DeepSeek 如何反映了美国出口管制的失败。这其中包括中国国有媒体,其中一家媒体《环球视线》(GlobalVision)播报说:"DeepSeek 和华为联手!......突破美国技术限制并非不可能!"
古尔德法学院教授 Angela Zhang 更进一步指出:
中国在效率方面取得的成就绝非偶然。它们是对美国及其盟国不断升级的出口限制的直接回应。美国限制中国获得先进的人工智能芯片,无意中刺激了中国的创新。
这种说法在某种意义上是正确的:像 DeepSeek 这样的中国公司优先考虑效率创新,特别是在 H800 的局限性下,效率创新是非常有用的,因为这是对他们最有吸引力的人工智能研究途径。
然而,这种说法存在很大缺陷,原因有三。首先,每一家领先的人工智能公司,包括美国和中国的公司,都在追求提高效率。如上所述,DeepSeek 的效率提升代表了人工智能计算效率提升方面既有行业趋势的延续,而非急剧加速。甚至在 DeepSeek 成立之前,甚至在美国实施出口管制之前,DeepSeek 的母公司就已经执着地专注于提高计算效率(尽管更侧重于优化延迟)。如果说出口管制导致人工智能公司做了一些它们--无论是美国还是中国--在出口管制实施之前就已经在做(并且已经有了巨大的经济动机去做)的事情,那就太奇怪了。
其次,这一论点存在缺陷,因为它没有涉及 "在没有出口管制的情况下,中国还能走多远?"这一问题。目前地球上运行的最大人工智能计算集群是 xAI 位于田纳西州孟菲斯市的 Colossus 超级计算机,它最近进行了升级,使用了 20 万个 H100 芯片。考虑到在出口管制生效之前,中国的领先企业已经拥有了更多的人工智能相关专利,发表了近乎同样多的高引用率研究论文,在数据中心资本支出上也花费了近乎同样多的资金,如果没有出口管制,中国完全有可能在人工智能领域领先于美国。瓦德瓦尼人工智能中心(Wadhwani AI Center)的非常驻高级助理吉米-古德里奇(Jimmy Goodrich)说得好:"众所周知,DeepSeek 拥有一支非常优秀的团队,如果他们能获得更多的计算能力,天知道他们会有多大的能力。"古德里奇关于 DeepSeek 的说法适用于整个中国。
第三,这一论点没有考虑到重新分配高性能芯片供应的好处,否则这些芯片将流向中国。据估计,Nvidia 在人工智能芯片领域的全球市场份额为 90%,它受到的是供应限制,而不是需求限制。自 2022 年以来,台积电能够生产的每一块 Nvidia 芯片,都有许多客户愿意购买。甲骨文公司联合创始人拉里-埃里森(Larry Ellison)在 2024 年 9 月讲述他自己、xAI 首席执行官埃隆-马斯克(Elon Musk)和 Nvidia 首席执行官黄仁勋(Jensen Huang)之间的一次晚宴时,绘声绘色地描述了 Nvidia 的客户如何不得不乞求获得更多的芯片分配:
我会把这次晚宴形容为甲骨文和埃隆向詹森乞讨 GPU,[说]"请收下我们的钱。请收下我们的钱。对了,我买了晚餐。不,不,多拿点。我们需要你多收点我们的钱,拜托了。"
这意味着,由于人工智能芯片短缺始于 2022 年,在没有出口管制的情况下,Nvidia 出售给中国的所有芯片都将以牺牲其他地方的客户为代价,而在这种情况下,其他地方的客户绝大多数是美国。换句话说,取消出口管制不仅会帮助中国,也会损害美国,至少在 Nvidia 芯片分配方面是如此。
DeepSeek 首席执行官梁文峰则不认为芯片出口管制对中国或 DeepSeek 有任何帮助。他在 2024 年 7 月接受采访时说:"我们短期内没有融资计划。对我们来说,钱从来都不是问题,先进芯片的运输禁令才是问题所在。"梁建章在接受一家中国媒体采访时还表示,美国对人工智能芯片的限制意味着中国公司必须使用两到四倍的计算能力才能达到同样的效果,他指的是使用 H800 而不是 H100 进行大型模型训练的惩罚。
DeepSeek 在用户数量大幅增长的情况下经历了大面积中断,不得不停止新用户注册,这一事实进一步证明了美国出口管制的影响。虽然 DeepSeek 声称这些中断是网络攻击造成的,但更有可能的解释是,他们只是缺乏足够的计算能力来满足日益增长的用户群。为少数用户提供人工智能大型语言模型所需的计算能力与 OpenAI 每周为 4 亿活跃用户提供人工智能模型所需的计算能力有很大不同。最近,DeepSeek 再次开放了新用户注册,这很可能反映出该公司成功购买或租用了额外的计算能力。尽管如此,问题依然存在:计算能力很重要。
DeepSeek发现的提高人工智能计算效率的技术可能会让美国公司比中国公司受益更多,因为美国公司可以将这些技术应用于其庞大得多的计算资源,从而为更多客户提供更好的人工智能服务。
DeepSeek 最近的一则招聘广告在描述其创造 AGI 的目标时说:"我们相信,AGI 是模型 x 数据 x 计算能力的暴力之美。虽然有些过于简单化,但这个公式本质上仍然是正确的,并有助于解释为什么在任何计算效率水平上,拥有更大的计算资源都是一个有用的优势。
DeepSeek 的所有创新都是算法和架构方面的。DeepSeek 似乎在其研究论文中详细描述了所有或几乎所有这些创新。这意味着,美国的人工智能实验室可以自由地将这些创新应用于训练和部署自己的人工智能模型。事实上,美国公司已经在这样做了。
但是,虽然 DeepSeek 的算法创新可以被美国公司复制,但 DeepSeek 将难以复制美国的人工智能芯片和计算优势。由于缺乏极紫外光刻(EUV)技术,中国最先进的人工智能芯片设计公司和逻辑芯片制造商--华为和中芯国际--很可能多年来仍将停留在 7 纳米(nm)或存在缺陷的 5 纳米技术节点上。ASML是全球唯一一家生产EUV光刻机的公司,其首席执行官说:"禁止出口EUV,中国将落后西方10到15年。这确实会产生影响"。
By contrast, U.S. chip firms such as Nvidia will in coming years press forward past the 4 nm era into the 2 nm and beyond future, delivering chips that might be thousands or tens of thousands of times better performing integrated into data centers using tens or hundreds of times more chips. While there are still many ways in which the U.S. government could bungle export control implementation and enforcement—which will be discussed later in this paper—the greatest potential for impacts from the export controls are still well ahead in the future as the United States races forward and China’s progress continues to slow. Implemented properly, they offer the opportunity for a meaningful, though far from permanent, edge over China.
- Just as the past six decades of Moore’s Law have driven increased demand for computer chips, DeepSeek and other AI efficiency innovations will continue to do the same for AI chips. There is no ceiling on the demand for intelligence.
Much of the initial commentary on DeepSeek suggested that demand for AI chips might plummet, as efficiency improvements meant that fewer chips were needed to achieve the same level of intelligence. While this is a generally accurate description of efficiency, it is a terrible description of how computing economics works.
As mentioned above, Moore’s Law has driven a more than billionfold improvement in computing efficiency over the past seven decades. Does this mean that all of the world’s computing needs are handled by a single computer that costs only a fraction of a penny? Of course not. Instead, the increased efficiency has made applying digital computation throughout the economy far more attractive, and so more governments, businesses, and consumers have invested in more computation across countless applications and use cases. This is the essence of Jevon’s Paradox, which describes how increasing efficiency can increase aggregate demand.
The same is obviously going to be true of increased AI computational efficiency. Indeed, after the DeepSeek revelations, the largest data center buyers in the world—Microsoft, Google, Meta, and Amazon—announced that they were going to spend hundreds of billions of dollars in 2025 on AI chips and data centers, up by nearly 50 percent over the already record amount invested in 2024. Chinese firms such as Alibaba have announced that they too will make massive chip purchases in the wake of DeepSeek.
Nor is this merely a long-term phenomenon. Even in the short term, rental prices for H100 chips hosted by Amazon’s cloud computing spiked upward in the wake of DeepSeek’s publication as customers worked to incorporate DeepSeek models into their operations. More recently, OpenAI CEO Sam Altman said that OpenAI would have to temporarily limit usage of its GPT 4.5 model because the company did not have sufficient GPUs to meet all of the demand.
Moreover, there is almost certainly no “ceiling” for a desirable amount of intelligence and the computing required to power that intelligence. Miles Brundage, who previously led policy research at OpenAI, described it this way:
To make a human-AI analogy, consider [Albert] Einstein or John von Neumann as the smartest possible person you could fit in a human brain. You would still want more of them. You’d want more copies. That’s basically what inference compute or test-time compute is—copying the smart thing. It’s better to have an hour of Einstein’s time than a minute, and I don’t see why that wouldn’t be true for AI.
This again relates to how the most significant impacts of the export controls are likely in the future, as the evermore extremely scaled deployment of evermore powerful chips begins to bear fruit. It is the next few rounds of increasing AI computation—by tenfold, a hundredfold, a thousandfold—where China will be most severely blocked and the impact of the export controls will be most severely felt.
Some Chinese AI researchers have made similar points. On February 13, 2025, Li Guojie, a scholar at the Chinese Academy of Engineering, told Chinese media outlet ChinaFund that DeepSeek’s success represents a step-change in Chinese AI capabilities but said that “due to the blockade of the U.S. government, China is currently unable to obtain the most advanced chip process technology.” Li went on to say [machine translation]:
The success of DeepSeek does not deny the key role of computing power in the development of artificial intelligence. In fact, since there are much more equipment for reasoning than for training, the computing power required for reasoning [aka inference] will become the main requirement in the future. It is very important to improve the efficiency of the model through algorithm optimization. It is our basic choice to take the green development path of saving computing power, but computing power is definitely a necessary condition for solving artificial intelligence problems and cannot be ignored.
- DeepSeek’s success in distilling U.S. AI models and replicating closed source algorithmic innovations does raise strategic questions about the nature of competitive advantage in AI in the absence of strong intellectual property protections. A worst-case scenario would be if AI is structurally similar to pharmaceuticals.
On January 29, OpenAI told the New York Times that
We know that groups in the P.R.C. are actively working to use methods, including what’s known as distillation, to replicate advanced U.S. A.I. models. . . . We are aware of and reviewing indications that DeepSeek may have inappropriately distilled our models.
Distillation is a well-known technique that allows “the transfer of knowledge from a larger, more complex language model (the ‘teacher’) to a smaller, more efficient version (the ‘student’).” In short, the inputs and outputs from the teacher model are used as very high-quality synthetic training data by the student model. Distillation is utilized by every major U.S. AI lab to offer smaller, more efficient versions of its models that are faster and cheaper to serve to customers. For example, GPT-4o-mini is the distilled version of GPT-4o, and both were made by OpenAI. There are multiple types of distillation, however, and the more advanced types, which AI labs use to distill their own models, require access to the internals of the models.
While a firm distilling its own models is clearly harmless, there is a strategic consideration if one firm can distill another firm’s models, incurring most or all of the benefits of acquiring a highly capable AI model while incurring far fewer of the costs of creating such a model.
In the case of OpenAI and DeepSeek, the distillation evidence appears quite strong: DeepSeek’s initially released models, when asked by users “what model are you?,” will often respond, “I’m ChatGPT.” DeepSeek’s updated versions of the model, likely modified using fine-tuning techniques, no longer produce such responses, but this does not reduce the strength of the evidence that DeepSeek relied on distillation. Such unauthorized distillation would be a violation of OpenAI’s terms of service, but the law firm Fenwick has written that “whether remedies against such [a Terms of Service] breach would sufficiently protect teacher-model owner’s IP remains to be determined.”
If OpenAI were to file a lawsuit against DeepSeek, for example, perhaps it would win in U.S. courts. But how would the size of judicial penalties compare to the strategic benefit for DeepSeek as a firm or for China as a country? And the access to judicial remedies is almost certainly limited to the United States and other countries with strong intellectual property protections. Even if DeepSeek were hypothetically clearly guilty of not only distillation but an even more extreme case of outright corporate espionage, Chinese courts would likely find OpenAI guilty of stealing IP from DeepSeek rather than the reverse.
This is what happened to U.S. chipmaker Micron when it accused China’s Fujian Jinhua of stealing memory chip trade secrets and when U.S. prosecutors charged Fujian Jinhua with economic espionage. In retaliation, Jinhua sued Micron in a Chinese court for patent infringement in early 2018. The Fuzhou Intermediate People’s Court in Fujian province sided with the Chinese side and issued an injunction blocking Micron from selling 26 chip products in China.
Similarly, when U.S. semiconductor equipment firm Veeco found that a Chinese competitor, Advanced Micro-Fabrication Equipment (AMEC), was using Veeco’s proprietary designs, Veeco secured a preliminary injunction in a U.S. court to stop a supplier from providing parts to AMEC. However, in response, AMEC filed a patent suit in China, and the Fujian High Court (the same province as above) acted with remarkable speed. On December 7, 2017—just weeks after AMEC’s filing—the court granted AMEC a preliminary injunction without even hearing Veeco’s defense.
Thus, one should separate the questions of what is “fair” or “true” from what the strategic consequences are likely to be. Neither geopolitics nor business is a contest where first prize always goes to the most innovative. Many companies, including U.S. tech companies, have a perfectly viable business strategy of closely monitoring their innovative competitors and then either buying the competitor or replicating its innovation. Chinese firms can do the same, including in ways that both do and do not violate intellectual property.
Thus, the meaningful strategic question for OpenAI and other U.S. AI firms is not whether DeepSeek is failing to play by the rules, it is whether U.S. firms have a mechanism for effectively preventing DeepSeek or any competitor from extracting the benefits of an AI lab’s investments without bearing the costs. What enduring sources of competitive advantages could the large U.S. AI labs secure if Chinese companies or other copycats (potentially even U.S. ones) can simply piggyback off their work to build cheaper and equivalently good models while incurring only a fraction of the costs?
One possible answer comes in the form of Meta’s strategy, which is to invest in developing open-source AI models justified not by the direct benefits of increasing monthly subscriptions, but by the indirect benefits of enhancing their existing social media and advertising business lines.
Among the worst outcomes for U.S. AI companies would be if the fundamentals of AI research and development were structurally similar to those of the global pharmaceutical industry. In 2023, the top 20 global pharmaceutical companies invested approximately $2.4 billion over 10 to 15 years into developing a typical new drug from start to finish. The costs of research and development dwarf those of drug manufacturing, marketing, and distribution, so the final price of the product must reflect the costs of research and development for pharmaceutical firms to have any hope of recovering their investments and earning a profit.
To incentivize drug R&D, the U.S. government offers companies 20-year patents that allow them the exclusive right to sell their products in the United States at a monopoly premium to recover upfront R&D costs. However, once a patent expires, so-called generic manufacturers can reproduce and sell the same drug for a far lower price—avoiding the burden of R&D costs altogether. Generic manufacturers can thereafter compete by selling identical drugs while incurring only a tiny fraction of the costs faced by the original drug developer. In a world where there was no patent protection on new pharmaceuticals, investment in new drug discovery (and thus the pace of innovative new drugs) would be radically limited because governments and philanthropic actors might be the only ones willing to invest, and they would almost certainly do so at far lower levels than the current private sector does.
Government and business-makers alike must now ask the question: What if frontier AI model research is structurally identical to that of the pharmaceutical industry, meaning extremely high upfront R&D costs and very low replication costs? The implications for AI investment and for U.S.-China AI competition could be significant.
The U.S.-China AI race is very likely not one in which gentlemanly concepts of “fairness” matter. Chinese companies have systematicallystolen American IP for years and will almost certainly continue to do so. Beyond Chinese firms, the Chinese government has a very clear history of either not enforcing or outright supporting Chinese firms that illegally violate U.S. patents in cases where the Chinese government has identified the industry as strategically significant. AI certainly falls into that category.
Kai-Fu Lee, arguably the top venture capitalist in China’s AI sector, wrote in his 2018 book AI Superpowers that imitation does not have the same stigma in China that it does in the United States. He went further to argue that China’s unabashed willingness to copy innovation and even ignore intellectual property makes its companies more competitive, not less. In describing the post-2010 rise of hypercompetitive and cutthroat Chinese tech firms as “copycat gladiators,” Lee’s writing on this topic is worth quoting at length:
Silicon Valley may have found the copying undignified and the tactics unsavory. In many cases, it was. But it was precisely this widespread cloning—the onslaught of thousands of mimicking competitors—that forced companies to innovate. Survival in the internet coliseum required relentlessly iterating products, controlling costs, executing flawlessly, generating positive PR [public relations], raising money at exaggerated valuations, and seeking ways to build a robust business ‘moat’ to keep the copycats out. Pure copycats never made for great companies, and they couldn’t survive inside this coliseum. But the trial-by-fire competitive landscape created when one is surrounded by ruthless copycats had the result of forging a generation of the most tenacious entrepreneurs on earth. As we enter the age of AI implementation, this cutthroat entrepreneurial environment will be one of China’s core assets in building a machine-learning-driven economy.
Taking Lee’s argument one step further: If the future global competitive landscape for AI is one of ruthless copying in the absence of robust intellectual property protections, that is an environment where Chinese entrepreneurs ought to do extremely well, because it resembles the domestic Chinese tech ecosystem in which they grew up and thrived.
- Unfortunately, developments in recent years do not give confidence that traditional approaches to protecting intellectual property and technology secrets are likely to be effective when it comes to China and AI.
Even over just the past three years, a diverse set of examples suggests that robustly protecting key knowledge in the AI field is likely to be extremely difficult:
- In February 2025, a federal grand jury charged a Chinese national and former Google employee with “seven counts of economic espionage and seven counts of theft of trade secrets in connection with an alleged plan to steal from Google LLC (Google) proprietary information related to AI technology.”
- In February 2023, ASML, the most important provider of the lithography equipment used to manufacture advanced chips, accused a former employee in China of stealing proprietary technology data in an attempt to circumvent export controls.
- In January 2025, Eindhoven University of Technology, a school only five miles away from ASML’s headquarters and with close ties to the company, had to suspend all activities as it worked to analyze the impact of a Chinese cyberattack.
- In 2022, WIRED reported that a group of cyber criminals breached Nvidia and stole “a significant amount of sensitive information about the designs of Nvidia graphics cards, source code for an Nvidia AI rendering system called DLSS, and the usernames and passwords of more than 71,000 Nvidia employees.” Nvidia claims to have since significantly upgraded its cybersecurity, and this particular attack does not seem to have originated in China. However, it is concerning that a hacking group got so close to the technology crown jewels of such an important U.S. AI company.
- In 2023, Chinese intelligence services successfully hacked the email accounts of then-Secretary of Commerce Gina Raimondo and other U.S. officials, apparently looking for intelligence related to U.S. export controls.
- In 2025, The Information reported that DeepSeek CEO Liang had visited San Francisco in mid-2024 and “met with researchers he knows, including some OpenAI employees, to stay up to date.” This is consistent with Center for AI Safety Director Dan Hendrycks’ claim that
If you know which research dead ends to avoid (e.g., MCTS) and roughly know what research direction to pursue, replication is much easier. These algorithmic insights likely slowly leaked through SF party conversations and other channels. It probably takes a few months once you have the idea for [OpenAI’s reasoning AI model] o1 to replicate it. That’s how DeepSeek did it without much compute.
While there is ongoing research into techniques to prevent model distillation, and AI companies will no doubt increase these efforts in the wake of DeepSeek, this remains an open question with significant implications. In an interview published in ChinaTalk, Anthropic CEO Dario Amodei said,
Like all problems in cybersecurity . . . [distillation is] going to be an ongoing issue. That’s why some companies have chosen to kind of hide the chain of thought in their reasoning models, because it makes it much harder to distill. Those can be jailbroken. But folks are working on antidotes to jailbreaking. We just released something today that makes it much harder to jailbreak models.
Even in a case where distillation and more explicit cases of technological espionage are difficult or impossible to prevent, however, there will remain the issue inference computation, which involves applying more computational resources so that the model can “think” harder about the answer to a question or so that the model can be used more times and serve more users. One way of thinking about this is that the nature of competitive advantage in AI could be shifting from a secret that one keeps (i.e., the model weights) toward an asset that one controls (i.e., massive data center compute infrastructure). This means that export controls—if implemented and enforced effectively—still have an opportunity to have a strategic impact.
Nevertheless, the U.S. government and U.S. AI firms need to take a tough look at whether and how they can remain competitive in a world where China does not respect intellectual property and is committed to devoting enormous state resources to technical espionage in the AI and semiconductor sectors.
- The effectiveness of export controls will depend upon effective implementation and enforcement to prevent chip smuggling. There is cause for significant concern on this point if the Trump administration reduces relevant government resources and staff or causes allied countries to not cooperate.
The Bureau of Industry and Security (BIS) at the U.S. Department of Commerce is the agency charged with adjudicating license applications and enforcing export controls, not just on semiconductors bound for China, but for all U.S. dual-use technology exports that might end up in Russia, Iran, North Korea, and many other restricted destinations. To implement its work overseeing trillions of dollars in economic activity and policing smuggling operations worldwide, BIS has fewer than 600 employees and a relatively paltry budget of just under $200 million. Semiconductors are just one technology category out of hundreds that this organization is responsible for enforcing.
Reporting by The Information found at least eight Chinese AI chip–smuggling networks, with each engaging in transactions valued at more than $100 million. More recent reporting by the Wall Street Journal suggests that this smuggling now includes the latest generation of Nvidia Blackwell AI chips. China is betting that its network of smugglers and shell companies can find the leaks in the BIS export control enforcement barrier. As long as Congress continues to neglect BIS by providing grossly inadequate resources compared to the size and importance of its mission, China has a reasonable expectation of success. BIS needs not only more money, but also more skilled staff, more enforcement agents, and better enabling technology, especially in data analysis.
Moreover, the Department of Commerce needs more help from the rest of the government, in particular the U.S. intelligence community. Declassified Central Intelligence Agency documents show that the intelligence community was deeply involved in assisting export control enforcement during the Cold War and delivered solid results by doing so. These are capabilities and priorities that have significantly atrophied in the post–Cold War era but urgently need to be restored.
Recent efforts by the Trump administration to reduce government spending risk making a bad situation worse. On February 5, 2025, U.S. Attorney General Pam Bondi signed a Department of Justice memorandum that, among many other actions, disbanded the National Security Division’s Corporate Enforcement Unit, which was “created with the goal of investigating and prosecuting corporate actors involved in sanctions evasion, export control violations, and other national security-related economic crimes.” The memo did not provide any explanation for how the duties of the unit would be performed elsewhere in the Department of Justice, which could mean that this will be implemented as a straightforward reduction in U.S. government capacity to enforce export controls.
Of all the choices available, the worst policy choice that the U.S. government could make is to regulate aggressively but with large loopholes and weak enforcement. Such an approach—which fairly describes the approach taken in the first Trump administration and large chunks of the Biden administration—incurs nearly all the costs of an aggressive export control policy but does so without providing any of the possible strategic benefits in terms of slowing Chinese progress in semiconductors and AI.
- Beyond smuggling, the greatest strategic challenge for the United States is the potential for China to produce AI chips domestically at sufficient quantity and quality to build AI data center infrastructure that is competitive with the United States.
As described above, the nature of AI geopolitical advantage appears to be moving away from software secrets that are difficult to protect and toward hardware chip and data center assets that are (hopefully) easier to protect. In this sense, DeepSeek is not in and of itself the most significant threat to U.S. leadership in AI. Instead, the greater challenge arises from the possibility of China having a domestic ecosystem for producing its own AI chips at large scale and integrating them into Chinese data center training, as well as running inference for DeepSeek and other AI models.
As U.S. technology firms are planning hundreds of billions of dollars in AI data center infrastructure investments, it is worth remembering that—for those investments to be possible—companies like TSMC must manufacture enough AI chips to fill those data centers. In the case of companies such as Nvidia, their revenue growth in recent years is less than it would have otherwise been due to shortages of TSMC production capacity.
The Biden administration took many steps designed to definitively cut China’s AI chip designers off from TSMC production capacity. Most recently, on January 15, the Commerce Department announced the final tranche of Biden administration export controls, often referred to as “the Foundry Rule.” The Foundry Rule moved advanced chip production to a white-list system that will likely make it impossible for Chinese AI firms to access TSMC capacity to produce chips above export control performance thresholds even when operating through complex shell company arrangements. However, as discussed below, TSMC manufactured a strategically significant quantity of chips on behalf of Huawei via shell companies prior to the rule going into effect.
That effectively means that China’s long-term future in AI is closely tied to its ability to produce AI chips domestically. The Biden administration sought to hamstring China’s domestic production of advanced chips by restricting the sale of advanced semiconductor manufacturing equipment, including from other countries.
- DeepSeek stands out among Chinese AI model developers because of its demonstrated ability to make architectural innovations below the level of Nvidia’s CUDA software ecosystem. If DeepSeek were devoted to strengthening Huawei’s Ascend chips and CANN software ecosystem, it would pose a much more significant threat to Nvidia.
Historically, one of the biggest sources of competitive advantage for Nvidia has been its CUDA (Compute Unified Device Architecture) software ecosystem. CUDA makes it much easier for programmers to write massively parallelized software (as all modern AI software is) and ensures backward and forward compatibility so that older chips can still run newer software and vice versa. Any customer who seeks to stop using Nvidia chips has to leave the CUDA ecosystem, which requires solving a lot of incredibly hard software problems for which CUDA already provides free answers. Those free answers reflect billions of dollars of investment in the CUDA platform by both Nvidia and its customers.
DeepSeek stands out among AI model developers because it implemented software changes below the CUDA level. Most AI researchers rely on CUDA, a higher-level programming language for NVIDIA GPUs, because it simplifies the development process. However, CUDA does not allow fine-grained control over GPU operations at the level DeepSeek required. DeepSeek modified software instructions written in a language called PTX (Parallel Thread Execution). By programming directly in PTX, DeepSeek engineers were able to optimize how the GPU handled specific workloads, particularly in managing expert specialization in the Mixture-of-Experts model and improving memory efficiency. This is an “assembly-level” approach to GPU programming—one that is both extremely difficult and (at least historically) rarely attempted. The trade-off, however, was a major increase in performance efficiency, particularly for large-scale AI training tasks.
This suggests that DeepSeek may have both the technical knowledge and the open-source community enthusiasm to finally start generating momentum around Huawei’s competitor to CUDA, which Huawei refers to as its Compute Architecture for Neural Networks (CANN). The greater maturity of the CUDA software ecosystem currently makes Nvidia chips more attractive, but this could change over the next few years. If it does, it would have major implications for Nvidia’s competitiveness both inside and outside of China.
At present, the combination of Ascend chips (discussed more below) and CANN software appears far from being competitive to CUDA. A Financial Times report from September 2024 found that even Huawei employees found the product “difficult and unstable to use” and prone to crashing frequently. Industry sources told CSIS that DeepSeek’s more recent evaluation of CANN was very negative and that DeepSeek assessed that it would be years before the combination of Ascend chips and CANN-compatible software was a viable alternative.
Still, this could change with time, effort, and investment. Huawei recently joined the open-source PyTorch foundation in an effort to increase Ascend and CANN compatibility of the PyTorch AI development framework.
There is at least one meaningful precedent regarding the difficulty of leaving the Nvidia CUDA ecosystem, which is Google’s shift from TensorFlow on Nvidia GPUs to JAX on their proprietary chips, called TPUs. In this case, it took several years for JAX to mature and for a critical mass of software libraries to appear—even with heavy Google investment and the payoff opportunity in terms of both efficiency and competitive differentiation. Even by 2023, Google was still heavily investing to improve JAX (for example, addressing pain points like multi-host setup and memory efficiency) and encouraging its use through education (tutorials, conference workshops). For a company like DeepSeek, migrating all AI workloads from CUDA to CANN would likely be a multiyear project. Google’s internal shift took 2–3 years to bear significant fruit, and even then Google maintained support for both for a long while.
- China’s alliance of Huawei (AI chip designer), SMIC (AI chip manufacturer), and CXMT/XMC (high-bandwidth memory manufacturers) have recently made strategically significant progress in advancing domestic production of AI chips.
Domestically producing large quantities of AI chips will require China to domestically replicate multiple segments of the AI chip value chain. The most important links are AI chip design, advanced node logic chip manufacturing, and advanced node high-bandwidth memory (HBM) manufacturing. Each of these will be addressed in turn.
- Huawei’s AI Chip design has long been strong. Before the 2020 export controls, it was poised to meaningfully challenge Nvidia in China with TSMC manufacturing and Chinese government pressure to purchase Huawei products over Nvidia ones.
Like the United States, China has many different companies working on AI chip design, including Huawei, Cambricon, Biren, and more. However, Huawei is unambiguously in the strongest position with its Ascend AI chip product line. Industry sources also told CSIS that Huawei—due to its highly influential position with the Chinese government and its size as a customer—has the greatest leverage over SMIC. In other words, even if Huawei were not the best AI chip designer in China, it would still be the most important since it is in a position to restrict the share of SMIC manufacturing capacity that goes toward its domestic competitors.
Huawei has been a player in the AI chip market for longer than is generally realized. Huawei had a 7 nm AI Accelerator chip planned for production at TSMC in 2020. At the time, Huawei was preparing to capitalize the so-called 3-5-2 policy, in which the CCP’s Central Office ordered all government agencies and many state-owned enterprises to eliminate the use of all non-Chinese technology within three years. However, Huawei was unable to fulfill demand because of the first Trump administration’s updated 2020 entity listing, which temporarily cut Huawei’s Ascend and Kirin lines off from TSMC. In this instance, Nvidia was the beneficiary of these early U.S. export controls, which delivered a setback to Chinese efforts to eliminate dependence on U.S. AI chip technology.
Today, Huawei’s Ascend product line is focused on the Ascend 910B and the Ascend 910C, the latter of which includes two Ascend 910B logic dies per integrated unit (meaning more real estate on each silicon wafer is required for manufacturing an Ascend 910C than a 910B). DeepSeek has reportedly evaluated the Ascend chips and found that they are unattractive for training AI models but that each Ascend 910C delivers roughly 60 percent of the performance of an Nvidia H100 for inferencing AI models. This matters greatly since more and more of the computing requirements for advanced AI models are expected to be devoted to inference in the coming years. Barclays, an investment bank, estimates that by 2026, 70 percent of AI compute demand will come from inference. In February 2024, Nvidia CEO Jensen Huang estimated that utilization of its chips was “40 percent inference, 60 percent training,” compared to 90 percent training-focused in the 2016 timeframe.
- Sources told CSIS that Huawei’s use of shell companies to gain access to TSMC manufacturing capacity allowed them to acquire more than 2 million AI chip dies and that Huawei has also stockpiled more than a year’s worth of HBM.
Chinese firms like Huawei have historically had two major options for manufacturing their AI chip designs: outsource production abroad to TSMC of Taiwan or produce chips domestically in partnership with SMIC.
For a time, it seemed as though the 2020 entity listing of Huawei definitively cut the company off from accessing TSMC advanced node manufacturing capacity. The October 2022 and October 2023 export controls were supposed to have done the same for all advanced node AI chip designers in China.
However, TSMC manufactured large quantities of Huawei Ascend 910B chips on behalf of Huawei shell companies and shipped the chips to China in violation of U.S. export controls. In an interview with the New York Times, an unnamed Taiwanese official acknowledged that these chips “ended up in the hands of Huawei.” Government officials told CSIS that TSMC manufactured more than 2 million Ascend 910B logic dies and that all of these are now with Huawei. If true, this is enough dies to make 1 million Ascend 910C units. However, the advanced packaging process by which two Ascend 910B dies and HBM are combined into a unified Ascend 910C chip also introduces defects that can compromise the functionality of the chip. Industry sources told CSIS that roughly 75 percent of the Ascend 910Cs currently survive the advanced packaging process.
This is a strategically significant stockpile of AI chips. For comparison, Nvidia reportedly shipped 1 million H20 chips (which are inference specialized and targeted at the Chinese market) to China in 2024. Nvidia’s January 26 SEC filing states that it generated $17 billion in revenues from Chinese customers in the most recently ended fiscal year (which would obviously exclude revenues from chips later smuggled to China). Presumably, the vast majority of these revenues are from H20 sales.
Even though Huawei likely has the more than 2 million Ascend 910B logic dies made by TSMC, there is a question as to whether it has enough HBM to integrate with those dies in manufacturing 1 million Ascend 910Cs. It seems likely that Huawei does, however, since the U.S. plan to restrict all advanced HBM sales to China on a country-wide basis was leaked to Bloomberg in August 2024 and did not go into effect until December of that year, giving Huawei ample time to legally acquire HBM chips as part of a stockpiling strategy. Industry sources told CSIS that Huawei has stockpiled enough HBM to meet their internal expectations for at least a full year’s worth of production, mostly in the form of purchases from Samsung of South Korea, potentially via shell companies, that occurred before the December controls went into effect.
It is as though the U.S. government was having an internal debate about whether or not to have a surprise attack, and in the spirit of compromise, the slow interagency process settled on attacking without the surprise. This was an egregious failure with strategic consequences.
With the adoption of the Foundry Rule, it will hopefully be impossible for Huawei or other Chinese AI chip designers to ever again access TSMC manufacturing capacity, even when using sophisticated shell company tactics. Huawei will instead be restricted to what Chinese domestic logic chip manufacturers can produce.
However, there is also the potential risk that an existing chip design company on the Foundry Rule white list could be tempted by massive revenue opportunities to act as a pass-through to give Huawei access to TSMC. Guarding against this risk will require making clear to TSMC that the negative consequences of continuing to supply Huawei with advanced AI chips would be colossal compared to the revenue and profit opportunity. Similarly, Huawei may attempt similar tactics with other chip foundries such as Samsung or even Intel.
- Huawei’s AI chip manufacturing partner, SMIC, has been struggling with low production yield (~20 percent) and 20,000 7 nm monthly wafer production due to U.S. and allied export controls. SMIC has a difficult and uncertain path toward producing at nodes more advanced than 7 nm.
The most advanced logic chip manufacturer in China is SMIC. SMIC’s SN2 facility in Shanghai is the sole facility in China with an active 7 nm logic chip production line and has been producing 7 nm chips since July 2021, more than a year before the first tranche of the Biden administration’s semiconductor equipment export controls went into effect. SMIC and Huawei are now working to bring a 5 nm node into scaled production but must do so without access to EUV lithography equipment, since China has no local producer of EUV lithography machines and since export controls have prevented such machines from ever being exported to China. Industry sources told CSIS that, in early 2020, ASML was poised to ship EUV tools to China and that SMIC was planning to work with key research labs in Europe, such as the Interuniversity Microelectronics Centre (IMEC), to help develop their EUV-based manufacturing process.
In December 2024, industry sources told CSIS that SMIC currently has enough immersion deep ultraviolet (DUV) lithography equipment supplied by the Dutch company ASML to produce 85,000 FinFET wafers per month (WPM) across both SN1 (which focuses on 14 nm node production) and SN2 (which focuses on 7 nm and 5 nm production). This acquisition of lithography tools reportedly took effect before Dutch DUV lithography export controls went into effect in mid-2023.
However, the bottleneck in expanding 7 nm (which in SMIC’s node naming system is called “N+2”) production capacity has not been lithography but rather U.S. tools for etching, deposition, inspection, and metrology. The shortage of inspection and metrology tools has also been an important factor preventing SMIC from improving its yield. SMIC acquired lithography equipment as a stockpiling measure in anticipation of future export controls by the Dutch government.
Low production rate also has an impact on yield, since manufacturing technicians and engineers tend to improve their techniques based on experimentation and observed results from production. Thus, more production is helpful in accelerating the pace of learning and thus improving the yield.
Based on the Ascend 910B die size of 665.61 mm², CSIS estimates that each 300-millimeter diameter silicon wafer produces roughly 80 chip dies. This is simply counting the number of small rectangles (chip dies) that can fit inside the large circle (the wafer). A source told CSIS that of these 80 chip dies:
- Roughly 20 percent (~16) are fully functional with either no defects or no defects that negatively impact performance. This is the same as saying that SMIC has 20 percent yield when making these chips.
- An unknown share of chips are functional with degraded performance, though customer demand for such degraded chips (in Huawei’s case, though not Nvidia’s) is low.
- An unknown share of chips are completely nonfunctional.
SMIC’s 20 percent yield when producing Ascend 910Bs is quite low, but it is not as low as it might seem. In 2020, when Nvidia moved its GPU production to TSMC’s 7 nm process, the defect-free yield rate was roughly 41.5 percent. Since manufacturing defects occur randomly on a defects per square centimeter of chip area basis, larger chips (such as AI chips) are more susceptible than smaller chips (such as smartphone application processors) to a high defect rate. Using TSMC during roughly the same time period and with roughly the same defect rate, Apple’s A12 processor enjoyed 90 percent+ yield. SMIC also makes the application processor for Huawei’s Mate smartphone line, and its yield when making these smaller chips is somewhere in the 50–70 percent range.
However, a February 24, 2025 report by the Financial Times, citing two anonymous individuals, claims that SMIC’s AI chip yield has increased to roughly 40 percent. Industry sources told CSIS that the Financial Times report is not correct and that Huawei/SMIC’s true yield remains at 20 percent. One explanation for the discrepancy would be if the Financial Times’ sources were mistakenly including both the fully functional chips and the chips that are functional but with degraded performance when estimating yield.
- Sources told CSIS that Huawei, SMIC’s most important customer, has successfully managed to move stockpiled U.S.-built equipment from SiEn (芯恩), Pensun (鹏新旭), and Huawei’s fab in Dongguan to SMIC SN2.
As mentioned above, SMIC’s shortage of deposition, etching, inspection, and metrology semiconductor manufacturing equipment also been an important factor preventing expanded production capacity of its SN2 facility. Some of this equipment is restricted on a country-wide basis, meaning that it cannot be legally sold anywhere in China. However, other types of this equipment were restricted only on an end-use and end-user basis. This means that the equipment can be sold to some customers in China but not others. In some cases, this even means that it can be sold to some facilities of a particular customer, but not others. In such cases, relocating the equipment from one facility to another would require a new export license in order to be legal. But SMIC’s production of 7 nm chips using U.S. equipment is already illegal, and both SMIC and other Chinese firms always have the option to choose illegal activity, particularly since such activity frequently enjoys the active support of the Chinese government.
Industry sources told CSIS that SiEn, Pensun, and Huawei’s fab in Dongguan all were able to legally acquire the needed etching, deposition, and inspection/metrology equipment that SMIC needs for two reasons: (1) the equipment was not restricted on a country-wide basis to all of China and (2) the equipment was restricted on an end-use and end-user basis, but SiEn and Pensun told U.S. firms that it would exclusively be used for producing chips less advanced than 14 nm. These firms also denied any affiliation with Huawei. Government officials told CSIS that in such circumstances, the equipment can often be sold under a no-license required status.
According to the sources, SiEn and Pensun, however, did not have sufficient customers providing demand for using all of the equipment they had purchased, and so some of it was never used operationally in their fabs. They purchased the equipment as a stockpiling move in anticipation of future export controls. The source described this as a “buy everything you can, while you can” strategy.
The SMIC SN2 facility needed the equipment. Since SiEn and Pensun were not making economically productive use of the equipment, they were amenable to a sale. This sale was negotiated in Q4 of 2024 and completed in Q1 of 2025. The sources are under the impression that all of the desired equipment is currently either installed at SMIC SN2 or on-site awaiting installation.
- As a result of the successful in-country equipment transfer, SMIC expects to achieve 50,000 7 nm WPM by the end of 2025. If all of this capacity was devoted to manufacturing Ascend AI chips (which is unlikely), that would imply the production of millions of Ascend 910C chips annually.
This equipment will eliminate the near-term U.S. equipment bottleneck facing SMIC for SN2 7 nm production. SMIC’s new 7 nm production bottleneck is the overall size of the facility. Prior public statements from SMIC indicated that the SN1 and SN2 facilities combined have a maximum capacity of 85,000 WPM and that SMIC intended to split production evenly between the two facilities, implying SMIC was targeting ~42,500 WPM of lithography capacity at SN2. Sources told CSIS that SMIC was targeting 50,000 WPM of 7 nm specifically by the end of 2025. It is unclear whether this means that 7 nm production will now also take place at SN1 or whether SMIC has figured out a production process and equipment configuration that allows them to squeeze more production capacity than expected into SN2. If, hypothetically, SMIC were to devote all 50,000 WPM to Ascend 910C manufacturing, this would imply producing 4 million Ascend 910B dies per month, of which 800,000 would be fully functional. 800,000 910B Ascend dies is enough to manufacture 400,000 910C chips, though the advanced packaging process would reduce yield further, and it is unclear how much HBM Huawei has on hand.
SMIC is unlikely to devote all of its 7 nm capacity to Ascend chips. Huawei needs that 7 nm capacity for its chips for smartphones, laptops, data centers, and telecommunications equipment. Moreover, SMIC has other customers besides Huawei. Still, the point remains that Huawei is likely poised to dramatically expand Ascend production in the near future.
Over time, SMIC’s 7 nm yield will likely improve significantly above 20 percent due to the additional equipment and the increased production rate. It will likely not match the best TSMC 7 nm yields for AI chips, however, since TSMC’s best 7 nm yields used EUV, and China has no credible near-term path for acquiring EUV technology.
Over the mid and long term, however, Huawei is working aggressively to produce a domestic alternative to ASML EUV technology. Huawei currently has two semiconductor manufacturing equipment tool research facilities, one in Shanghai and one in Shenzhen. According to a report by Nikkei Asia, Huawei is investing $1.66 billion in the Shanghai facility alone and has hired a large number of chip industry veterans with experience working at companies like Applied Materials, Lam Research, KLA, ASML, TSMC, Intel, and Micron.
Taylor Ogan, a venture capitalist based in Shenzhen, China, focused on investing in Chinese technology companies, described Huawei’s EUV efforts as follows:
There are teams at Huawei in two different parts of China that are working around the clock. . . . They are literally cut off from their friends and family just because the work they are doing is so sensitive, and imagine how quickly they’re going to accelerate Chinese complete domestic chip development. . . . One of them is in Shenzhen. That’s the bigger [EUV lithography] breakthrough lab, and you can look on Google Earth. There was nothing there and now there is something there, and there are thousands of people working there. The other one is around Shanghai and that is more for the next chips in the next phones. So, yeah, the bigger lithography breakthroughs are [being worked on] in Shenzhen. . . . They’re working their asses off. . . . This is like some of the smartest people in the space, and they’re all Chinese.
As mentioned above, these secretive efforts underway at Huawei are extremely likely to be benefitting from state-backed industrial espionage, including the cyberattacks on ASML and its partners.
- Huawei’s Ascend chips continue to face challenges in terms of a lack of compatible AI software that is driving low utilization of purchased chips. However, this could change if DeepSeek’s open-source community enthusiasm improves Huawei’s CANN software ecosystem competitiveness with Nvidia’s CUDA.
A high-quality recent analysis by Nicholas Welch, Lily Ottinger, and Jordan Schneider of ChinaTalk concludes that China’s current compute shortage has more to do with practical challenges and suboptimal deployment—many idle small and medium data centers rather than fewer fully-utilized superclusters—than it does with an absolute shortage of chips. A combination of recent Chinese government policy changes, such as “hand[ing] over their idle computing resources to cloud providers” and strategic moves by China’s largest tech companies to favor DeepSeek models even over their in-house ones, suggests that this could change in the near future.
According to reporting by Caijing Magazine, the chips in China most likely to be underutilized are Huawei’s Ascends, which have benefitted in terms of government pressure to buy domestic but remain underutilized because of a lack of useful AI models that are Ascend-compatible. This could change if DeepSeek adapts its models to run on Ascends, which it is reportedly working toward for model inference, though not model training.
- Between the chip dies acquired from TSMC, the stockpiled HBM, and SMIC’s increasing Ascend production, Huawei and DeepSeek have a credible path to a million-Ascend-chip AI supercluster should they seek to build one, though they will face challenges in large scale chip integration and software frameworks.
As mentioned above, sources told CSIS that TSMC has supplied Huawei with enough chip dies to manufacture a million Ascend 910C chips, each of which has roughly 60 percent the performance of an Nvidia H100 when used for inference. With the help of Huawei and the Chinese government, DeepSeek could plausibly succeed in driving a reallocation of the Nvidia H800s and H100s already in China toward itself for construction of a new AI model training data center. This could then be augmented by an additional, much larger cluster of Huawei Ascend 910Cs (or Nvidia H20s) to be used for AI model inference. Such a combination is a plausible, perhaps even likely, path for China to continue making significant progress in its AI development.
As discussed above, a million-chip cluster of Huawei Ascends would face the challenge of a shortage of AI models, software, and developer tools that are compatible with Huawei’s CANN software ecosystem. A second challenge is in integrating all of the chips as part of a single cluster where the whole is more than the sum of the parts. Financial Times reporter Eleanor Olcott interviewed one Beijing-based chip investor who said “The bottleneck at the moment isn’t getting the chips but figuring out how to make them work in a cluster. This is really complicated work.” Evidently the true attractiveness of the Huawei Ascend 910C chips is worse than the “60 percent of H100” figure might suggest.
- U.S. firms are still ahead of China in the race toward human-level artificial general intelligence and beyond human-level artificial superintelligence. However, the gap has narrowed significantly, and it is unrealistic to expect a lead of more than a year or two, even with extremely aggressive export controls.
As discussed above, in the absence of export controls, it is plausible that Chinese firms would have already surpassed their U.S. competitors in developing and deploying frontier AI models. It is likewise plausible that China might have already succeeded in driving widespread adoption of the Huawei Ascend chips, just as Huawei’s smartphone division, bolstered by internal chip designs, was looking poised to overtake Apple’s iPhone in China prior to the introduction of effective export controls in 2020. The export controls have done much to slow and complicate China’s technological rise and march toward their longstanding objective of technological self-sufficiency. They are still having a significant effect even now and are poised to have even greater impact in the future as U.S. firms benefit from Nvidia’s next-generation Blackwell chips and as China likely remains stuck at the 7 nm technology node until and unless they develop a domestic EUV lithography capability.
However, China’s successes in substantial government investment, chip smuggling, exploiting gaps in U.S. export control coverage, completing in-country transfers of equipment, hiring talent with experience from leading international firms, reverse-engineering foreign technology, harnessing state-backed economic espionage, and producing genuine domestic innovation are a formidable combination. U.S. firms, working at maximum effort and with the benefit of all their own advantages, will still likely be the first to develop artificial general intelligence or artificial superintelligence. This should not, however, be taken for granted.
At a meeting on February 17 between CCP Chairman Xi Jinping and Chinese technology executives, including DeepSeek CEO Liang Wenfeng, Huawei founder Ren Zhengfei told Xi that his previous concerns about the lack of domestic advanced semiconductor production and the damaging impacts of U.S. export controls had eased because of recent breakthroughs by Huawei and its partners. It is possible that he is referring to the aforementioned domestic equipment transfer to SMIC’s facility. Ren further said that he is leading a network of more than 2,000 Chinese companies that are collectively working to ensure that China achieves self-sufficiency of more than 70 percent across the entire semiconductor value chain by 2028. These predictions should be taken seriously and have enormous strategic implications beyond just the AI sector.
However, for AI, the key question remains the one that Dario Amodei, CEO of Anthropic, has succinctly posed: “whether China will also be able to get millions of chips.”
China’s success to date suggests that, at least for Huawei Ascend chips, the answer is that they will have millions of chips within the next year or two. Thankfully, these chips are, at present, dramatically lower performing than Nvidia ones for training advanced AI models; they are also supported by a much weaker software ecosystem with many complex issues that will likely take years to sort out. This is the time that the export controls have bought for the United States to win the race to AGI and then use that victory to try and build more durable strategic advantages. At this point, all the margin for sloppy implementation of export controls or tolerance of large-scale chip smuggling has already been consumed. There is no more time to waste.
Gregory C. Allen is the director of the Wadhwani AI Center at the Center for Strategic and International Studies in Washington, D.C.
This report is made possible through general support to CSIS. No direct sponsorship contributed to this report.
The author would like to thank Georgia Adamson, Joshua Turner, and Ryan Featherston for their research support. The author would also like to thank Richard Danzig, Lennart Heim, William Reinsch, Scott Kennedy, Navin Girishankar, and others who wish to remain anonymous for their helpful feedback on earlier drafts of this report.