The Mantra of This AI
Age: Don’t Repeat Yourself
这个时代的箴言:不要重复自己
AI won't kill your job. But it will steal your repetitive tasks.
人工智能不会取代你的工作,但会帮你处理重复性的任务。
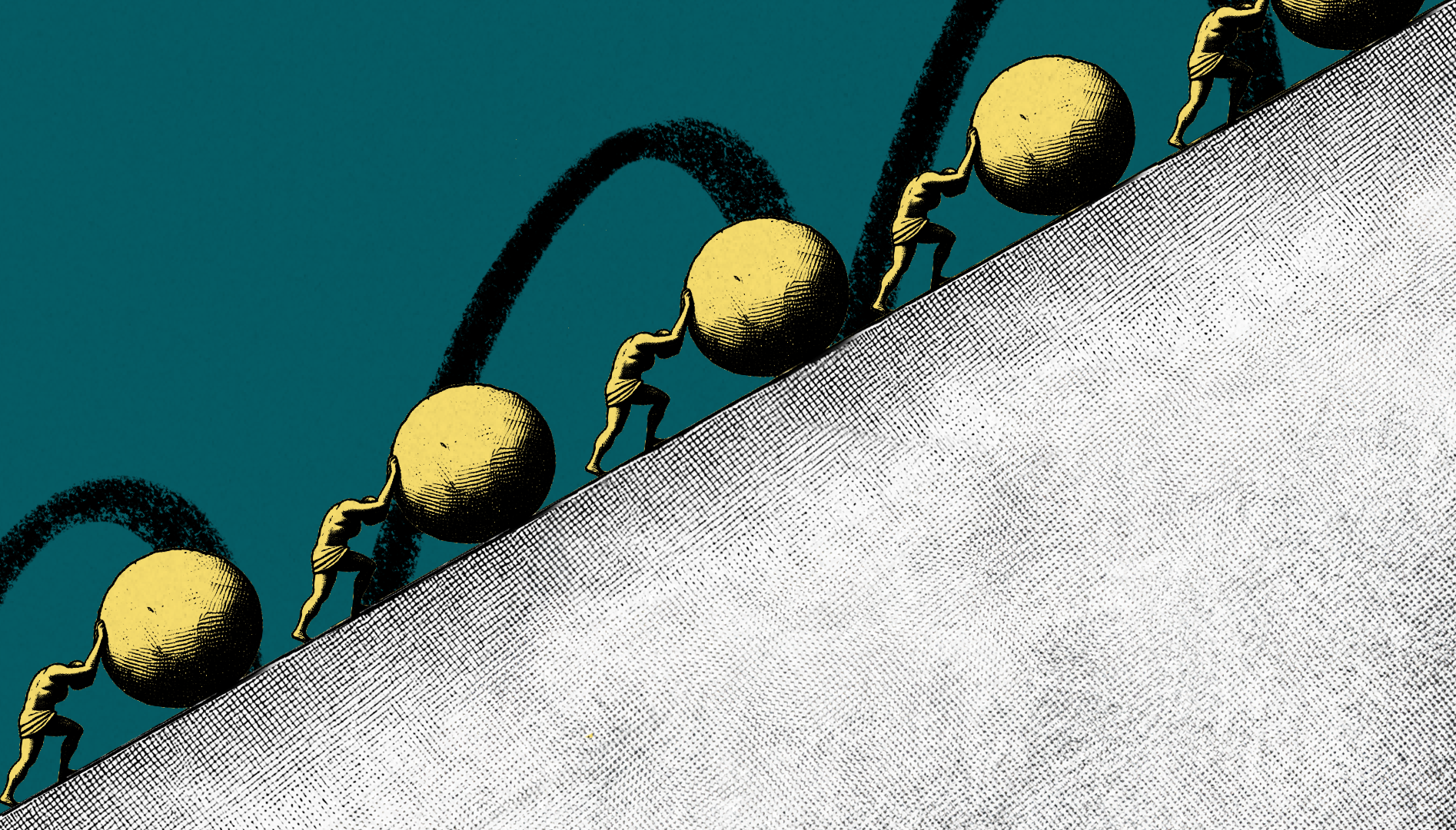
DALL-E/所有插图。
Was this newsletter forwarded to you? Sign up to get it in your inbox.
这个新闻通讯是转发给你的吗?注册后可以直接发送到你的邮箱。
Contrary to popular belief, this generation of artificial intelligence technology is not going to replace every single job. It’s not going to lead employers to fire every knowledge worker. It’s not going to obviate the need for human writing. It’s not going to destroy the world. We don’t have to strafe the data centers or storm Silicon Valley’s top labs.
与普遍看法相反,这一代人工智能技术并不会取代所有工作,也不会导致雇主解雇所有知识工作者。它不会消除人类写作的需求,更不会摧毁世界。我们不需要轰炸数据中心或攻占硅谷的顶尖实验室。
The current generation of AI technology doesn’t live up to the AGI hype in that it can’t figure out problems that it hasn’t encountered, in some way, during its training. Neither does it learn from experience. It struggles with modus ponens. It is not a god.
目前的人工智能技术并未达到通用人工智能的期望,因为它无法解决在训练中未曾遇到过的问题,也无法从经验中学习。它在处理模态前提时存在困难。它并不是神。
It does, however, very much live up to the hype in that it’s broadly useful for a dizzying variety of tasks, performing at an expert level for many of them. In a sense, it’s like having 10,000 Ph.D.’s available at your fingertips.
不过,它确实非常符合预期,因为它在各种任务中都非常实用,并且在许多任务上表现得像专家一样。从某种意义上说,这就像你手边有 10,000 个博士学位。
The joke about Ph.D.’s is that any given academic tends to know more and more about less and less. They can talk fluently about their own area of study—maybe, the mating habits of giant isopods, or 16th-century Flemish lace-making techniques. But if you put them to work in an entirely new domain that requires the flexibility to learn a different kind of skill—say, filling in as a maître d' during dinner rush at a fancy Manhattan bistro—they’ll tend to flounder.
关于博士的笑话是,任何学者往往对越来越少的事情了解得越来越多。他们可以流利地谈论自己研究的领域——比如巨型等足虫的交配习性,或者 16 世纪佛兰德的蕾丝制作技巧。但如果让他们在一个全新的领域工作,需要灵活学习不同的技能——比如在曼哈顿一家高档餐厅的晚餐高峰期担任领班——他们往往会感到无所适从。
That’s a little like what language models are. Imagine a group of Ph.D.’s versed in all of human knowledge—everything from the most bizarre academic topics to the finer points of making a peanut butter and jelly sandwich. Now imagine tying all of the Ph.D.s together with a rope and hoisting a metal sign above them that says, “Answers questions for $0.0002,” with a little slot to insert your question. By routing the question to the appropriate Ph.D., this group would know a lot about a lot, but they still might fail at a task sufficiently new to the recorded sum of human knowledge.
这有点像语言模型。想象一下,一群精通人类知识的博士——从最奇特的学术主题到制作花生酱和果冻三明治的细节。现在想象把这些博士用绳子绑在一起,并在他们上方悬挂一个金属标志,上面写着“回答问题只需$0.0002”,还有一个小槽可以插入你的问题。通过将问题转交给合适的博士,这个团队对很多事情都有深入了解,但在某些对人类知识总和来说足够新颖的任务上,他们仍然可能会失败。
This is in line with University of Washington linguistics professor Dr. Emily Bender’s idea of the “stochastic parrot”—that language models are just regurgitating sequences of characters probabilistically based on what they’ve seen in their training data, but without really knowing the “meaning” of the characters themselves.
这与华盛顿大学语言学教授艾米莉·本德博士提出的“随机鹦鹉”理论相符——语言模型只是根据训练数据中看到的内容,以概率的方式重复字符序列,但并不真正理解这些字符的“意义”。
It’s also in line with observations made by Yann LeCun, chief AI scientist at Meta, who has repeatedly said that large language models can’t answer questions or solve problems that they haven’t been trained on.
这与 Meta 的首席人工智能科学家 Yann LeCun 的观察一致,他多次指出,大型语言模型无法回答或解决它们没有接受过训练的问题。
There’s room to quibble with whether both of their takes truly represent the current state of the technology. But even if you grant their point, what both Bender and LeCun misunderstand is that they think the powers of the current generation of AI technology is a letdown. They say, in a pejorative sense, that language models are only answering questions they’ve seen in some form in their training data.
有人可能会对他们的观点是否真正反映当前技术的状态提出异议。但即使你承认他们的看法,Bender 和 LeCun 都误解了当前一代人工智能技术的能力,他们认为这是一种失望。他们以贬义的方式说,语言模型只是回答他们在训练数据中见过的某些问题。
I think we should get rid of the “only.” Language models are answering questions they’ve seen before in their training data. HOLY SHIT! That is amazing. What a crazy and important innovation.
我觉得我们应该去掉“仅仅”这个词。语言模型正在回答它们在训练数据中见过的问题。真是太惊人了!这是一项疯狂而重要的创新。
LLMs allow us to tap into a vast reservoir of human knowledge and expertise with unprecedented ease and speed. We’re no longer limited by our individual experiences or education. Instead, we can leverage the collective wisdom of humanity to tackle challenges and explore new frontiers.
LLMs 让我们以前所未有的便利和速度接触到人类知识和专业的丰富资源。我们不再局限于个人的经历或教育,而是可以利用人类的集体智慧来应对挑战,探索新的领域。
For anyone trying to figure out what to use AI for, or what kinds of products to build with the current generation of technology, it implies a simple idea: Don’t repeat yourself.
对于那些想要弄清楚如何利用人工智能或用当前技术构建什么产品的人来说,这传达了一个简单的理念:不要重复自己。
Because language models are good at doing anything they’ve seen done before, they’re good at any human task that’s repetitive. New technology changes how we see the world, and what language models reveal is that there is a lot of our day-to-day that is repetitive.
由于语言模型擅长处理他们曾经见过的任务,因此它们在任何重复性的人类工作中表现出色。新技术改变了我们对世界的看法,而语言模型所揭示的是,我们的日常生活中有很多内容是重复的。
Take the humble startup founder, for example. Most people start a company because they want to build new things, discover new frontiers, and explore what can be unburdened by what has been.
以谦逊的创业者为例。大多数人创办公司是因为他们希望创造新事物,开拓新领域,探索那些不再受过去束缚的可能性。
The reality is that founder life is sometimes like that, but very often it consists of repeating yourself over and over in various, subtly different, contexts. You have to repeat yourself to investors when you pitch them, giving the same biographical details, and telling the same anecdotes over and over again. You do the same thing with potential customers, new hires, and journalists writing articles about you.
现实是,创始人的生活有时确实如此,但更多时候,它是在不同的微妙背景下反复自我重复。你在向投资者推介时需要不断重复自己,提供相同的个人经历和一次又一次讲述相同的故事。你对潜在客户、新员工以及撰写关于你的文章的记者也都是如此。
You have to repeat yourself with your team—to reinforce the mission, your values, and norms, and how to think about solving problems.
你需要与团队反复强调使命、价值观和规范,以及如何思考解决问题。
It’s a repetitive job, and that’s part of what makes it so hard. You have to say the same thing all the time without losing enthusiasm, and do so dozens or even hundreds of times a day.
这是一份重复的工作,这正是它如此困难的原因。你必须不断地说同样的话而不失去热情,每天要这样做几十次甚至上百次。
Language models allow us to see this repetition, this drudgery, in a new way because we finally have a solution for it. Imagine Slack bots and email copilots that automatically jump in to answer repetitive questions or provide a first round of feedback by simulating the founder’s perspective in scenarios that have come up before. It would dramatically ease the burden on the founder’s time, opening them up to handle more important work.
语言模型让我们以全新的视角看待这种重复和乏味,因为我们终于找到了应对之策。试想一下,Slack 机器人和电子邮件助手能够自动介入,回答重复性问题,或者通过模拟创始人的视角在以往出现过的场景中提供初步反馈。这将大大减轻创始人的时间压力,让他们有更多精力去处理更重要的工作。
Language models expose how much of this same drudgery occurs in every field of human endeavor, and how it might be streamlined.
语言模型展示了在各个领域的人类活动中有多少重复的繁琐工作,以及如何能够优化这些过程。
This shift in how we see the world aligns with what I've previously called the allocation economy. As AI takes over these repetitive tasks, our role changes from doing the work ourselves, to deciding what work needs to be done and how to best allocate our resources to do it.
这种我们看待世界的转变与我之前提到的分配经济相一致。随着人工智能接管这些重复性任务,我们的角色从亲自完成工作转变为决定需要做哪些工作,以及如何最佳地分配资源来完成这些工作。
In the allocation economy, the key skill becomes knowing how to effectively leverage AI to handle these repetitive elements, freeing us up for more creative and strategic thinking.
在分配经济中,关键技能是有效利用人工智能处理重复性工作,从而让我们有更多时间进行创造性和战略性思考。
So, if you’re wondering how your world might change over the next few years, do a little experiment today. Try to notice all of the ways you’re repeating yourself.
所以,如果你想知道未来几年你的世界可能会如何变化,今天可以做个小实验。试着留意一下你是如何重复自己的。
Soon enough, language models will be doing a lot of that stuff for you. And that will free you up to do more interesting things.
不久之后,语言模型将为你处理许多这样的事务,这样你就可以腾出时间去做更有趣的事情。
Dan Shipper is the cofounder and CEO of Every, where he writes the Chain of Thought column and hosts the podcast AI & I. You can follow him on X at @danshipper and on LinkedIn, and Every on X at @every and on LinkedIn.
Dan Shipper 是 Every 的联合创始人兼首席执行官,他在这里撰写“思维链”专栏,并主持播客《AI 与我》。你可以在 X 平台上关注他,用户名是@danshipper,也可以在 LinkedIn 上找到他,Every 的 X 用户名是@every,LinkedIn 上也可以关注。
Comments 评论内容
乔治亚·帕特里克在大约 19 小时前的消息
Ahhhhhh!!! That's the Dan Shipper I fell in love with the first time. Clear. Uncomplicated. One main point and no need to crawl through multiple screenshots. We can feel the authority--the lived experience of Dan. He didn't get this from a book or a video. He got this by doing the work and then reporting back to all the Earthlings in a simple exercise we can all do, immediately: Take the time, just for a day, to get aware of your every movement, write it down, and then, tonight or tomorrow, notice where there is repetition. Then make a good decision. Genius.
啊啊啊啊啊!!!那就是我第一次爱上丹·希普的样子。清晰而简单。只有一个主要观点,不需要翻阅多个截图。我们能感受到他的权威——丹的生活经验。他不是从书本或视频中学到这些,而是通过实践,然后向所有地球人分享,做一个我们都可以立即进行的简单练习:花一天的时间,关注你每一个动作,记录下来,然后,今晚或明天,注意哪里有重复。然后做出一个明智的决定。真是天才。
丹·希珀大约在一个小时前
@georgia@communicators.com thanks Georgia!
@georgia@communicators.com 感谢乔治亚!
@jdricher2017 约 19 小时前
Fantastic 精彩