请问有谁真的成功借助chatgpt写论文的吗?
创建时间:2023-02-09 17:57:05
最后编辑:2023-02-09 18:10:44
update: 09/07/2023 我已经把一个具体的例子,怎么从无到有迭代的例子,放在的最后面。
以下是原回答
我已经使用chatgpt写了大半年的各种英文论文和内部报告了。
首先,毫无疑问的,通过使用chatgpt,我的书写能力直线上升,得到了老板和同事的一致好评。当然,这里面也有一个必须要说清楚的。我怎么的也是正经的一步步考G考托,硕士博士这样走过来的。早在使用chatgpt之前,我已经有了近10年的科技写作经验,并且也通过了同行的评议,……。自我标榜的话就不说了,简而言之,我不是菜鸟,我是老鸟。
于我而言,chatgpt能帮助我解决几个非常困难的痛点,过往都需要我做大量的工作,如今,依靠chatgpt基本分分钟搞定。
- polish/rephrase:大多数情况,我不需要它帮我添加内容,我只需要它帮我改写的更好。尤其是在有字数限制的各种场合。用chatgpt进行缩写和扩写到规定字数,简单又方便。
- explain:很多时候,作为国人中的一员,我也经常为了不能以简单的方式把事情讲述清楚而困扰。早年间,导师教的办法,是能用数学表达式的,就别用英语。但是还是很多情况,并不适合使用数学表达式。这时候,我就可以写出来一堆啰里八嗦的话,然后让chatgpt给整理清楚。然后我再仔细校对下,看看有没有miss的点,或者臆想出来的内容,一般两三轮对话就能弄清楚了。
- title:写文章里面,最重要的,也常常被忽视的就是题目。用chatgpt来起题目,感觉棒棒的。尤其是那种带缩写的题目,多给点例子,in-context learning,提出来的题目,当真是不要太好。
- plot:尤其是画那种不怎么擅长,没怎么画过的图。比如那种很fancy的radar plot。Chatgpt基本可以做到,直接给数据,它就能画。多提点建议也很容易搞定,比如用个log scale,改个字体大小 —— 以上的每一个小改动在过去,都可能画上我十几二十分钟;因为可能要用到一个新的plot的库,而我对syntax不熟悉。
以上四条,放在原来,基本要在我文章大体成型之后,还要花上一个礼拜,甚至两个礼拜的全职时间。如今依靠chatgpt,只要1,最多2天就能搞定。
我知道很多人心里想的挺美,直接给个提纲,然后就指望chatgpt给你把所有内容都给生成出来。这大概是真的想多了。你们永远要记住,chatgpt是个通才模型,而不是个专才模型。
正因为,他是通才模型,他才能各种学科都能胜任。当然也因为同样的原因,他是不可能写到你专业博士写论文的那个深度的 —— 注意哈,我说的是不会,而不是不能。
下面可以来说说,怎么样才能让他写到你想要的内容。很不幸,要达到这个目标的先决条件是,你自己是个专家,至少应该是个二把刀的专家 —— 你自己可以写不出来,你觉得好的东西,但是你要自己看过很多写的很有好的东西,以至于说,当chatgpt写出来一段文章的时候,你可以有目的性的,让他进行修改。翻译成白话,那就是,你可以没吃过猪肉,但是你要至少看过猪跑。
更好的做法是,你可以考虑使用in-context learning (给你觉得写的好的例子), 或者retrieval-augmented generation(提供额外的背景信息和内容)的办法,使得chatgpt写出来更加有深度,带洞察力的文章。当问题任务困难的时候,也可以考虑使用chain-of-thoughts (帮助分解一个大问题到一步步的小问题)来解决。
总体来说,有了chatgpt之后,我在乎的只是自己的token是不是要用完了。基本我花在写上面的时间,不说缩减了一半,也差不多了 —— 毕竟,我的习惯,还是自己先写初稿,然后用chatgpt进行迭代,所以真正缩减的,其实是迭代的时间。
-- 前方高能 ---- 前方高能 ---- 前方高能 ---- 前方高能 ---- 前方高能 ---- 前方高能 --
update:09/07/2023。省流提示,这是一个完整的例子,怎么从无到有,怎么一步步迭代,中间我是怎么想的,chatgpt又是怎么回应的,……
虽然不能匿名了,但我不想被人肉。我今天花这么多token,来说这个事情,是因为想要尽量说清楚,如何依靠chatgpt来增强个人的生产力,实现有赚钱有摸鱼的快乐。
其实和题目更加相关的例子是,给你们看一篇文章的不同阶段,但是吧,用已经发表的,会直接被定位;用么有发表的,容易被白嫖。另外呢,这样做,也会违背我这个回答的初衷 —— 我是想要大多数人明白我在干什么;而不是去做一个只有某个专业,某个领域的读者才能明白的事情。
为了要大多数人明白整个这个过程,我在这里进行了反向操作 —— 我放弃了用chatgpt,来写某个非常专业的领域或者技术;而是使用它来以一个浅显易懂的方式来解释一个技术,并且在里面加上各种要求和限制。
请注意,我要传递的是方法,而不是具体的这个例子。并且我跟大家分享的例子,也不是那种神奇的单个prompt的例子。而是一个更加贴近现实的例子 —— 一个一开始,我也不知道应该怎么写,写什么的例子。我只是有个朦胧idea,知道现在这个写出来的东西,合适与否,好不好。
所以,我在这里,找个了大多数人听过,但是又不怎么完全了解的东西了 —— RLHF (reinforcement learning with human feedback)。
对就是那个在chatgpt里面用的技术。以下所有的引用部分中标注了ChatGPT的,都是chatgpt4的结果。未有删减或增加。
为了更加符合现实中的问题,我还添加了以下的额外描述
背景:大老板发了我个消息,问我,什么是RLHF。说,等下找我一起喝咖啡。
具体的任务:我要准备,给大老板解释清楚,RLHF是个什么技术。
先预警下,这来来回回的多次调教,和编写知乎,加起来用了我三个小时,所以,如果你要都看完,可能也要个十几分钟。另外,你会看到我的回答和提示里面,会有额外的内容和信息。这并不是我在故意的,以加私货的方式,得到更好的答案,而是一个非常贴近真实的操作 —— 你人是没可能一下子就能把自己的需求,方方面面,在第一次就想的清清楚楚,明明白白的。Chatgpt在迭代,你也在迭代。
Round 1: 先来裸奔一波~,当然,如你们所想的,这肯定是不行的。不然还要什么调教?
我:
Write me an explanation about the RLHF (reinforcement learning with human feedback) technologies. I will later talk it to my CEO.
ChatGPT:
Certainly! Reinforcement Learning with Human Feedback (RLHF) is an advanced technology that merges traditional reinforcement learning (RL) with human guidance to optimize system performance in complex tasks that are difficult to define using straightforward rules or algorithms. In other words, it blends machine intelligence with human intuition to make decisions in a more robust, safe, and effective manner. Here's how to explain it to your CEO in a straightforward manner:
1. What is Reinforcement Learning (RL)?
Reinforcement Learning (RL) is a type of machine learning where an agent learns to interact with its environment to achieve a particular goal. The agent learns by receiving rewards or punishments based on its actions, optimizing its strategy over time to maximize the cumulative reward.
1.1 Components of RL:
- Agent: The entity that takes actions
- Environment: The context in which the agent operates
- State: The current situation of the agent
- Action: A move made by the agent
- Reward: Feedback given to the agent based on its actions
- Policy: The strategy that the agent employs to take actions
2. What is RLHF?
Reinforcement Learning with Human Feedback (RLHF) is an emerging paradigm that combines traditional reinforcement learning algorithms with human-generated feedback to create models that are both effective and aligned with human values. This report aims to provide a comprehensive overview of RLHF technologies, focusing on their potential, mechanisms, advantages, and drawbacks. Understanding this technology is critical for making informed decisions on its integration into your business strategy.
3. Why RLHF?
3.1 Shortcomings of Traditional RL:
- Sparse Rewards: In many real-world problems, rewards are not immediate, making it difficult for the agent to learn effectively.
- Misalignment: The agent's objective might not perfectly align with human values or goals, leading to unintended consequences.
- Sample Inefficiency: Traditional RL often requires a large number of trials and errors, which can be costly or infeasible in some environments.
3.2 Benefits of RLHF:
- Human Alignment: Incorporating human feedback helps the model understand and align better with human values and objectives.
- Efficiency: The human feedback can act as a more frequent and informative reward signal, allowing the agent to learn faster.
- Safety: Humans can help the agent avoid making dangerous or costly mistakes.
4. How RLHF Works
4.1 Core Mechanisms:
- Pre-training: The agent is initially trained on a dataset that includes human feedback, actions, and possible rewards.
- Fine-tuning: The model undergoes further training in the environment, supplemented with ongoing human feedback.
- Reward Modeling: Human feedback is converted into a reward model that the agent uses to evaluate its actions.
- Policy Optimization: The agent adjusts its policy based on both the environmental rewards and the reward model built from human feedback.
4.2 Types of Human Feedback:
- Comparison Data: Humans compare two or more actions and indicate which is better.
- Rankings: Humans rank multiple actions in terms of their effectiveness or desirability.
- Direct Feedback: Humans provide explicit rewards or penalties based on the agent’s actions.
5. Applications
- Healthcare: Personalized treatment recommendations
- Finance: Automated trading strategies that align with ethical investment criteria
- Customer Service: Chatbots that understand nuanced human sentiment
- Robotics: Robots that interact safely and effectively with human
- Supply Chain Management: Optimized, yet ethical, logistics planning
6. Challenges and Risks
- Quality of Human Feedback: If the human feedback is inconsistent or biased, the model will learn incorrectly.
- Scalability: Continual human involvement may be resource-intensive.
- Overfitting: The agent may become too specialized in the tasks it was trained on and may not generalize well to new tasks.
- Ethical Concerns: Decisions made based on human feedback may not be universally acceptable, leading to ethical dilemmas.
7. Conclusion
RLHF presents a promising avenue for creating intelligent agents that can learn effectively while aligning closely with human objectives and values. Given its potential to dramatically improve efficiency and effectiveness in a variety of applications, RLHF technologies warrant serious consideration for inclusion in our strategic technology roadmap.
By understanding the mechanisms, applications, and challenges of RLHF, we can make well-informed decisions on how to leverage this technology for maximum impact and ROI.
感觉,写的怎么样?其实打一眼看上去,还是可以的。至少这要比一个纯小白写出来的,更加有逻辑性,解释了最基本的如同,what,why,how的问题。
那这个chatgpt写的这个东西合格了吗?取决于你的对象,在我这个任务里面,他显然是不合格的。这东西,他写的更像是个学术或者科普文章。哪里像给CEO解释的东西?不用你照着说到第二段,就应该被老板打断了。
不合格的地方具体的地方在哪里呢?有这么几个方面,
- 某些内容太具体了,比如components of RL,大老板基本不会去关心这些细节。
- 某些内容又太抽象了,比如在引言的部分说的什么human values,又在某些部分假定读者有不错的机器学习的专业背景。
- 某些内容有些不恰当,比如整个应用的部分,大老板让做调研,或者下面主动做这个事情,肯定已经有了想法的。脱离这些去谈什么医疗,金融区的应用,显然不合时宜。
- 某些老板关心的内容,比如成本,时间,技术,等等,都没有涵盖清楚。
Round 2: 提供具体的改进的意见。注意,设置好这个任务的context,很重要。
好了,有了上面的一些观察,你接下来就能去迭代了。记住,尽量明确你的要求。
这里有一个在后面你们会看到很起作用的描述,那就是我不但告诉了chatgpt它要写的文字,所要面对的对象是一个不怎么懂技术的CEO,更是明确的告诉了他,解释技术的时候,不要过于纠结于技术细节,反而要尽量用生活中的例子和类比,帮助他来理解各种技术上可能很深奥的内容。
我:
I forget to mention the context: I need to explain RLHF to my CEO during coffee time, and thus I don't think the above explanation is good enough. Several places need significant improvements. On the high-level, we should assume that he is non-teach with very limited tech background, that is, he for sure heard of terms like "machine learning", "training", "optimization", know their meanings, but not any algorithmic details like what is SGD. Therefore, the explanation should stay on a high-level, with daily life examples or analogies if needed.
Here are a list of noticed problems that you should improve:
- The focuses of your explanations are somewhat wrong. For example, why does our CEO need to know the detailed components of RL?
- Some contents are difficult for non-tech people, for example, you mentioned "models aligned with human values". What does it really mean? You may want to provide a concrete daily life example.
- the applications of RLHF is out of the scope, as my team works on foundation model training
- things that our CEO might be interested in like time, cost, technologies and infrastructure are completely not discussed by mistake.
Make sure you generate in plain text not markdown text.
ChatGPT:
Certainly, let's aim for a more casual yet informative discussion tailored for a non-technical audience.
Intro & Basic Idea
Reinforcement Learning with Human Feedback (RLHF) is like training a dog. Imagine you have a dog (your machine) that you want to teach tricks to (complete tasks). You could let the dog figure it out by trial and error, rewarding it with treats (this is the "Reinforcement Learning" part). But what if you could also guide it by saying "good dog" or "no, not like that" (this is the "Human Feedback" part). Over time, your dog learns faster and behaves more like you want it to.
So, RLHF is a way to teach computers to complete tasks while ensuring they behave in a manner consistent with what we want, all by using human guidance alongside the machine's own trial and error.
Why Is This Relevant?
Traditional reinforcement learning, like the initial treats-for-fetching scenario, can have gaps. The machine may not understand exactly what you want, and mistakes can be costly, especially if we're talking about a model that helps us make decisions in our business. Adding human feedback helps us fine-tune the machine's behavior, much like you corrected your dog's fetching habits.
How Complex Is This?
In terms of time, think of this as an ongoing relationship with your machine. Initially, it needs a lot of guidance, like a new employee or even a puppy. But over time, it should become more autonomous, requiring less of your input but still occasionally needing check-ins for complicated tasks.
From a cost perspective, the initial setup may require some investment to collect feedback and train the model. But the idea is that this should pay off by making the machine more efficient and less prone to expensive errors down the line.
What Technology Do We Need?
To make all this happen, we'd need to upgrade our existing infrastructure to support real-time data gathering and human feedback loops. This involves both hardware to run the models and software to collect and integrate human feedback seamlessly.
Any Risks or Challenges?
Sure, there are a few. One is that the quality of the model depends on the quality of the human feedback. Bad advice can lead to a poorly performing model. Additionally, the technology isn't "set it and forget it." It will require ongoing human involvement, although hopefully less and less over time.
---
So, in a nutshell, RLHF is like teaching your dog new tricks, but with the added benefit of ongoing guidance. It's a time and resource commitment, but the payoff could be a machine that not only does its job but understands the "why" behind it.
这下感觉如何了?那个chatgpt给的那个训狗的例子,是不是超级棒的?反正,我感觉比我解释的要好。真正做到了,用简明扼要的语言,解释了一个非常专业的知识,使得整个过程,如此的浅显易懂。嗯,至少写出来这个例子,chatgpt已经开始产出,对我来说有意义的内容了。
但是除了这个”What is RLHF“的开头之外,其他的内容一样的具体而空洞。比如,为什么老板要关心这个技术?说了一大堆貌似有点关系,但是又不那么直接的东西。
题外话1,如果你不懂什么是RLHF,那么看完这个,我觉得你可以出去说自己已经懂了。这么好的例子,值得翻一下
RLHF就像训练狗一样。想象一下,你有一只狗(你的机器),你想教它一些技巧(完成任务)。你可以让狗通过不断尝试和犯错来摸索,并给它食物作为奖励(这就是 "强化学习 "的一部分)。但如果您也能通过说 "好狗狗 "或 "不,不能这样 "来引导它呢(这是 "人反馈 "部分)。随着时间的推移,您的狗狗会学得更快,表现得更像您想要的样子。
因此,RLHF 是一种教计算机完成任务的方法,同时确保它们的行为符合我们的要求,所有这些都是通过人类的指导和机器自身的试错来实现的。
题外话2,我所见过的技术大拿们,不少都有着能把一个很困难的知识点用非常朴素平实,准确的来说,小学生都能听明白的话,把它解释清楚的能力。
我越来越感觉,这是往上混,尤其是和上层通道里面,那些不做技术的大领导们打交道,所必备的能力。因为大领导们,通常都是非常自信的。他们如果听不懂你在说什么,放心,他们一定不会觉得是自己的问题。相反,这一定是你这个人的问题。因为,没有对比,就没有伤害 —— 明明谁谁谁几句话,就能解释清楚的事情,到了你这里,啰里八嗦说了半天,整了一堆专业术语,根本也不知道你在说什么!!!
Round 3: chain-of-thoughts (CoT)改进
我们当然可以接着之前的那样Round2的改进,继续提意见,让chatgpt继续迭代。 但是,这里可以提供一个更加有效的迭代方式。这是完全从CoT里面来的。当然,实际的操作中,你可以混合上其他的技术,比如,我这里就显性的赞美了之前”What is RLHF“的工作。
我
Hmm. I guess we are now on the right path, but still have a long way to go. I believe you did an awesome job in explaining RLHF with the dog training example. I am sure that our CEO could grasp the core idea of RLHF easily. Thank you.
However, I believe we need a different strategy to prepare the talk. Let us do it step-by-step. First, I want you to pretend that you are a CEO with little tech background in a Global 100 tech company, and come up a list of things and questions that you might be interested in regarding RLHF from different aspects. Then, you help me prepare the content according to the list of CEO interested RLHF topics.
ChatGPT
Absolutely, let's break this down further. First, I'll list out the topics and questions that a CEO of a Global 100 tech company might have after hearing the initial RLHF concept explained through the dog-training analogy.
List of CEO Interested RLHF Topics
Business Strategy and Value Proposition
1. How does RLHF align with our company's current strategy and long-term vision?
2. What is the unique value proposition of RLHF for our specific business needs?
3. Is this a competitive advantage? If yes, how sustainable is it?
Financial Concerns
4. What is the ROI (Return on Investment) we can expect from implementing RLHF?
5. What's the initial cost for setting it up and the ongoing costs for maintaining it?
6. How does the cost and potential benefit compare to other solutions we are considering
Timeframe and Implementation
7. What's the estimated timeframe for implementation and seeing measurable results?
8. Do we have the necessary resources and skills in-house or do we need to hire?
Risks and Mitigation
9. What are the primary risks of adopting RLHF, and how can they be mitigated?
10. Are there any regulatory or compliance considerations we need to be aware of?
Technology and Infrastructure
11. What are the technological requirements for implementing RLHF?
12. Will our existing infrastructure support it, or do we need upgrades?
Stakeholder Alignment
13. How easily can this be explained to stakeholders and how will it affect customer/user experience?
14. What kind of internal training or change management is required?
Scalability and Future Proofing
15. How scalable is the RLHF approach as our business grows?
16. How adaptable is it to future technological changes or market needs?
--------
With these questions in mind, let's proceed to craft the content that will address these topics. Would you like to move ahead with that?
这下子是不是有点清楚了?有可能在解释完什么事RLHF之后,我还要干点啥?毕竟和RLHF的内容千千万,你要是不加限制,那么生成的结果,大概率和你真正想要的不一样。
以上的这么多条里面,只有一些是我觉得CEO可能会关心的点,其他的都不怎么合适。比如#4的投资回报率,这根本不是我的专业范围,我也给不了这样的回答。又比如#13 和 #14,也不是我的专业领域,另外,大概率正常CEO不会问一个技术专才这样的问题。
Round 4: 继续给直接的反馈,和帮助chatgpt更准确的了解我的意图和目的。
请记住,所有你觉得重要的事情,都显性的表达出来。别指望chatgpt会来猜你想干嘛?不是说他不会猜,而是说,既然可以直接说清楚明白,为什么要用低效的方法让它来猜。
比如,我就在这个地方,明确了,如何在CEO关心列表中,删选好的和坏的点。其中有再次强调的这个context的咖啡时间,并且是显性的告诉他,这意味着我没有很多时间,也做不了需要深入回答的问题。我还添加了,它之前没有提到的,关于我们竞争对手和产品的话题。
最后,我又提了个新的要求 —— “自己不想当工具人,就是给老板解释这个技术。我要夹带私货,我要展现给老板,我不光了解技术,而且我们团队还能……”。我甚至,变相承认自己很懒,告诉他,我需要他帮助有过度,来引导CEO询问我所希望他关心的点。而所有的这些,都是显性表达。
I need to remind you the context is that I, as a tech lead, was asked to explain RLHF to my CEO in a coffee time. Therefore, you should consider the implication of this context and remove those topics that are inappropriate, e.g.,
- I probably only have a few minutes to explain RLHF and related things, and thus anything requires a deep dive or may trigger a long list of new questions should be avoided.
- I am not a finance person, and I don't have any idea about things like ROI, and when we can expect returns.
In contrast, I think questions regarding our competitors makes sense. I would like to add questions about existing well-known products/models that using RLHF technologies.
Finally, I don't want to simply explain RLHF to my CEO, but take it as a great opportunity to show my expertise in the area and my team impacts on the RLHF relevant things. Therefore, we should somehow prepare the talk in such a way that we can guide the conversation towards to things that relevant more to my team.
ChatGPT:
Thank you for clarifying the context and your objectives for this coffee-time conversation with the CEO. Given the constraints, we should focus on explaining RLHF in a concise yet impactful way, emphasizing its relevance to the work your team is doing. Here's a trimmed list of topics that suit the scenario:
Streamlined CEO's List of Interests and Questions on RLHF:
1. (Strategic Importance) Why is RLHF a game-changer for foundational model training?
2. (Timeframe) How quickly can we see initial results using RLHF techniques?
3. (Human Resources) Does our team have the expertise to implement and fine-tune RLHF?
4. (Competitors) Are any of our competitors using RLHF?
5. (Market) Are there any well-known products or models that use RLHF technologies?
6. (Showcasing Team's Impact) How has our team already impacted RLHF implementation or research?
---
Prepared Talk Points
Intro:
"After that dog-training example, you're probably wondering how RLHF fits into the larger picture, especially for our team, right? Let me explain."
Strategic Importance
"Think of RLHF as the future of machine learning, but more user-friendly. It's like giving our technology an intuitive 'sense' about what the end-user actually wants. For foundational models, this could be groundbreaking. Imagine a model that evolves based on both data and real human needs."
Timeframe
"We can get preliminary results in a matter of weeks. But remember, like training a dog, it gets better over time. The more human feedback we have, the smarter the model becomes.
Human Resources
"We've got some of the brightest minds in the field. And, with a little more focus, our team could be the one pushing the envelope in RLHF."
Competitors and Market
"Now, we're not the only players eyeing this. Big names like Google and Facebook are already exploring RLHF. And you've heard of GPT-3, right? They're using similar technologies. But what they lack is our specialized focus on foundational models."
Showcasing Team's Impact
"Our team has already made strides in this area, from publishing research to optimizing existing algorithms. We have the potential to not just follow in the footsteps of RLHF innovation but to actually lead the way."
Closing
"So, in a nutshell, RLHF isn't just a trend; it's the next big thing. And we have the expertise and the vision to be at the forefront. How does that sound for a future roadmap?"
---
With this prepared talk, you'll be able to convey the essence of RLHF, why it matters, and, importantly, how your team is pivotal in this space—all within a short coffee break conversation.
Wow,这下感觉如何了?是不是有那么点意思了?你看它还贴心的教我进行自说自话的过度
Intro: "After that dog-training example, you're probably wondering how RLHF fits into the larger picture, especially for our team, right? Let me explain."
这下,是不是觉得,可以拿它来作为跟老板闲扯提纲了?觉不觉得,我真能抓住这么一个机会,在自己大老板面前,留个好印象了?
我觉得是可以了。该有的,大体上,都有了。那对比前后两次回答的不同,到底哪里改进了呢?主要就是在,如今的回答,都是开始以“我”和“我的团队”为中心了,并且涉及的也是更多在技术这个层面,而不再是之前的没啥营养的似乎哪里都能扯上一嘴的高谈阔论了。
那为什么这就是个更好的回答了呢?一方面是因为,CEO不需要我教他做人,他要找我聊聊,也是聊我专业的领域,他要关心其他的,自然会去找别人去聊。另外一方面是,我可以在自己的领域里面尽情发挥,怎么聊也不会陷于一个空洞无物的状态。而CEO找我,又怎么会期待我跟他说一堆没有营养的废话呢?而聊一个自己不了解的领域呢?聊的没深度是肯定的。
当然,也还是可以有改进的地方。所以,我们再次迭代一波。
round 5: 继续细化自己的要求
继承我要摸鱼的心,我连老板可能要问的问题和答案,都要chatgpt给我准备好。另外呢,我觉得还要加强下,我在老板面前的印象,把他的问题,和我们现在的项目结合下。
我:
I like the prepared talk points in general. Two more requests: 1. for each talk point, please again pretend that you are the CEO and ask up to three follow up questions. List me these questions, and prepare answers on behalf of me. 2. our team is now working on vision transformer modeling, which is not trained in RL at all. Can you somehow connect RLHF with it? For example, explain what if we train it with RLHF? Again, don't be too technical.
chatGPT:
Absolutely, let's proceed with your additional requests.
---
Follow-Up Questions from the CEO and Prepared Answers:
On Strategic Importance
CEO's Question 1: "How exactly would incorporating human feedback make our foundational models more user-friendly?"
Your Answer: "It makes the models more aligned with what people actually want. Instead of just analyzing data, these models also learn from human guidance. Imagine a GPS that learns to avoid routes with too many stoplights because it knows you dislike them, not just because the data says it's faster."
CEO's Question 2:"Is RLHF technology mature enough for us to implement now, or is it still experimental?"
Your Answer: "It's an evolving field but far from experimental. Major tech companies are already investing in it. The technology is ripe for us to both adopt and innovate."
On Timeframe
CEO's Question: "Can you provide any measurable milestones for how we'll know it's improving?"
Your Answer: “Certainly, we can track how often the model makes recommendations or decisions that align with our objectives or user preferences. If those numbers go up, we know the model is getting better."
On Human Resources
CEO's Question: "Do we need to bring in external experts, or can our current team handle it?"
Your Answer: "Our team is highly skilled and can definitely take this on. With some targeted training, we can become self-sufficient in RLHF."
On Competitors and Market
CEO's Question: "How long before our competitors catch up to us if we start implementing RLHF now?"
Your Answer: "That's hard to predict, but implementing RLHF would certainly give us a competitive edge. Our specialized focus on foundational models could make us market leaders before others even enter the race."
CEO's Question 2: "Do we have examples of well-known products or models using RLHF that I can refer to when discussing this with stakeholders?"
Your Answer: "Yes, OpenAI's GPT-3 has elements of RLHF, and it's one of the most talked-about AI models today. It's a concrete example stakeholders can relate to."
On Showcasing Team's Impact
CEO's Question: "What exactly has our team already achieved in this area?"
Your Answer: "We've published research papers that are gaining attention in the academic community and we've successfully optimized some existing algorithms to work better with real-world data. So, we've already laid the groundwork."
Connecting RLHF with Vision Transformer Modeling:
”In fact, speaking of foundational models, our team's current work on Vision Transformer models could be significantly augmented by RLHF. Imagine a Vision Transformer model trained to recognize and interpret images, but now, it can also learn from photographers, designers, or everyday users on what makes an image 'good' or 'important.' It would not just be recognizing objects but understanding the human aesthetic or safety considerations behind images. That opens up a whole new avenue for applications, from smarter photo editing software to more nuanced surveillance systems. All without changing our core expertise in vision models."
---
With these added elements, you'll be well-prepared to guide the conversation, address potential follow-up questions, and spotlight your team's impact and potential in a concise, impactful way.
这下,反正我看着是可以了。基本就可以照着准备去了。即便不中,也不远矣。聊天的时候,照着引导下,这里面的问题和回答,我不用都用上。能压中一两个就好,因为我自己有信心可以根据这个,接着聊下去。
round 6: 结束轮,我不想上下翻页去找相关内容copy-paste,直接给个需求,让chatgpt全部清理干净。
我:
awesome, please put everything together and make the content consistent, including the introduction of RLHF with the dog training example, the prepared talk points, the vision transformer connection, and the list of Q&A. I need to print it and do some preparation.
chatgpt的回答,就是合并起来的那些东西,我就不贴了。你们自己脑补吧。
回顾整个过程,你们可以看到,这是一个我和chatgpt,彼此迭代的过程。
虽然,大的设定,我在round1开始就已经做出来了。但是很显然,就和其他的很多时候,很多任务一样,在最开始的时候,你是很难很完备的,把各种需求都说清楚的。事实上,你可以说,我是两眼一抹黑的就开始给chatgpt发任务了。
于是乎,第一轮对话,基本就是个废物。虽然,他不能用,但是他给我指出了,自己要求里面的潜在问题。
题外话:chatGPT特别擅长写各种内容看上去丰富,但是实质空乏的,各种废话和套话。主打的就是,虚心接受,前改后忘……,可你还生不了气。
我在第二轮的时候,进行了修改,明确了哪些是合适的内容,哪些是不合适的。尤其强调了两点在背景上的内容,第一,我是在一个非正式的咖啡时间;第二,我的对象不懂技术,所以别用很专业的词汇和句子来解释这个RLHF技术。这是一种提供ChatGPT进行"in-context learning"的方式。我更是明确的,要求它以日常生活中的例子,或者类比,深入浅出的解释这个RLHF。
在这一轮之后,chatgpt产生了第一个有用的输出 —— 如何用训狗来做类比,使得一个没有技术背景的CEO,也能明白这个RLHF技术是个什么东西,它又是怎么样来训练我们机器的。可以说,chatgpt成功的做到了,深入浅出的解释了RL和HF。
但是,除此以外,其他的内容,还是依然非常的空洞 —— 解释为什么他是和我们有关的,他到底有多复杂,有些什么具体的困难……
这个时候,也就是第三轮对话,我也开始第二次迭代,我意识到自己的提问方式有问题。直接让chatgpt扮演我来写怎么给CEO解释RLHF,是可以有千千万万种写法的。因为每次要是提出来不同的意见,他就是会去修改 —— 但是,我能提出来什么意见呢?在这个时间点上,我也和大多数人一样,其实不知道要写点啥。好吧,我承认,我还是知道的更多一点点。但是这里个关键是,如果你给chatgpt的反馈,只是你觉得什么好,什么不好,你大概需要很多轮,才能迭代出个像样的答案。并且如果你不做好正确的反馈的话,很有可能出现前面犯过的错,后面接着来的情况。
那我干了什么呢?我做了两件事情。第一,我给了明确的正反馈,高度评价了训狗的那个例子。我要让他记住这个好的点,以期望他能继续这样发挥。第二,我使用了CoT技术,帮助他分解了任务,让他通过逐步完成具体的小任务的方式,来最后解决更加困难的大任务。后者,是我调教成功的关键之一。我让chatgpt,不再来扮演我,而是去扮演我老板,看看有什么是从老板的角度,比较关心的点先。
题外话,chatgpt在帮助我跳出自己的思维定势,更好了解自己的讨论对象和受众,有着极其显著的优势。因为我们都是不同背景的人,很多时候,我感兴趣的,其实并不是别人感兴趣的。而以自己的背景,很多时候并不能真正的跳到别人的鞋子里面去考虑他的问题。但是,在这一点上,chatgpt做的很好。
这就到了第四轮了。Chatgpt果然就给了一系列的CEO关注的和RLHF相关的不同的点。粗粗看了下,还是大量没用的内容,有用的内容有,但是不多。另外,大概因为我让他扮演CEO提问的原因,它又忘记了这个任务的大的context —— 我(作为tech lead)要给(不怎么懂技术的)CEO,在咖啡时间讲解RLHF。
于是乎,我再次强调了以上的内容,并且明确了要根据以上的场合删减它所给出来的CEO所关心内容。同时,我也提出了一个之前没有提的要求 —— 帮我抓住机会,展现自己的能力,而不仅仅是解释清楚RLHF就行了。
好了,这下ChatGPT就明确了,它回应我说
Thank you for clarifying the context and your objectives for this coffee-time conversation with the CEO. Given the constraints, we should focus on explaining RLHF in a concise yet impactful way, emphasizing its relevance to the work your team is doing.
我看了下,把我前后说的,合并的不错。我再次确认了这个回应的正确性。由此,就进入CoT的第二个环节了。这回ChatGPT回应了我的要求,选择了更加贴近我工作范围的,并且CEO也会感兴趣的话题,来准备talking points。
Thank you for clarifying the context and your objectives for this coffee-time conversation with the CEO. Given the constraints, we should focus on explaining RLHF in a concise yet impactful way, emphasizing its relevance to the work your team is doing. Here's a trimmed list of topics that suit the scenario:
Streamlined CEO's List of Interests and Questions on RLHF:
1. (Strategic Importance) Why is RLHF a game-changer for foundational model training?
2. (Timeframe) How quickly can we see initial results using RLHF techniques?
3. (Human Resources) Does our team have the expertise to implement and fine-tune RLHF?
4. (Competitors) Are any of our competitors using RLHF?
5. (Market) Are there any well-known products or models that use RLHF technologies?
6. (Showcasing Team's Impact) How has our team already impacted RLHF implementation or research?
其实到了这里,大体上就已经满足了我的要求了。剩下的都是让我能更省力的需求了。
我在第五轮,再次要求它扮演老板,根据之前的talking points,接着准备提问,然后准备好参考答案。最后,更是让他给我找一个更加贴近我们组现在工作的例子,展现我在专业领域的信手拈来。
这里面没有用到的是RAG技术,因为我现在这个任务不需要那么专业的内容。但是除此以外,基本跟我用chatgpt写论文,也没有什么区别了 —— 在一轮轮的对话中,我在不停的细化自己的需求和限制。
从这个角度说,如果你不知道怎么更加明确的提出自己的需求,了解自己的限制,给不了有针对性的评价,是很难用好chatgpt的。
当然,从优化的角度说,你的每一轮对话,理想情况下,是想不停的通过提供更加明确的信息和知道,使得解空间不断的缩小。这个过程越快,越准确,也就意味着,你能用越少的轮数,得到越满意的内容。
最后来聊下怎么才能用好chatgpt来写论文。
我不提倡,你在什么都不知道的情况下,让chatgpt自由发挥。因为如你们所见,你要不给出来正确的方向,它能自由发挥的余地太大了,而且很可能是一堆英语连贯,逻辑严谨,但是空洞无物的废话。
我一般都会自己写一个初稿。哪怕是论文要8页,我自己只写出来2页。至少我要把这个思路给理顺了。不然的话,用chatgpt是不会顺手的。注意,这是初稿,不是提纲。单单只有提纲,chatgpt一样也可能走的很偏。
然后,我就会一点点往这里面用chatgpt一点点的填东西。先写非常确定的内容,比如自己的方法,自己的实验,自己的结果。最后才是,引言,摘要,结论,还有题目。
使用的过程,一般我都会具体的任务化 —— 你们可以想象成是那种大实验室里面,几个人一起合作写paper的情况。每个人的写作内容,都可以看成是一个独立的任务。这样子能最大程度简化chatgpt处理任务的难度。最后把每个处理好的任务,放在一起,当成一个新的任务,让chatgpt总揽全局似的修改,才能做到更好的前后呼应。
当然,在这里面,你还是要会提问题,给context,尤其是chatgpt在废话连篇的时候。要能一步步帮它理清自己的目标。如果token不够,那就把简单的任务丢给3.5去做,只在4上面做关键的任务。
另外有一些小tip,是我经常使用的,用来在成文之后,继续打磨文章的。
- 让chatgpt扮演一个只有1分钟时间阅读的这个章节的reviewer,让chatgpt指出来,哪里不够浅显易懂,或者有没有get到我们的重点
- 给另一篇早先文章的方法和他们的背景内容,让chatgpt扮演这篇文章的作者,来评价我们的工作,尤其是,我们到底有没有说清楚了,和这篇文章的异同,我们highlight出来的贡献和novelty是不是足够明显的区别他们
- 让chatgpt扮演一个仔细的reviewer,来看我们的文章,有没有什么前面承诺了,但是后面没有cover的优点,贡献,等等。
更多回答
今天分享给大家21个能帮助你润色文章的GPT指令,按大学写作流程顺序整理的,让你的文章更加有逻辑且通顺,希望他们能让你的文章更出色,助你顺利毕业~
一、文章写作润色指令
1、写作选题指令
① 确定研究对象
我是一名【XXXXX】,请从以下素材内容中,结合【XXXXX】相关知识,提炼出可供参考的学术概念。以下是结合素材内容,提炼出的几个可供参考的学术概念:
【概念 a】、【概念 b】、【概念 c】、【概念 d】
② 确定研究选题
我是一名【XXX】学生,我的专业是【XXXXX】,我的研究方向是【XXXXX】,你将扮演我的导师角色。我目前正在准备撰写一篇学术论文,需要你的指导。我对【XXXXX】技术领域感兴趣,但是我还没有形成具体、明确的研究问题我注意到有研究学者会对【XXXXX】进行探究。我想从这种技术入手来挖掘研究问题。请帮我推荐 10 个参考选题。
2、写作大纲指令
我想写一篇关于【XXX主题】的:
我的选题方向是【XXXXX方向】你能帮我拟定一份论文大纲吗?请包括以下几个部分:标题、摘要、引言、相关工作、方法、实验、结果、讨论、结论和参考文献。
3、研究理论指令
我想在我的论文中使用一些研究理论来支持我的观点。
你能给我推荐些关于【XXX主题】的研究理论吗?请给出理论的名称、作者、出处和主要观点。
4、参考文献指令
我想在我的论文中引用一些文献来支持我的论点和方法。
你能给我推荐一些关于【XXX主题】的文献吗?请给出文献的标题、作者、年份、摘要和关键词。
5、文献综述指令
我想请你帮我写一份关于【XXX主题】的文献综述我的论文的选题方向是【XXXXX方向】我已经找到了以下几篇文献
(文献 1的标题,作者年份,摘要和关键词)
(文献 2的标题,作者年份,摘要和关键词)
(文献 n的标题,作者年份,摘要和关键词)
你能根据这些文献,写一份大约【XXX字数】字的文献综述吗?请按照以下的结构组织你的内容:
引言:介绍主题的背景、意义、目的和范围
主体:按照主题、方法或观点等分类方式,对文献进行梳理分析和评价
结论:总结文献综述的主要发现贡献和不足。
6、写作致谢指令
我想请你帮我写一份关于我的论文的致谢。
我的论文的题目是【XXXXX题目】,我的导师是【XXX导师】。我的合作者是【XXX合作者】,我想感谢以下的人或机构:
(感谢对象 1):感谢他们对我的(帮助或贡献)
(感谢对象 2):感谢他们对我的(帮助或贡献)
(感谢对象 n):感谢他们对我的(帮助或贡献)
你能根据这些信息,写一份大约【XXX字数】字的致谢吗?请使用礼貌和诚恳的语气并注意格式和标点。
7、润色优化指令
① 精简文章内容:
输入“删除不必要的内容”,可以去除文章中的多余内容,使得文章更加简洁。
例如:文章中有些内容与主题无关,删除这些内容可以使得文章更加清晰。
② 提高段落之间的连贯性:
输入“加强段落之间的过渡”,可以通过增加过渡句或调整段落顺序来提高文章的流畅度。
例如:段落之间的跳转让文章读起来有些断层加强段落之间的过渡可以帮助读者更好地把握文章的脉络。
③ 矫正错别字和语法错误:
输入“修正拼写和语法错误”,可以发现文章中的拼写错误和语法错误,并给出修改建议。
例如:文章中可能有一些明显的拼写错误或语法错误,使用纠错功能可以快速发现并修改这些错误。
④ 改善段落结构逻辑:
输入“优化段落结构”,可以检测段落缺少连贯性的地方,并给出建议来强化段落逻辑。
例如:某些段落可能缺少一些逻辑上的联系,通过优化段落结构可以使文章更加条理清晰。
⑤ 替换过时用法:
输入“替换过时的词汇或短语”,可以使用更现代的用法或词汇来替换过时的短语。
例如:文章中使用了一些过时的词汇或短语,通过使用更常用的同义词或现代的短语可以使文章更加时尚。
⑥ 增加详细信息:
输入“增加更多的细节和具体内容”,可以增加更多的具体例子或数据来使文章更加生动、有趣。
例如:文章中可能缺少必要的细节,通过增加更多的例子或数据可以让文章更加详实。
⑦ 澄清表达含义:
输入“澄清表达意思”,可以使用更加明确的语言来澄清文章中含义模糊的地方。
例如:某些表达方式可能会造成混淆,使用更加明确的语言可以让文章更加易于理解。
⑧ 更改字母大小写规范:
输入“调整字母大小写规范”,可以检测不正确或不统一的大小写,并给出调整建议。
例如:标题中的每个单词的首字母应该大写,保持标题中大小写规范的统一性。
⑨ 提高段落可读性:
输入“提高段落可读性”,根据段落内容和特点,在段落结构、句子长度和行文风格等方面给出调整建议。
例如:某些段落长度过长,建议使用更短的句子简化段落,使用清晰的措辞和避免余,则段落读起来更加流畅。
⑩ 更换垃圾词:
输入“替换文章中的垃圾词语”,ChatGPT 会识别和提供一些不太专业的短语和词汇的更佳替代方案。
例如:将一些口语化或者过于简单的单词或短语替换成更加正式或专业的词汇,可以使文章更加严谨和专业。
⑪ 使用严谨的学术逻辑和语言对文字进行润色:
这意味着你需要确保你的论文或文章遵循了一种清晰、连贯的逻辑结构,每个观点都有充分的证据支持。同时,你需要使用专业、准确的学术语言,避免使用口语或非正式的表达方式。
⑫ 优化段落结构,确保段落之间的可读性:
这意味着你需要确保每个段落都有一个明确的主题句,然后是支持这个主题的详细句子。同时,你需要确保段落之间有适当的过渡,使得读者可以顺畅地从一个段落过渡到下一个段落,而不会感到突兀或混乱。
⑬ 增加更多细节和具体内容:
这意味着你需要在你的论文或文章中提供更多的具体例子、数据或引证,以支持你的观点。这可以帮助读者更好地理解你的观点,并增加你的论文的说服力。
⑭ 进行同义词的替换:
这意味着你可以使用不同的词语来表达相同的意思,以增加你的论文的语言多样性和丰富性。但是,你需要确保替换的词语在语境中的含义是准确的,否则可能会导致误解。
⑮ 修订文字中的错别字和语法错误:
这意味着你需要仔细检查你的论文,找出并修正所有的拼写错误和语法错误。这是一个非常重要的步骤,因为错别字和语法错误会影响你的论文的专业性和可读性。
二、精选AI工具分享:
① Chat AI: aichat.com 通用ChatGPT,支持3.5和4.0、最新模型GPT-4.0 Turbo
② ChatGPT:chat.openai.com 目前一枝独秀,独步天下
③ Chat Plus:chat.aiplus.vip ChatGPT加强版,支持3.5和4.0、最新模型GPT-4.0 Vision、AI画图、AI读图、插件功能
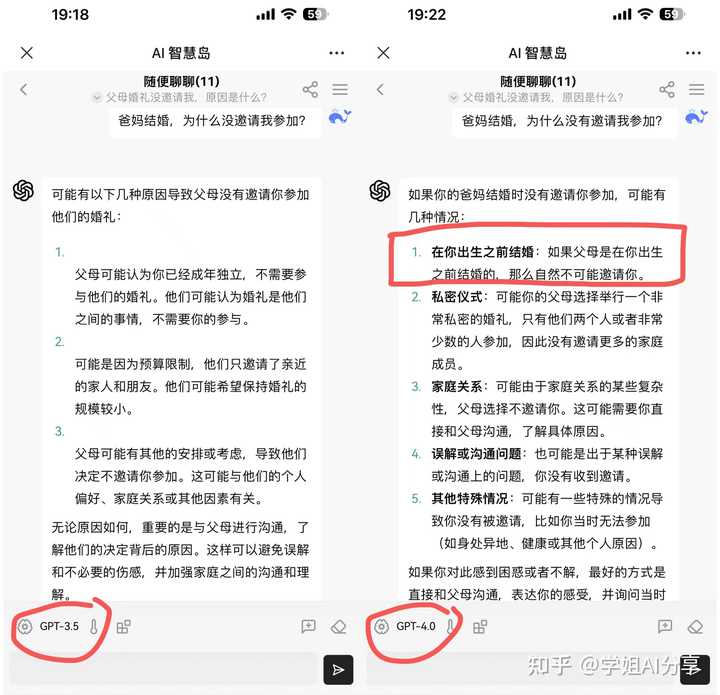
④ NewBing:bing.com/new 被驯化过的ChatGPT内核
⑤ 谷歌巴德:bard.google.com 世界第一搜索引擎硬刚ChatGPT的第一把斧头
⑥ 百度文心一言:文心一言 国产聊天AI第一杆大旗
⑦ 阿里通义千问:通义大模型 大佬马云给出阿里的「答案」
⑧ Notion Al:notion.ai 堪称目前最好的文档类工具,没有之一
⑨ Copy.ai:copy.ai 营销软文,自媒体稿件小助手
⑩ Chat File: chatfile 支持pdf、word、excel、csv、markdown、txt、ppt等文件,所有格式简直通吃
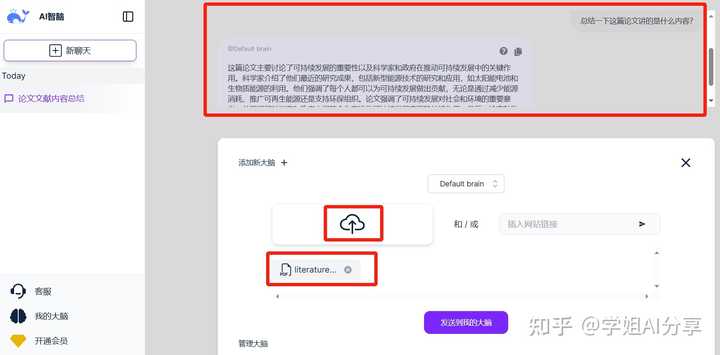
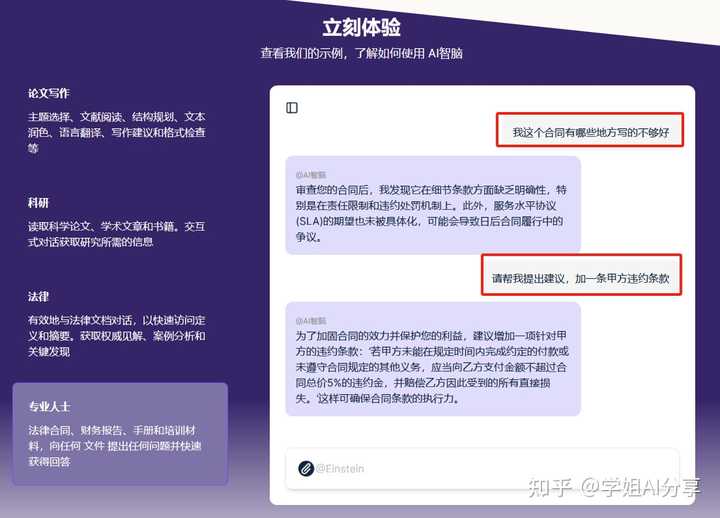
⑪ AskYouPDF: chatPdf 释放PDF的力量!深入你的文档,找到答案,并将信息带到你的指尖。
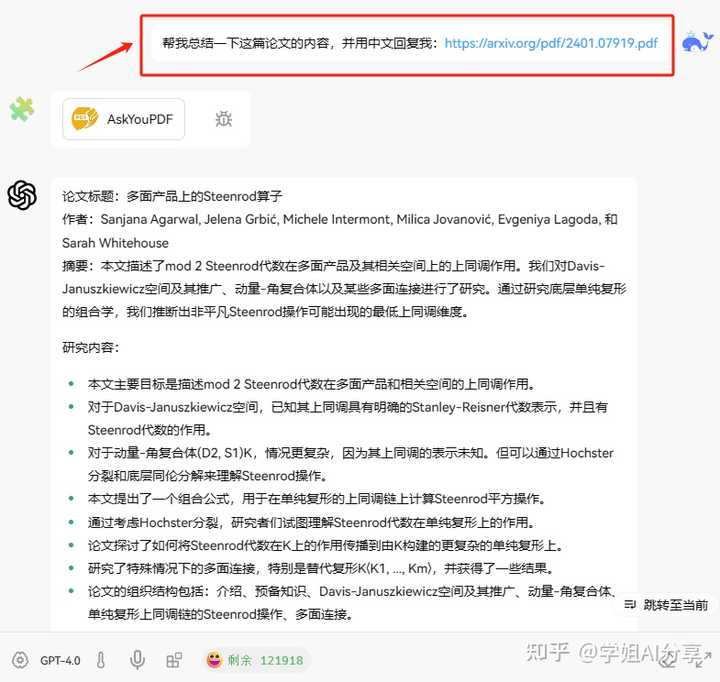
⑫ Chat Excel: ChatExcel 与excel聊天,支持excel计算,排序等
⑬ Chat XMind: chatMind 通过聊天创建和修改思维导图
我整理了很多干货, 可关注
领取有想一起学习、使用、分享的~ 欢迎加入~
如果看完文章有收获,请帮忙【点赞】支持下~
The final thing to avoid is relying on ChatGPT to create content that includes references!
这是前段时间有个培训会上给我们反复强调的一句话,因为这不是危言耸听,而是很多学生经常犯的问题。
用ChatGPT来辅助(划重点)写论文是不可避免的,不可能防得住,因为论文就是文字的组合,不管是中文,还是英文,本质上就是语言对于脑子里面的想法的映射,在物理世界中就是文字的组合,Hello world不管是一个人手打键盘敲出来,还是一只猴子敲出来,抑或是ChatGPT生成出来,它本质上代表的内容是没有丝毫的差别的。
那么对于ChatGPT生成出来的文字,你顶多能隐约的觉得它的写作手法有点儿非人类,但是实际表达的意思还是人想要传达出来的。
但是有一点儿要注意,绝对不能用ChatGPT这类型的大模型,那就是参考文献References。
原因很简单,那就是ChatGPT是一个模型,它的作用是根据你给他提供的内容,尽自己最大的可能生成合适的回答。
而如果你要参考文献的话,你可能会得到两种答案:
第一种就是真实的参考文献。
对于ChatGPT来说,一般它会给你这样的回答。当你发现这样的“”的时候,基本上可以认为是真实的参考文献。
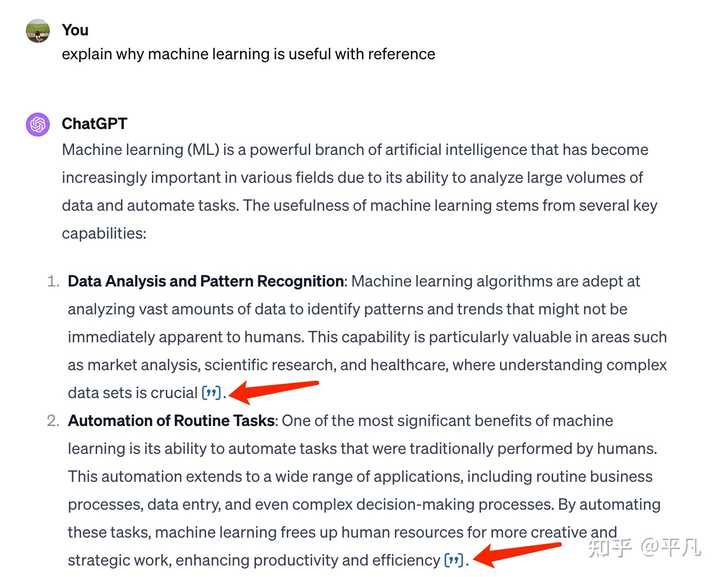
比如我们来点击第一个“,它出现了这样的一个链接。
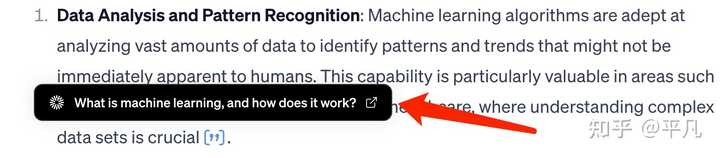
参考的是这个网站的这篇文章的这段话。
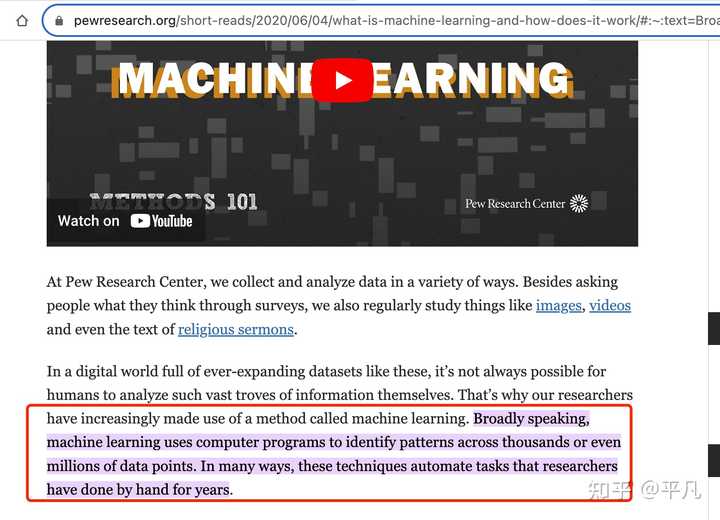
这种就是真实的参考文献,它也有可能给你期刊论文,也有可能是网站的博客等等,但起码是可以保持真实的。
如果你想要真实的链接,你可以尝试这样问ChatGPT,但是不保证每次都奏效。
explain why machine learning is useful with reference
重要的是加上「with reference」。
第二种情况是最差的情况,生成了假的参考文献
这种是最不容易发现,但是最最最危险的情况。
我让ChatGPT生成了一篇论文的大概,并提供了bib格式的参考文献。
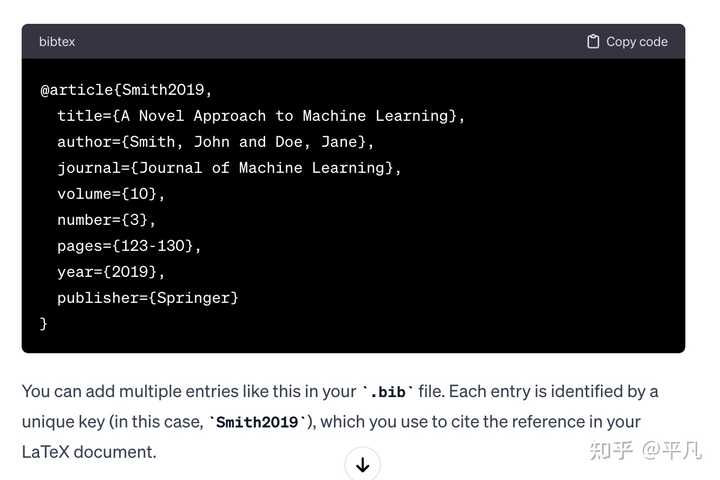
说实话,你能一眼看出来这个参考文献是假的吗?
我自己反正是没看出来,除非你去查。
首先,这个期刊是存在的,出版社也对的上。
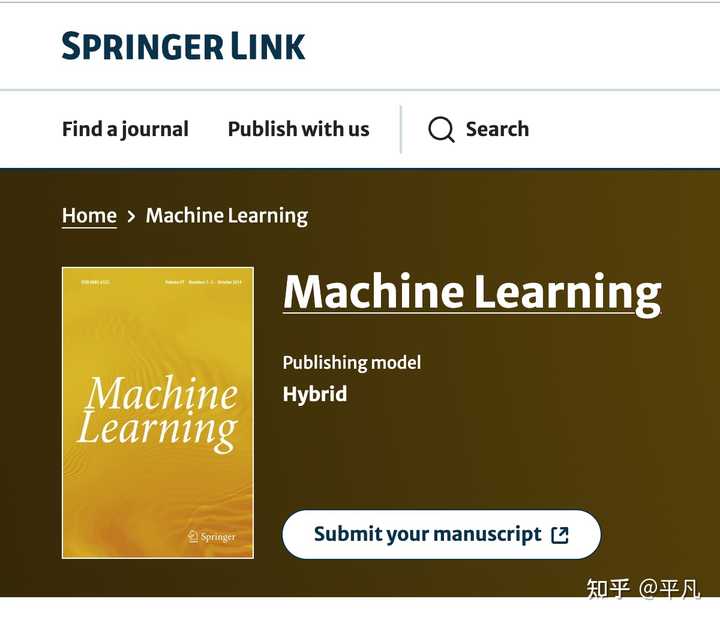
但是这篇文章根本找不到。
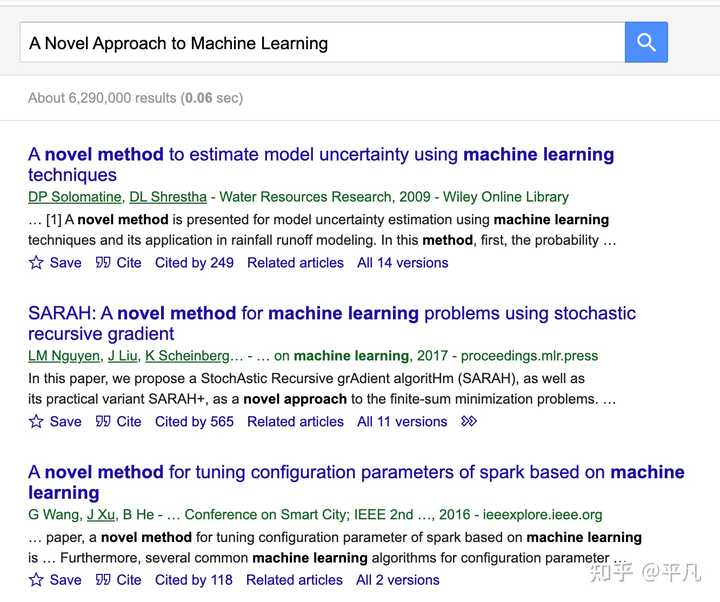
这就是ChatGPT在完完全全的给你编造了一篇参考文献,并且看起来几乎没问题,这就是问题的所在。
因为论文写的不好,这是水平的问题,但是你要用了假的参考文献,那性质就变成「学术不端」了,轻则警告,重则退学。
所以,千万千万千万不要再用GPT提供的参考文献。
GPT最好用的方式是来辅助你写论文,而不是直接用来生成。
AI是跟学术和工作方面的结合可以是很多方面的,如果你还不了解怎么跟学习工作结合,我建议你先花2个小时去看一下AI智能办公课,这门课程正是针对当下最火的AI大模型工具推出的,主打如何利用AI提高办公场景下的工作效率。内容覆盖了新媒体创作、内容整理、高效阅读、图片/视频制作等9大办公场景,特别适合IT互联网、数据分析处理、文字编辑/撰写等相关工作的职场人。课程是由业内大佬主讲的,所以干货含量不用担心。
官方入口我放在下面了 ⬇️ 感兴趣的可以报名听一下

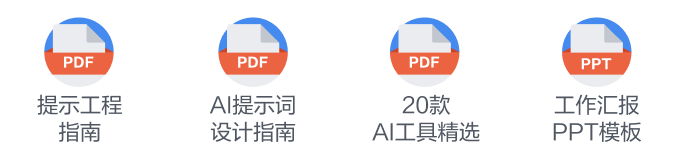
完课后还有几个非常有用的文件,提示工程指南,提示词设计等,目的就是不仅教会你AI可以做什么,还教给你怎么更高效的用Prompt跟AI沟通。
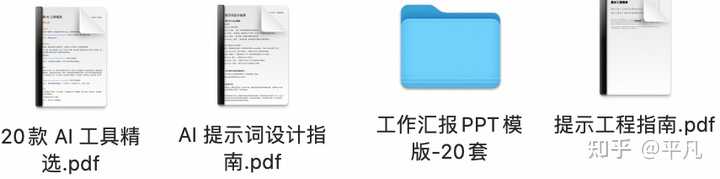
比如我这篇文章提供的几个非常实用的Prompt。
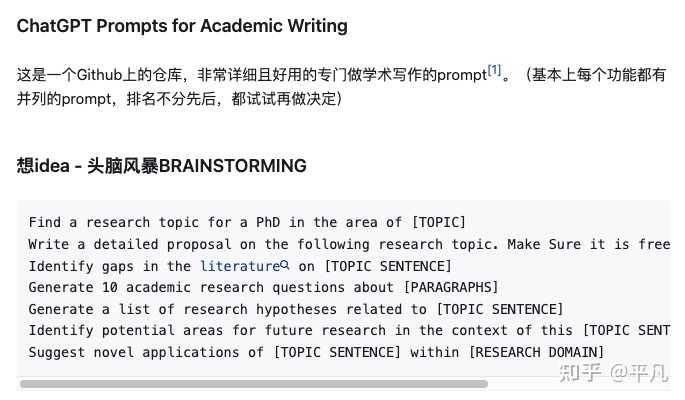
另外,参考文献其实也没那么难找,比如你可以用Elicit这个工具。
比如你拿到图二的这段话,不知道哪里去找参考文献,你可以这样。
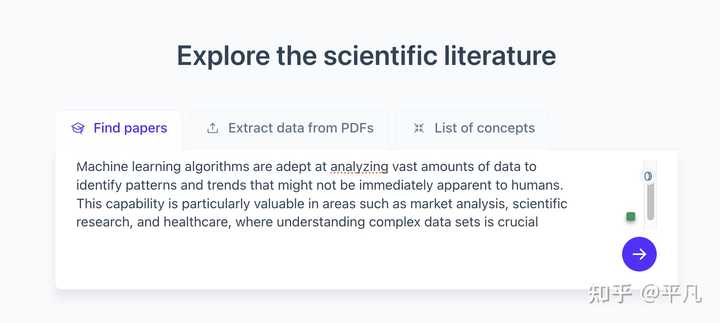
它会自动的根据你提供的内容找最相关的参考文献。
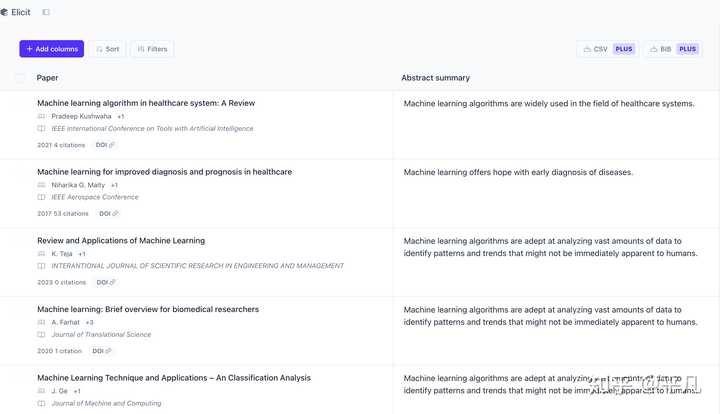
比如第一篇,这个从官网提供的参考文献就可以保证绝对是真的了。
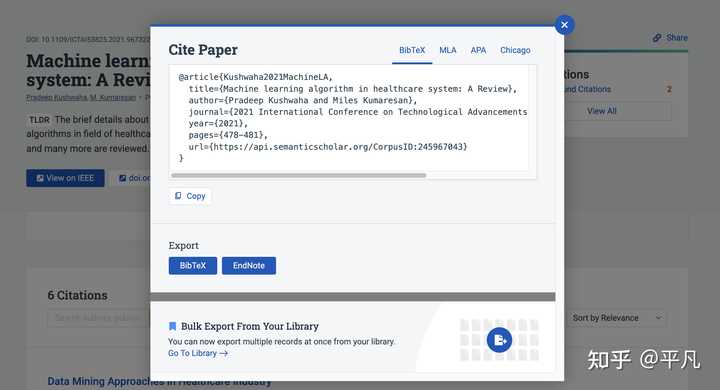