What I Learned From Thinking Fast And Slow
我从《快而慢》中学到的东西
Quite possibly the single most important book ever written for data scientists, decision makers, and everyone else.
这可能是有史以来为数据科学家、决策者和其他人撰写的最重要的一本书。
In case you missed it, Nobel Laureate (Economics) Daniel Kahnemann passed away recently. Kahnemann was best known for his research into human decision-making and the various flaws/biases that influence our decision-making processes.
诺贝尔经济学奖获得者丹尼尔-卡内曼(Daniel Kahnemann)最近去世了。卡内曼因其对人类决策以及影响决策过程的各种缺陷/偏见的研究而闻名于世。
His book, “Thinking Fast and Slow”, was perspective-changing for me. I do not use this lightly, and there are only two other books that I would say this about (Don Quixote and The Prince).
他的著作《思考的快与慢》改变了我的观点。这句话我可不是随便说说的,我只对另外两本书(《堂吉诃德》和《王子》)说过这句话。
In this email/post, I want to convince you to give this exceptional book a read. Here are some highlights:
在这封电子邮件/这篇帖子中,我想说服你读一读这本杰出的书。以下是一些要点:
A Summary of the Book: Thinking Fast and Slow is about people and our decision making. It states that our minds operate using two distinct systems:
《本书摘要:思考快与慢》是一本关于人和决策的书。书中指出,我们的大脑使用两种不同的系统进行运作:
- System 1: Fast, automatic, intuitive, and emotional. This is the default system our brain runs on.
系统 1:快速、自动、直观、感性。这是我们大脑运行的默认系统。 - System 2: Slow, effortful, logical, and deliberate. We have to intentionally activate this system. It takes a lot of effort, so we can’t use this as a default. Furthermore, System 1 can often skew the analysis done by System 2 (for eg. take confirmation bias: our analysis (S2) will often build a narrative from cherry-picked examples that suit our original conceptions/gut feelings (S1) ). This is why being mindful of our biases is key to making better decisions- this helps us counteract some of the underlying pressure that System 1 exerts to skew our decisions.
系统 2:缓慢、努力、合乎逻辑、深思熟虑。我们必须有意激活这一系统。这需要很大的努力,所以我们不能将其作为默认设置。此外,系统 1 经常会歪曲系统 2 所做的分析(例如,确认偏差:我们的分析(S2)经常会根据我们最初的概念/直觉(S1),从挑选出来的例子中建立一个叙述)。这就是为什么意识到我们的偏见是做出更好决策的关键--这可以帮助我们抵消系统 1 所施加的一些潜在压力,从而使我们的决策出现偏差。
The book covers (in a lot of depth) how this split impacts decision-making and the various cognitive biases that arise out of our architectural design.
这本书(非常深入地)介绍了这种分裂如何影响决策,以及我们的建筑设计所产生的各种认知偏差。
Why you should read it: We live in a world fueled by rapid information, and instantaneous decisions. The sensory overload heightens the weaknesses of our System 1, and lulls us into automatic judgments and a lack of critical thinking. Thinking, Fast and Slow is an antidote. Studying the biases helped me step back, question our automatic responses, and make better choices (much of my writing has arisen from me stepping back and questioning whether the dominant narrative made sense to me). The book is also very very humbling.
为什么要读这本书我们生活在一个信息飞速发展、决策瞬息万变的世界。超负荷的感官刺激加剧了我们 "系统1 "的弱点,使我们陷入自动判断,缺乏批判性思维。思考,快与慢》就是一剂解药。对偏见的研究帮助我后退一步,质疑我们的自动反应,并做出更好的选择(我的许多写作都源于我后退一步,质疑主流叙事对我是否有意义)。这本书也非常非常令人谦卑。
How to Read Thinking Fast and Slow: The book gets very deep into the experiments and their various implications. This makes it a drag to read, and it’s not a book made for reading over a day/weekend (unless you’re very interested in the experiment design itself).
如何阅读《思考快与慢》:这本书非常深入地探讨了实验及其各种影响。这让它读起来很费劲,而且这本书不适合在一天/周末内读完(除非你对实验设计本身非常感兴趣)。
Instead, I’d recommend reading TFAS over months, where you come back to the book regularly to study the various biases and the experiments.
相反,我建议您用几个月的时间来阅读《TFAS》,定期回过头来研究书中的各种偏见和实验。
I also had a lot of fun critiquing the setup of the experiments themselves, poking at the limitations, and thinking about how I would redesign them.
我还对实验本身的设置进行了点评,指出了实验的局限性,并思考了如何重新设计实验,从中获得了很多乐趣。
The biggest benefit I got from the book was when I started looking into how various PR communications/marketing messages online try to exploit these biases to convince us to go with their messages.
这本书给我带来的最大益处是,我开始研究网上的各种公关传播/营销信息是如何试图利用这些偏见来说服我们接受他们的信息的。
Without this book, I’m sure I would have been a lot more vulnerable to getting caught up in the hype-based repetitive falsehoods that we often discuss in our cult.
如果没有这本书,我肯定会更容易陷入我们在邪教中经常讨论的炒作式的重复谬误中。
I want to spend the rest of this write-up talking about some of the most important realizations that Thiking Fast and Slow has helped me reach (both directly and indirectly).
在这篇文章的其余部分,我想谈谈《快与慢》帮我实现的一些最重要的认识(包括直接和间接的)。
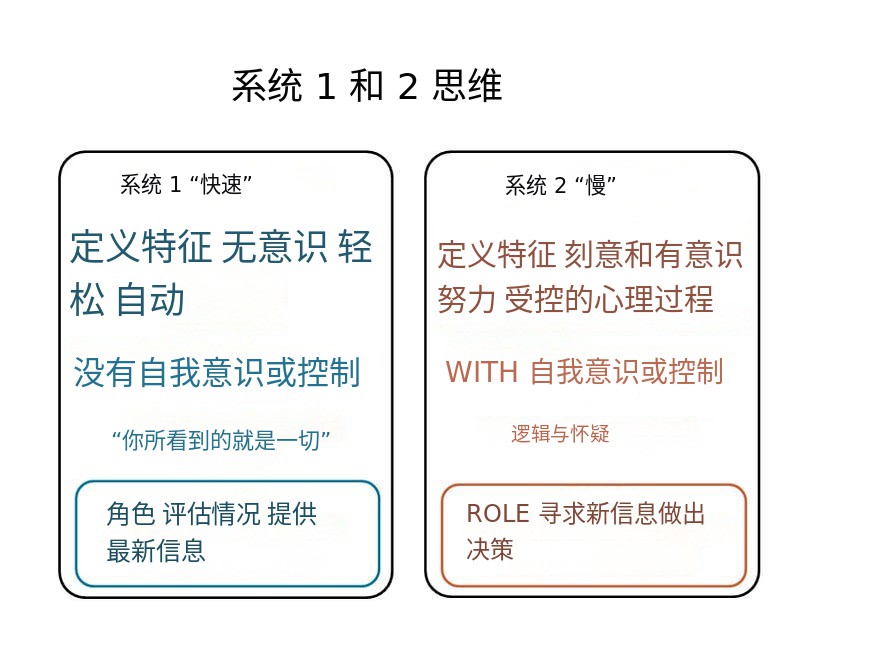
Systems 1 vs 2 and Understanding Biases
系统 1 与系统 2 和了解偏见
System 1 thinking is our default — fast, automatic, and effortless. It operates on intuition, emotions, and learned patterns. Here are some key characteristics of System 1:
系统 1 思维是我们的默认思维--快速、自动、毫不费力。它依靠直觉、情感和习得的模式运作。以下是系统 1 的一些主要特征:
- Fast and Automatic: System 1 processes information quickly and without much conscious effort. It’s the system that works with your reflexes or recognizes your favorite song playing somewhere in a public space. System 1 is always activated and helps you move through the world without being overwhelmed by sensory information. This is why so many ads and media communications rely on imagery and emotional anchoring: these get processed by System 1 before our critical thinking can kick in.
快速自动:系统 1 能快速处理信息,无需太多的有意识努力。它是一个与你的条件反射或识别公共场所播放的你最喜欢的歌曲有关的系统。系统 1 始终处于激活状态,帮助你在这个世界中穿梭,而不会被感官信息淹没。这就是为什么许多广告和媒体传播都依赖于图像和情感锚定:在我们的批判性思维启动之前,这些信息就已经被系统 1 处理过了。 - Pattern Recognition: System 1 excels at identifying patterns and making quick judgments based on past experiences. It uses mental shortcuts called heuristics to navigate the world efficiently.
模式识别:系统 1 擅长识别模式,并根据以往经验快速做出判断。它使用被称为启发式的思维捷径来高效地浏览世界。 - Emotionally Driven: Emotions play a significant role in System 1 thinking. We often make impulsive decisions based on feelings like fear, anger, or excitement.
情感驱动:情绪在系统 1 思维中扮演着重要角色。我们经常会因为恐惧、愤怒或兴奋等情绪而做出冲动的决定。 - Powerful: System 1 drives a majority of our thinking, and can exert influence on our System 2 thinking even when we don’t want it to. Kahnemann has various tests/trick questions that the reader can take throughout TFAS.
强大:系统 1 驱动着我们的大部分思维,即使在我们不希望的情况下,它也能对我们的系统 2 思维施加影响。卡内曼在《TFAS》中为读者提供了各种测试/技巧问题。
I found myself falling for the various tricks, even when I knew the premise of this book and was expecting tricks. Many of these tricks relied on using anchors/frames to make my System 1 focus on certain pieces of information and discard others.
我发现自己被书中的各种技巧所迷惑,即使我知道这本书的前提并期待着这些技巧。许多技巧都依赖于使用锚点/框架,让我的系统 1 专注于某些信息,而放弃其他信息。
This made my System 2 work on ‘Bad Data’ leading to false conclusions.
这使得我的系统 2 在 "坏数据 "上工作,从而得出错误的结论。
System 2: The Slow and Deliberative Thinker
系统 2:缓慢而慎重的思考者
System 2 is the more deliberate and energy-consuming way of thinking. Here’s what sets System 2 apart:
系统 2 是一种更深思熟虑、更耗费精力的思维方式。下面是系统 2 的与众不同之处:
- Slow and Calculated: System 2 takes time and mental effort to activate. It’s used for complex calculations, critical thinking, and problem-solving.
缓慢而缜密:系统 2 需要时间和心力才能激活。它用于复杂的计算、批判性思维和解决问题。 - Logic and Reason: System 2 relies on logic and reason to analyze information and make decisions. It’s the system we use to solve math problems or weigh different options before making a choice.
逻辑与理性:系统 2 依靠逻辑和理性来分析信息和做出决策。这是我们用来解决数学问题或在做出选择前权衡不同选项的系统。 - Limited Capacity: System 2 has limited resources and can easily get overloaded. This is why we tend to rely more on System 1 for most daily tasks (this is a good thing).
容量有限:系统 2 的资源有限,很容易超负荷。这就是为什么我们倾向于在大多数日常任务中更多地依赖系统 1 的原因(这是件好事)。
The way these two systems interact determines how we think.
这两个系统的互动方式决定了我们的思维方式。
How Cognitive Biases Shape the Interactions in our Systems
认知偏差如何影响我们系统中的交互作用
While these systems work together, System 1 often takes the lead. It’s efficient and keeps our brains from being constantly bogged down by analysis paralysis. This is accomplished by the use of Cognitive Biases.
虽然这些系统协同工作,但系统 1 往往起主导作用。它效率很高,让我们的大脑不至于一直陷入分析瘫痪。这是通过使用认知偏差来实现的。
While biases are often treated like a dirty word, they are a value-neutral phenomenon. From an information theory perspective, a bias is simply a shortcut used by a decision-making model (whether our brains or an AI) uses to prioritize and work with information. This can be positive (not eating something b/c it smells funny), value-neutral (my love for chocolate milk), or negative (racial biases).
虽然偏见常常被当作一个肮脏的词,但它却是一种价值中立的现象。从信息论的角度来看,偏差只是决策模型(无论是我们的大脑还是人工智能)用来对信息进行优先排序和处理的捷径。它可以是积极的(闻起来怪怪的就不吃),也可以是价值中立的(我喜欢巧克力牛奶),还可以是消极的(种族偏见)。
The big difference between human and AI biases is simply where it comes from: human bias is architectural while AI bias tells us something deeper about our data.
人类偏见和人工智能偏见的最大区别在于它们的来源:人类偏见是建筑学上的,而人工智能偏见则告诉我们有关数据的更深层次的东西。
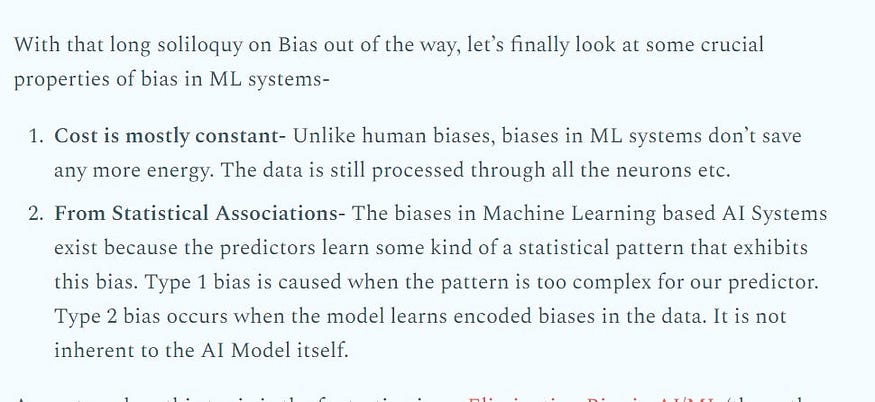
人类与深度学习的偏差以及为何应避免在招聘中使用 GPT
In AI terms, System 1 is a simple checker, while System 2 is a much more powerful Deep Learning model. However, it the role of System 1 extends beyond that.
就人工智能而言,系统 1 是一个简单的检查器,而系统 2 则是一个强大得多的深度学习模型。然而,系统 1 的作用远不止于此。
In most cases, System 1 also acts like our feature extractor/Data Pipeline, directly feeding the input that goes into System 2. Here are 2 major ways how:
在大多数情况下,系统 1 也像我们的特征提取器/数据管道一样,直接为系统 2 提供输入。以下是两种主要方式:
- Confirmation Bias: System 1 confirmation bias reinforces our existing beliefs by seeking out information that confirms them and ignoring information that contradicts them. This creates a skewed perception of reality.
确认偏差:系统 1 确认偏差通过寻找能证实我们现有信念的信息,忽略与之相悖的信息,来强化我们现有的信念。这就造成了对现实的认知偏差。 - Framing Effects: The way information is presented (the “frame”) can influence our decisions even if the underlying facts remain the same. System 1 is more susceptible to framing effects, leading to choices influenced by the presentation rather than logic.
框架效应:即使基本事实保持不变,信息的呈现方式("框架")也会影响我们的决策。系统 1 更容易受到框架效应的影响,从而做出受表述方式而非逻辑影响的选择。
We could spend the entirety of human existence studying why you (yes, you specifically), your mom, and Manchester United’s Transfer Division are the holy trinity of making terrible decisions (Happy Easter to those who celebrate, btw).
我们可以用整个人类的时间来研究为什么你(是的,特别是你)、你妈妈和曼联的转会部门是做出糟糕决定的神圣三位一体(顺便说一句,祝那些庆祝复活节的人复活节快乐)。
But that would involve watching too many clips of Anthony spinning around, so I’ll have to pass on that. Fortunately, risk experts like Filippo Marino have dedicated their lives to answering just that.
不过,这需要看太多安东尼转来转去的片段,所以我还是算了吧。幸运的是,像菲利普-马里诺(Filippo Marino)这样的风险专家毕生致力于回答这个问题。
Our risk judgment is often clouded by an instinctual bias towards the dramatic and memorable. Terrorist attacks, for example, despite their relative rarity, loom large in our collective consciousness. This is a result of the ‘availability heuristic,’ a cognitive shortcut where we inflate the likelihood of events that are eye-catching and easily recalled, while we downplay or disregard the risks associated with more common but statistically more hazardous situations.
我们对风险的判断往往被一种本能的偏好所蒙蔽,即偏好戏剧性的、令人难忘的事件。例如,恐怖袭击尽管相对罕见,但在我们的集体意识中却十分重要。这是 "可得性启发式 "认知捷径的结果,在这种认知捷径中,我们会夸大那些吸引眼球、容易让人联想起的事件的可能性,而淡化或忽视与更常见但从统计角度看更危险的情况相关的风险。Similarly, our judgment is frequently skewed by ‘base rate neglect’ — a tendency to ignore broad statistical information and instead focus on specific, anecdotal accounts. With this bias, our recent experience visiting a foreign city, for instance, can feel more significant than (more predictive) violent crime or road accident rates.
同样,我们的判断经常会受到 "基数忽视 "的影响,即忽视广泛的统计信息,转而关注具体的传闻。在这种偏差的影响下,例如,我们最近去外国城市旅游的经历,可能比(更具预测性的)暴力犯罪率或交通事故率更有意义。In each of these instances, our instinctive risk assessment falls short, underscoring the need for decision-making processes that incorporate sound data and rational analysis.
在上述每一种情况下,我们本能的风险评估都会出现偏差,这就凸显出决策过程需要结合可靠的数据和理性的分析。- Beyond Instinct, by Filippo Marino. The Safe-Esteem substack is a goldmine to help you understand cognitive biases and how they often mess with our risk judgements.
- 超越本能》,作者菲利波-马里诺。安全自尊 "子集是帮助您了解认知偏差以及它们如何经常扰乱我们的风险判断的金矿。
With that out of the way, let’s talk about some of the most powerful ideas discussed in Thinking Fast and Slow.
说完这些,让我们来谈谈《思考:快与慢》中讨论的一些最有力的观点。
Biases, Phenomena, and Frames
偏见、现象和框架
Reversion to the Mean 回归均值
Reversion to the mean describes the statistical tendency for extreme results or events to be followed by less extreme results until things converge back to the average.
回归平均值描述了一种统计趋势,即极端结果或事件之后会出现不那么极端的结果,直到事情趋于平均。
It sounds incredibly obvious, but appreciating its implications is key for any decision-maker (especially for those who work with data).
这听起来非常明显,但对于任何决策者(尤其是数据工作者)来说,理解其含义都是关键所在。
Why does it happen? 为什么会这样?
Several factors contribute to reversion to the mean:
有几个因素会导致向均值回归:
- Random Variation: Any outcome is influenced by chance. An exceptional result may simply be due to a string of good luck/external factors that’s unlikely to repeat itself.
随机变异:任何结果都会受到偶然性的影响。一个特殊的结果可能只是因为一连串的好运气/外部因素,而这种好运气/外部因素不太可能重复出现。 - Measurement Error: Tests and measurements aren’t perfect. Unusually high or low scores may partly be influenced by measurement error, leading to more average results later.
测量误差:测试和测量并不完美。不寻常的高分或低分可能部分受到测量误差的影响,从而导致日后出现更多的平均结果。 - Underlying Stability: Most systems have a natural average or equilibrium they tend to gravitate towards. Deviations from this average will often correct over time.
基本稳定性:大多数系统都有其趋向的自然平均值或平衡点。随着时间的推移,偏离这一平均值的情况往往会得到纠正。
Why it Matters 为何重要
Understanding reversion to the mean is crucial to avoid bad reasoning in various areas:
了解均值回归对于避免在各个领域出现错误推理至关重要:
- Misinterpreting Performance: We need to be careful not to overestimate the impact of one extreme performance, positive or negative. Reversion to the mean suggests that it might be partially fluke, not a true reflection of permanent change.
曲解绩效:我们需要小心谨慎,不要高估一个极端表现的影响,无论是积极的还是消极的。回归均值表明,这可能只是部分侥幸,而不是永久性变化的真实反映。 - Evaluating Interventions: If we make a change (like starting a new training program for employees) and then observe an extreme improvement, it’s tempting to attribute the change solely to the intervention.
评估干预措施:如果我们做出了改变(比如为员工启动一项新的培训计划),然后观察到了极大的改善,那么我们很容易将这种改变完全归因于干预措施。
However, reversion to the mean could also be at play, and we need to take that into account to properly assess results.
不过,均值回归也可能在起作用,我们需要考虑到这一点才能正确评估结果。 - Predicting the Future: Reversion to the mean suggests that extrapolating too far from recent extreme results is often misleading. Future outcomes are likely to be less extreme. This why it is crucial to improve your data sampling and understand the underlying domain, since both help us evaluate whether a particular set of prior data is a good model for predicting the future.
预测未来:回归平均值表明,从最近的极端结果中推断出太远的结果往往会产生误导。未来的结果可能不会那么极端。这就是为什么改进数据采样和了解基础领域至关重要,因为这两者都有助于我们评估特定的先验数据集是否是预测未来的良好模型。
I took a long time to appreciate this one, but now I see it everywhere (including the numbers in my writing).
我花了很长时间才体会到这一点,但现在我到处都能看到它(包括我写作中的数字)。
Loss Aversion 损失厌恶症
Something that changed the way I saw risk-taking was Kahenmann’s study on Loss Aversion. Our drive to avoid a loss is 2.5x stronger than our drive to pursue wins of similar magnitude.
卡恩曼(Kahenmann)关于 "损失厌恶"(Loss Aversion)的研究改变了我对冒险的看法。我们避免损失的动力是追求同等程度胜利的动力的 2.5 倍。
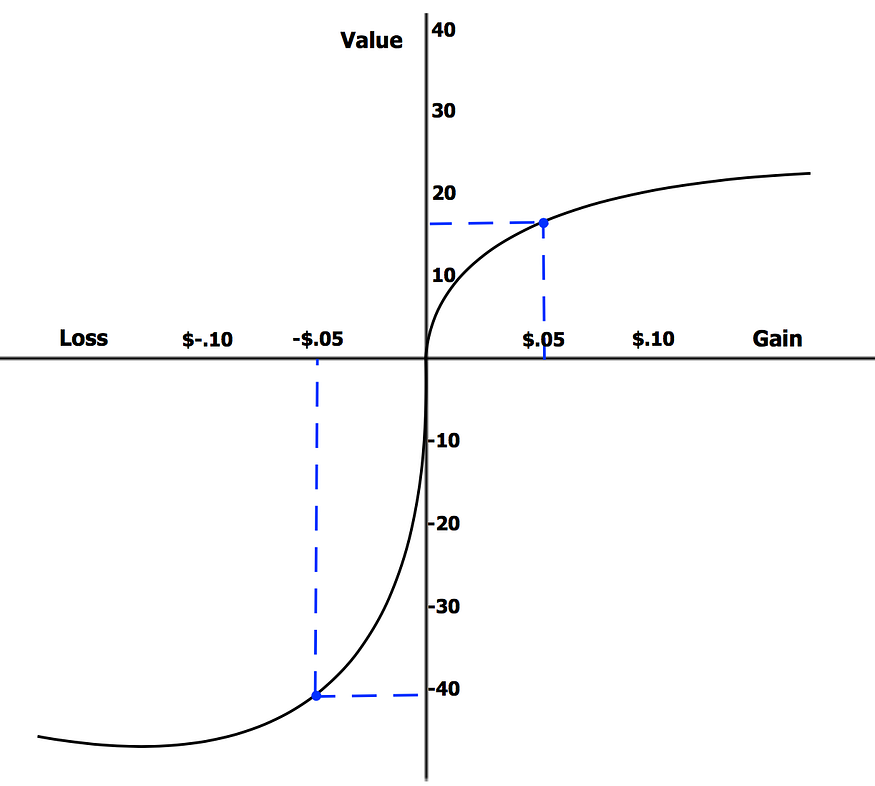
This might’ve risen from an evolutionary context (in the jungle, taking too many risks can lead to death, and the conservative people are the ones that survive). We’ve inherited this, even when it’s not fully suited for the modern world.
这可能源于进化论(在丛林中,冒太多风险会导致死亡,而保守的人才能存活下来)。我们继承了这一点,即使它并不完全适合现代社会。
Understanding Loss Aversion has freed me in a way, and helped me be more experimentative in what I do, both personally and professionally.
对 "损失厌恶 "的理解在某种程度上解放了我,帮助我在个人和专业领域的工作中进行更多尝试。
Anchoring Bias: 锚定偏差:
We have a tendency to become overly fixated on the first piece of information offered (the “anchor”). This is a technique used in salary negotiations to low-ball prospective employees during salary negotiations.
我们往往会过分执着于所提供的第一条信息("锚")。这是薪资谈判中使用的一种技巧,目的是在薪资谈判中压低潜在员工的工资。
The converse is also used by organizations trying to sell us something.
试图向我们推销产品的机构也会反其道而行之。
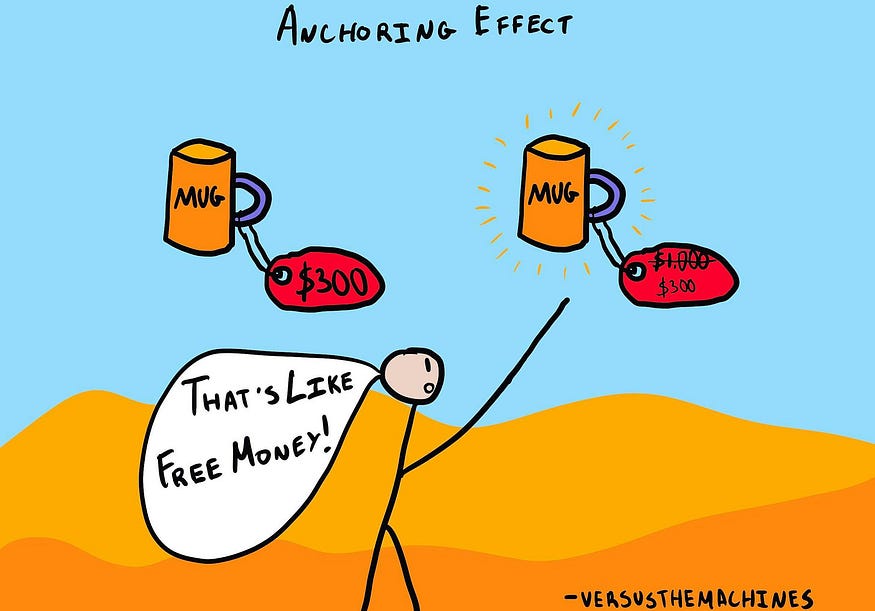
Two things especially blew my mind about the anchoring effect. Firstly, turns out that we can use completely irrelevant things as anchors. This is done very subtly, so being on the lookout for this nudge is key.
关于锚定效应,有两件事尤其让我大吃一惊。首先,原来我们可以使用完全不相关的事物作为锚点。这种做法非常巧妙,所以留意这种暗示很关键。
Secondly, the anchoring bias might worsen the impact of the automation bias (our tendency of accept the results from an automated decision making system more less critically).
其次,锚定偏差可能会加剧自动化偏差(我们倾向于不那么严格地接受自动化决策系统的结果)的影响。
The responses we get from AI machine learning models can potentially trigger the anchoring bias and thus affect decision-making. A response provided by an AI tool may cause individuals to formulate skewed perceptions, anchoring to the first answer they are given.
我们从人工智能机器学习模型中得到的回答可能会引发锚定偏差,从而影响决策。人工智能工具提供的回答可能会导致个人形成偏差的认知,锚定于他们得到的第一个答案。
This allows us to disregard other potential solutions and limits us in our decision-making.
这让我们忽略了其他潜在的解决方案,限制了我们的决策。After being exposed to an initial piece of information, feeling short on time and being preoccupied with many tasks is thought to contribute to insufficient adjustments. But this can be avoided by taking the time and effort to avoid jumping to conclusions. A study by Rastogi and colleagues found that when people took more time to think through the answers provided by the AI, they moved further away from the anchor, decreasing the effect on their decision-making.
人们认为,在接触到最初的信息后,感到时间不够和被许多任务所困扰会导致调整不足。但是,这可以通过花时间和精力避免匆忙下结论来避免。拉斯托吉及其同事的一项研究发现,当人们花更多时间思考人工智能提供的答案时,他们会进一步远离锚点,从而降低对决策的影响。
Framing Effects: 框架效应:
The way information is presented (the “frame”) dramatically influences how we perceive it and the decisions we make. Once again, this has some interesting implications when looking at communications and how they attempt to subtly manipulate perceptions.
信息的呈现方式("框架")极大地影响着我们对信息的感知以及我们做出的决定。当我们审视传播以及它们如何试图巧妙地操纵人们的感知时,这再次产生了一些有趣的影响。
In a study among undergraduate students, respondents were presented with the following medical decision-making problem, described with both a positive and a negative frame. Responses were recorded on a 6-point Likert scale ranging from 1 (very bad) to 6 (very good).
在一项针对本科生的研究中,我们向受访者提出了以下医疗决策问题,并对其进行了正面和负面的描述。受访者的回答采用李克特 6 点量表进行记录,从 1 分(非常糟糕)到 6 分(非常好)不等。Positive: 100 patients took the medicine, and 70 patients got better. How would you evaluate the drug’s effect?
正方:100 名患者服药后,70 名患者的病情有所好转。您如何评价这种药物的效果?Negative: 100 patients took the medicine, and 30 patients didn’t get better. How would you evaluate the drug’s effect?
反方:100 名患者服药后,有 30 名患者的病情没有好转。您如何评价这种药物的效果?The results revealed that the framing influenced the evaluation: when the drug’s effect was described with a loss frame (30 patients didn’t get better), respondents gave negative evaluations.
结果显示,框架对评价有影响:当用损失框架(30 名患者没有好转)来描述药物效果时,受访者会给出负面评价。
When the effect was described with a gain frame (70 patients got better), respondents gave positive evaluations.
当以 "收益"(70 名患者的病情有所好转)来描述效果时,受访者给予了积极的评价。
Many AI Researchers and Marketers understand this very well, and deliberately name their benchmarks/technologies to nudge towards favorable decisions.
许多人工智能研究人员和营销人员都非常了解这一点,他们会故意为自己的基准/技术命名,以引导人们做出有利的决定。
Take the benchmark TruthfulQA for example. Due to its name People utilize it as a test for alignment, unaware of various limitations.Researcher and Youtuber Yannic Kilcher had a fantastic demonstration on its weakness when he fine-tuned an LLM on 4-Chan data and showed that it was the best performer on this benchmark. However, certain sections of the AI Safety crowd got triggered by this, and this led to a massively one-sided coverage of the whole fiasco. Yannic’s bot was framed as a “hate-speech machine”.
以 TruthfulQA 基准为例。顾名思义,人们利用它作为对齐测试,却不知道它存在各种局限性。研究人员兼 Youtuber Yannic Kilcher 在 4-Chan 数据上对 LLM 进行了微调,结果表明它是该基准中表现最好的,从而展示了它的弱点。然而,人工智能安全人群中的某些人却因此受到了刺激,这导致了对整个惨败的片面报道。Yannic 的机器人被诬陷为 "仇恨语音机器"。
Ultimately this controversy led to HuggingFace gating this model (the problems w/ TruthfulQA that this model demonstrated have never been solved to my knowledge).
最终,这一争议导致 HuggingFace 屏蔽了这一模型(据我所知,这一模型所显示的 TruthfulQA 问题从未得到解决)。
FYI: Looking at all the strawmen that the AI Safety/Ethics people created, their constant refusal to engage in good faith discussions, and the spinelessness of AI Academia to call said Ethics folk out on their egregious behavior was one of the biggest reasons I decided not to do a Ph.D. or pursue Professorship (I only started writing to help with my journey into academia).
仅供参考:看着人工智能安全/伦理人士制造的各种稻草人,看着他们始终拒绝参与真诚的讨论,看着人工智能学术界毫无骨气地指责伦理人士的恶劣行径,这是我决定不读博士或不追求教授职位的最大原因之一(我开始写作只是为了帮助我进入学术界)。
Once again, one need only look at the communications by AI Doomers to find countless examples where AI is portrayed as a risk similar to nuclear war, pandemics, or climate change.
同样,只要看看人工智能末日论者的传播,就会发现无数例子都把人工智能描绘成与核战争、大流行病或气候变化类似的风险。
This framing is used to draw out a visceral fear, so that people are more likely to agree with the Doomer. Any agenda-heavy communication will invariably use various types framing of manipulate consumer opinion.
这种陷害被用来引起人们内心的恐惧,从而使人们更有可能同意 "道默 "的观点。任何重议程的传播都会不可避免地使用各种框架来操纵消费者的意见。
Availability Heuristic: 可用性启发式
“Repeat a lie often enough and it becomes the truth”
"谎言重复多次就会变成真理"-Goebbels -戈培尔
We assess the likelihood or frequency of an event by how easily examples come to mind and not by their true base rate. This frequently leads to misjudging true probabilities.
我们在评估一个事件的可能性或频率时,只看其例子是否容易浮现在脑海中,而不看其真实的基本比率。这经常导致对真实概率的错误判断。
Availability Heuristic is particularly nasty when combined with social media. Social Media by it’s very nature tends to push up the most extreme kinds of content/creators.
可用性启发式与社交媒体的结合尤为糟糕。社交媒体就其本质而言,倾向于推崇最极端的内容/创作者。
People that spend a lot of time online can make the mistake of conflating these anomalies with the ‘normal’, and thus feel bad about themselves by comparison.
经常上网的人可能会犯一个错误,就是把这些反常现象与 "正常 "现象混为一谈,从而在比较中对自己产生不好的感觉。
Financial Scammers often prey upon this by constantly bombarding financially illiterate people with constant ads and images of lifestyles, to confuse their marks and ultimately scam into them buying their products/investments.
金融骗子往往利用这一点,不断用广告和生活方式图片轰炸金融盲,混淆他们的视线,最终骗取他们购买自己的产品/投资。
Because these people often use their social proof to sell their products. They rely on massive ad campaigns, where they pay a lot of celebrities, outlets, and other influencers, to promote their products.
因为这些人经常利用他们的社会证明来推销自己的产品。他们依靠大规模的广告活动,花钱请大量名人、网点和其他有影响力的人,来推广自己的产品。
Regular Folks following these people/organizations come across these promotions and google the original product. There, they see the paid releases + massive following and think this product is legit.
关注这些人/组织的普通人会发现这些促销活动,然后谷歌搜索原始产品。在那里,他们会看到付费发布+大量追随者,并认为这个产品是合法的。
So now real people put real money into this project, which further increases the hype behind this, forming a strong feedback loop.
因此,现在真正的人把真金白银投入到这个项目中,这进一步增加了背后的炒作,形成了一个强大的反馈回路。- SBF is a fraud. But he was never the problem
- SBF 是个骗子。但他从来不是问题所在
The crazy about this heuristic is that it doesn’t even have to be true. AI Hype Merchants (both positive and negative) have been using this tactic to build their profiles, scare millions, and sell their cures to problems that no one is having.
这种启发式的疯狂之处在于,它甚至不一定是真的。人工智能炒作商(包括正面和负面的)一直在使用这种策略来建立自己的形象,吓唬数百万人,并推销他们解决无人遇到的问题的方法。
The key is to create an echo chamber where the same message is repeated all the time. Most people don’t have the cognitive bandwidth to take a step back and start evaluating these claims (especially when stated by ‘experts’).
关键是要创建一个回音室,让相同的信息不断重复。大多数人没有足够的认知带宽来退一步开始评估这些说法(尤其是 "专家 "的说法)。
These experts also often combine this with ‘false consensus’ and social proof to avoid intellectual critiques.
这些专家还经常将其与 "虚假共识 "和社会证明结合起来,以避免智力批评。
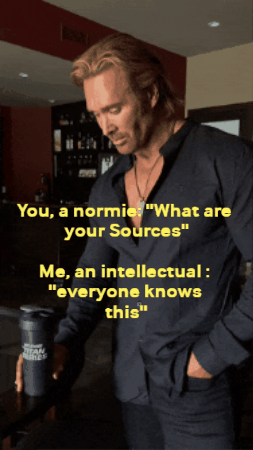
When it comes to the internet, and soo many social media debates, this is probably one of the most important swerves that you should be on the lookout for.
说到互联网,说到这么多社交媒体上的争论,这可能是你应该注意的最重要的转向之一。
Let’s talk about another bias that shows up often in AI discussions.
让我们来谈谈人工智能讨论中经常出现的另一种偏见。
Representativeness Heuristic:
代表性启发式
We make judgments about things or people based on how closely they resemble a typical example, sometimes ignoring statistical probabilities. “For example, if we see a person who is dressed in eccentric clothes and reading a poetry book, we might be more likely to think that they are a poet than an accountant.
我们会根据事物或人物与典型例子的相似程度对其做出判断,有时会忽略统计概率。"例如,如果我们看到一个穿着古怪的人在读一本诗集,我们可能更倾向于认为他是一个诗人,而不是一个会计。
This is because the person’s appearance and behavior are more representative of the stereotype of a poet than an accountant.”
这是因为此人的外表和行为更能代表诗人而非会计师的刻板印象"。
Many conversations about AGI often loop around this heuristic.
许多关于人工智能的讨论常常围绕这一启发式展开。
Dr. Bill Lambos, computational biologist, had a very salient observation about LLMs and their usage of language: LLMs were exceptional at mimicking the behavior of human intelligence and language comprehension but did not display the underlying drivers of intelligence-
计算生物学家比尔-兰波斯博士对LLMs和它们的语言使用有一个非常突出的观察:LLMs在模仿人类智能和语言理解能力方面表现出色,但却没有显示出智能的潜在驱动力--LLMs的语言使用。
The answer is that AI programs seem more human-like. They interact with us through language and without assistance from other people. They can respond to us in ways that imitate human communication and cognition.
答案是,人工智能程序看起来更像人类。它们通过语言与我们互动,无需他人协助。它们可以模仿人类的交流和认知方式来回应我们。
It is therefore natural to assume the output of generative AI implies human intelligence. The truth, however, is that AI systems are capable only of mimicking human intelligence.
因此,人们很自然地认为,生成式人工智能的输出意味着人类智慧。但事实上,人工智能系统只能模仿人类智能。
By their nature, they lack definitional human attributes such as sentience, agency, meaning, or the appreciation of human intention.
就其本质而言,它们缺乏人类的定义属性,如知觉、能动性、意义或对人类意图的理解。-AI is Truly Brainless… -人工智能是真正的无脑...
In his recent instant classic, Logan Thornloe expressed something similar about Devin, i.e- that a lot of the hype/fear about Devin replacing software engineers exists because many people don’t truly understand what a software engineer is supposed to do.
Logan Thornloe 在他最近的即时经典文章中也对 Devin 表达了类似的看法,即很多人对 Devin 取代软件工程师的炒作/恐惧,是因为很多人并不真正了解软件工程师应该做什么。
Since Devin seems like it does the same things as a software engineer, people assume that is a replacement for one.
由于 Devin 似乎做着与软件工程师相同的工作,因此人们认为它可以替代软件工程师。
The supposed most techno-literate group of society has become the most fear-based regarding AI advancement. There are certain tasks AI is good at and they’ll replace those tasks within jobs first. This isn’t exclusive to software engineering. There are aspects of all jobs that will be automated soon and many more that will take a longer time for AI to do so. By the time software engineers are fully replaced, there are many other jobs that will already have been replaced.
本应是最懂技术的社会群体,却对人工智能的发展产生了最大的恐惧。人工智能擅长某些工作,它们会首先取代这些工作。这并不局限于软件工程。所有工作中都有一些方面将很快实现自动化,而更多工作则需要人工智能花更长的时间才能实现自动化。当软件工程师完全被取代时,还有许多其他工作也将被取代。
Why software engineers are the group panicking most about AI taking jobs, I will never understand.
我永远不明白,为什么软件工程师是最担心人工智能抢走工作的群体。The release of Devin has uncovered two things about most software engineers that are way more interesting to me than the autonomous engineer:
Devin 的发布揭示了大多数软件工程师的两点,这两点对我来说比自主工程师更有趣:1.Most engineers have no idea what their job actual consists of and why they’re paid well.
1.大多数工程师都不知道自己的工作到底是什么,也不知道自己为什么能拿到高薪。2.Most engineers seriously lack an understanding of machine learning.
2.大多数工程师严重缺乏对机器学习的了解。-Devin Has Exposed a Major Issue with Software Engineering
-德文暴露了软件工程的一个重大问题
In both cases, we see this bias at play: AI does a particular task related to competency, so we assume that the AI displays the underlying competency. AI Doomers often utilize a similar fallacy when talking about how OS AI can be used to create biological weapons.
在这两种情况下,我们都能看到这种偏见在起作用:人工智能完成了一项与能力相关的特定任务,因此我们假定人工智能展示了基本能力。人工智能末日论者在谈论操作系统人工智能如何被用来制造生物武器时,也经常使用类似的谬论。
Keep this one in mind as you observe communications. You’ll see how often people utilize this to manipulate opinion in their favor.
在观察交流时,请牢记这一点。你会发现,人们经常利用这一点来操纵舆论,使其对自己有利。
Thinking Fast and Slow is a book I go back to every year, and each year it teaches me something new. Its dense material means that it has a lot of re-reading value since there’s always a nuance or an idea that I missed in previous interactions.
《思考得快与慢》是我每年都会翻阅的一本书,每年它都会教给我一些新的东西。这本书的内容密集,因此有很多重读的价值,因为总有一些细微差别或想法是我在之前的交流中忽略的。
It’s a book that will hook into your soul and permanently mold its texture into something different. It does what all great books/media do: takes you on a journey of self-discovery. If you haven’t had the opportunity to read it yet, now’s a good time.
这本书会勾住你的灵魂,永久地塑造出与众不同的质感。它做了所有伟大书籍/媒体都会做的事情:带你踏上自我发现之旅。如果你还没有机会阅读这本书,现在正是时候。
If you have read it already, let me know what your experience with the book was.
如果您已经读过这本书,请告诉我您的阅读感受。
This is quite different from the usual pieces that I do. In case you’re itching for something more technical: check out my conversation with the amazing Sean Falconer. We dive deep into the world of prompt injection attacks in Large Language Models (LLMs). Our conversation covers LLM injection attacks, existing vulnerabilities, real-world examples, and the strategies attackers use.
这与我平时做的作品截然不同。如果您想了解更多技术方面的内容:请查看我与了不起的 Sean Falconer 的对话。我们深入探讨了大型语言模型 (LLMs) 中的提示注入攻击。我们的对话涉及 LLM 注入攻击、现有漏洞、实际示例和攻击者使用的策略。
Got great feedback from the viewers, and I’m sure you’d enjoy it too.
观众反馈很好,我相信你也会喜欢的。
That is it for this piece. I appreciate your time. As always, if you’re interested in working with me or checking out my other work, my links will be at the end of this email/post. And if you found value in this write-up, I would appreciate you sharing it with more people. It is word-of-mouth referrals like yours that help me grow.
这篇稿子就写到这里。感谢您的宝贵时间。如果您有兴趣与我合作或了解我的其他作品,我的链接会在这封电子邮件/帖子的末尾。如果您觉得这篇文章有价值,我希望您能与更多人分享。正是像您这样的口碑推荐帮助我成长。

I put a lot of effort into creating work that is informative, useful, and independent from undue influence. If you’d like to support my writing, please consider becoming a paid subscriber to this newsletter.
我花了很多精力来创作信息量大、有用且不受不当影响的作品。如果您想支持我的写作,请考虑成为本时事通讯的付费订阅者。
Doing so helps me put more effort into writing/research, reach more people, and supports my crippling chocolate milk addiction. Help me democratize the most important ideas in AI Research and Engineering to over 100K readers weekly.
这样做可以帮助我投入更多精力进行写作/研究,让更多人了解我,还能让我过足巧克力牛奶瘾。帮助我向每周超过 10 万的读者传播人工智能研究和工程领域最重要的观点。
Help me buy chocolate milk
帮我买巧克力牛奶
PS- We follow a “pay what you can” model, which allows you to support within your means. Check out this post for more details and to find a plan that works for you.
PS- 我们采用 "量力而行 "的模式,让您量力而行。详情请查看这篇文章,找到适合您的计划。
I regularly share mini-updates on what I read on the Microblogging sites X(https://twitter.com/Machine01776819), Threads(https://www.threads.net/@iseethings404), and TikTok(https://www.tiktok.com/@devansh_ai_made_simple)- so follow me there if you’re interested in keeping up with my learnings.
我定期在微博网站 X(https://twitter.com/Machine01776819)、Threads(https://www.threads.net/@iseethings404)和 TikTok(https://www.tiktok.com/@devansh_ai_made_simple) 上分享我所读到的小更新--如果你有兴趣跟上我的学习进度,请关注我的微博。
Reach out to me 联系我
Use the links below to check out my other content, learn more about tutoring, reach out to me about projects, or just to say hi.
请使用下面的链接查看我的其他内容,了解更多家教信息,就项目与我联系,或者只是打个招呼。
Small Snippets about Tech, AI and Machine Learning over here
有关技术、人工智能和机器学习的小段子,请点击此处
Check out my other articles on Medium. : https://rb.gy/zn1aiu
查看我在 Medium 上的其他文章。 : https://rb.gy/zn1aiu
My YouTube: https://rb.gy/88iwdd
我的 YouTube: https://rb.gy/88iwdd
Reach out to me on LinkedIn. Let’s connect: https://rb.gy/m5ok2y
在 LinkedIn 上联系我。让我们联系: https://rb.gy/m5ok2y
My Instagram: https://rb.gy/gmvuy9
我的 Instagram: https://rb.gy/gmvuy9
My Twitter: https://twitter.com/Machine01776819
我的 Twitter: https://twitter.com/Machine01776819