Popular information 流行信息
Popular science background:
科普背景:
They have revealed proteins’ secrets through computing and artificial intelligence (pdf)
他们通过计算和人工智能揭示了蛋白质的秘密(pdf)
Populärvetenskaplig information:
大众科学信息:
De har avslöjat proteinernas hemligheter med hjälp av datorer och artificiell intelligens (pdf)
他们利用计算机和人工智能揭示了蛋白质的秘密(pdf)
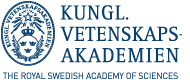
The Nobel Prize in Chemistry 2024
2024 年诺贝尔化学奖
Chemists have long dreamed of fully understanding and mastering the chemical tools of life – proteins. This dream is now within reach. Demis Hassabis and John Jumper have successfully utilised artificial intelligence to predict the structure of almost all known proteins. David Baker has learned how to master life’s building blocks and create entirely new proteins. The potential of their discoveries is enormous.
化学家们长期以来一直梦想着全面理解和掌握生命的化学工具——蛋白质。这个梦想现在触手可及。德米斯·哈萨比斯和约翰·贾姆珀成功地利用人工智能预测了几乎所有已知蛋白质的结构。大卫·贝克学会了如何掌握生命的基本构件并创造全新的蛋白质。他们发现的潜力是巨大的。
They have revealed proteins’ secrets through computing and artificial intelligence
他们通过计算和人工智能揭示了蛋白质的秘密
How is the exuberant chemistry of life possible? The answer to this question is the existence of proteins, which can be described as brilliant chemical tools. They are generally built from 20 amino acids that can be combined in endless ways. Using the information stored in DNA as a blueprint, the amino acids are linked together in our cells to form long strings.
生命的丰富化学是如何可能的?这个问题的答案是蛋白质的存在,它们可以被描述为出色的化学工具。蛋白质通常由 20 种氨基酸构成,这些氨基酸可以以无尽的方式组合。利用储存在 DNA 中的信息作为蓝图,氨基酸在我们的细胞中连接在一起,形成长链。
Then the magic of proteins happens: the string of amino acids twists and folds into a distinct – sometimes unique – three-dimensional structure (Figure 1). This structure is what gives proteins their function. Some become chemical building blocks that can create muscles, horns or feathers, while others may become hormones or antibodies. Many of them form enzymes, which drive life’s chemical reactions with astounding precision. The proteins that sit on the surfaces of cells are also important, and function as communication channels between the cell and its surroundings.
然后,蛋白质的魔力发生了:氨基酸的链条扭曲并折叠成一个独特的——有时是独一无二的——三维结构(图 1)。这个结构赋予了蛋白质其功能。有些蛋白质成为可以构建肌肉、角或羽毛的化学基块,而其他的可能成为激素或抗体。它们中的许多形成酶,以惊人的精确度驱动生命的化学反应。位于细胞表面的蛋白质也很重要,作为细胞与其周围环境之间的沟通渠道。

© 约翰·雅恩斯塔德/瑞典皇家科学院
It is hardly possible to overstate the potential encompassed by life’s chemical building blocks, these 20 amino acids. The Nobel Prize in Chemistry 2024 is about understanding and mastering them at an entirely new level. One half of the prize goes to Demis Hassabis and John Jumper, who have utilised artificial intelligence to successfully solve a problem that chemists wrestled with for over 50 years: predicting the three-dimensional structure of a protein from a sequence of amino acids. This has allowed them to predict the structure of almost all 200 million known proteins. The other half of the prize is awarded to David Baker. He has developed computerised methods for achieving what many people believed was impossible: creating proteins that did not previously exist and which, in many cases, have entirely new functions.
生命的化学基石——这 20 种氨基酸所蕴含的潜力几乎无法夸大。2024 年诺贝尔化学奖旨在理解和掌握它们,达到全新的水平。奖项的一半授予德米斯·哈萨比斯和约翰·贾姆珀,他们利用人工智能成功解决了化学家们苦苦挣扎了 50 多年的问题:从氨基酸序列预测蛋白质的三维结构。这使他们能够预测几乎所有 2 亿已知蛋白质的结构。奖项的另一半授予大卫·贝克。他开发了计算机化的方法,实现了许多人认为不可能的事情:创造以前不存在的蛋白质,并且在许多情况下,这些蛋白质具有全新的功能。
The Nobel Prize in Chemistry 2024 recognises two different discoveries but, as you will see, they are closely linked. To understand the challenges this year’s laureates have overcome, we must look back to the dawn of modern biochemistry.
2024 年诺贝尔化学奖表彰了两项不同的发现,但正如您将看到的,它们密切相关。要理解今年获奖者所克服的挑战,我们必须回顾现代生物化学的黎明。
The first grainy pictures of proteins
蛋白质的第一张颗粒状图片
Chemists have known since the nineteenth century that proteins are important for life’s processes, but it took until the 1950s for chemical tools to be precise enough for researchers to start exploring proteins in more detail. Cambridge researchers John Kendrew and Max Perutz made a groundbreaking discovery when, at the end of the decade, they successfully used a method called X-ray crystallography to present the first three-dimensional models of proteins. In recognition of this discovery, they were awarded the Nobel Prize in Chemistry in 1962.
化学家自十九世纪以来就知道蛋白质对生命过程的重要性,但直到 1950 年代,化学工具的精确度才足够高,使研究人员能够开始更详细地探索蛋白质。剑桥大学的研究人员约翰·肯德鲁和马克斯·佩鲁茨在十年末期取得了突破性发现,他们成功地使用一种称为 X 射线晶体学的方法,展示了蛋白质的第一个三维模型。为了表彰这一发现,他们于 1962 年获得了诺贝尔化学奖。
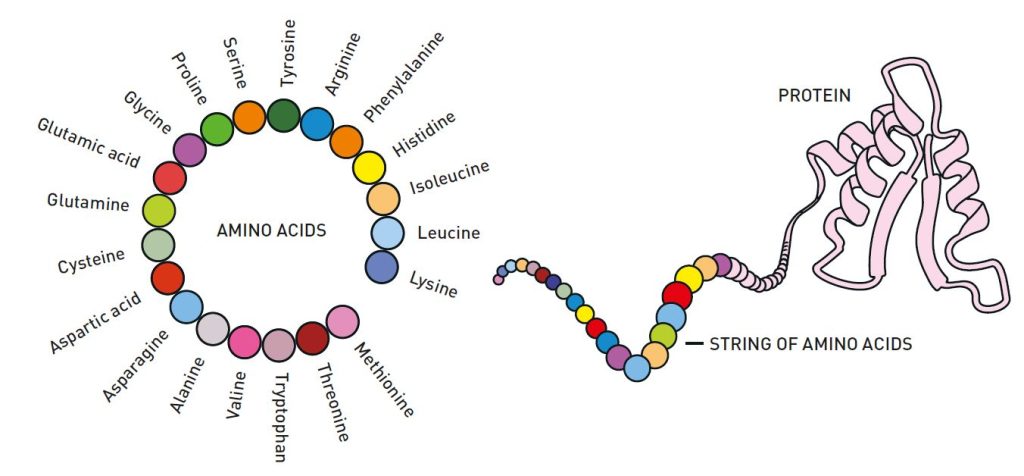
图 1. 一种蛋白质可以由十几个氨基酸到几千个氨基酸组成。氨基酸的链条折叠成三维结构,这对蛋白质的功能至关重要。©Johan Jarnestad/瑞典皇家科学院 © Johan Jarnestad/瑞典皇家科学院
Subsequently, researchers have primarily used X-ray crystallography – and often a great deal of effort – to successfully produce images of around 200,000 different proteins, which laid the foundation for the Nobel Prize in Chemistry 2024.
随后,研究人员主要使用 X 射线晶体学——通常需要付出大量努力——成功地生成了约 200,000 种不同蛋白质的图像,这为 2024 年诺贝尔化学奖奠定了基础。
A riddle: how does a protein find its unique structure?
一个谜题:蛋白质是如何找到其独特结构的?
Christian Anfinsen, an American scientist, made another early discovery. Using various chemical tricks, he managed to make an existing protein unfold and then fold itself up again. The interesting observation was that the protein assumed exactly the same shape every time. In 1961, he concluded that a protein’s three-dimensional structure is entirely governed by the sequence of amino acids in the protein. This led to him being awarded the Nobel Prize in Chemistry in 1972.
克里斯蒂安·安芬森是一位美国科学家,他又做出了一个早期的发现。通过各种化学手段,他成功地使一种现有的蛋白质展开,然后再重新折叠。一个有趣的观察是,这种蛋白质每次都恰好呈现出相同的形状。1961 年,他得出结论,蛋白质的三维结构完全由蛋白质中氨基酸的序列决定。这使他在 1972 年获得了诺贝尔化学奖。
However, Anfinsen’s logic contains a paradox, which another American, Cyrus Levinthal, pointed out in 1969. He calculated that even if a protein only consists of 100 amino acids, in theory the protein can assume at least 1047 different three-dimensional structures. If the chain of amino acids were to fold randomly, it would take longer than the age of the universe to find the correct protein structure. In a cell, it just takes a few milliseconds. So how does the string of amino acids actually fold?
然而,安芬森的逻辑包含一个悖论,另一位美国人赛勒斯·莱文索尔在 1969 年指出了这一点。他计算出,即使一个蛋白质仅由 100 个氨基酸组成,理论上该蛋白质可以呈现至少 1047 种不同的三维结构。如果氨基酸链随机折叠,找到正确的蛋白质结构所需的时间将超过宇宙的年龄。而在细胞中,这只需几毫秒。那么,氨基酸链究竟是如何折叠的呢?
Anfinsen’s discovery and Levinthal’s paradox implied that folding is a predetermined process. And – importantly – all the information about how the protein folds must be present in the amino acid sequence.
安芬森的发现和莱文索尔的悖论暗示了折叠是一个预定的过程。而且——重要的是——关于蛋白质如何折叠的所有信息必须存在于氨基酸序列中。
Throwing down the gauntlet for the great challenge of biochemistry
抛下挑战书,迎接生物化学的伟大挑战
The above insights led to another decisive realisation – if chemists know a protein’s amino acid sequence, they should be able to predict the protein’s three-dimensional structure. This was an exciting idea. If they succeeded, they would no longer have to use fiddly X-ray crystallography and could save masses of time. They would also be able to generate structures for all the proteins where X-ray crystallography was not applicable.
上述见解导致了另一个决定性的认识——如果化学家知道一种蛋白质的氨基酸序列,他们应该能够预测该蛋白质的三维结构。这是一个令人兴奋的想法。如果他们成功了,就不再需要使用繁琐的 X 射线晶体学,从而节省大量时间。他们还将能够为所有不适用 X 射线晶体学的蛋白质生成结构。
These logical conclusions threw down the gauntlet for what has become the great challenge of biochemistry: the prediction problem. To encourage more rapid development in the field, in 1994 researchers started a project called Critical Assessment of Protein Structure Prediction (CASP), which developed into a competition. Every other year, researchers from around the globe were given access to sequences of amino acids in proteins whose structures had just been determined. However, the structures were kept secret from the participants. The challenge was to predict the protein structures based on the known amino acids sequences.
这些逻辑结论向生物化学领域提出了一个巨大的挑战:预测问题。为了促进该领域的快速发展,1994 年,研究人员启动了一个名为蛋白质结构预测的关键评估(CASP)的项目,该项目发展成为一项竞赛。每两年,来自全球的研究人员可以获取刚刚确定结构的蛋白质的氨基酸序列。然而,这些结构对参与者是保密的。挑战在于根据已知的氨基酸序列预测蛋白质结构。
CASP attracted many researchers, but solving the prediction problem proved incredibly difficult. The correspondence between the predictions researchers entered in the competition and the actual structures hardly improved at all. The breakthrough only occurred in 2018, when a chess master, neuroscience expert and pioneer in artificial intelligence entered the field.
CASP 吸引了许多研究人员,但解决预测问题证明是极其困难的。研究人员在比赛中输入的预测与实际结构之间的对应关系几乎没有改善。突破发生在 2018 年,当时一位国际象棋大师、神经科学专家和人工智能的先驱进入了这一领域。
Boardgame master enters the Protein Olympics
桌游大师参加蛋白质奥林匹克比赛
Let’s take a quick look at Demis Hassabis’ background: he started playing chess at the age of four and achieved master level as a 13-year-old. In his teens, he started a career as a programmer and successful games developer. He began exploring artificial intelligence and took on neuroscience, where he made several revolutionary discoveries. He used what he learned about the brain to develop better neural networks for AI. In 2010 he co-founded DeepMind, a company that developed masterful AI models for popular boardgames. The company was sold to Google in 2014 and, two years later, DeepMind came to global attention when the company achieved what many then believed to be the holy grail of AI: beating the champion player of one of the world’s oldest boardgames, Go.
让我们快速了解一下德米斯·哈萨比斯的背景:他在四岁时开始下棋,并在 13 岁时达到了大师级水平。在青少年时期,他开始了程序员和成功游戏开发者的职业生涯。他开始探索人工智能,并涉足神经科学,在这一领域取得了几项革命性的发现。他利用对大脑的理解来开发更好的人工智能神经网络。2010 年,他共同创立了 DeepMind,这是一家为流行棋盘游戏开发出精妙人工智能模型的公司。该公司于 2014 年被谷歌收购,随后两年,DeepMind 因其实现了当时许多人认为是人工智能的圣杯——击败世界上最古老棋盘游戏之一围棋的冠军选手而引起全球关注。
However, for Hassabis, Go was not the goal, it was the means for developing better AI models. After this victory, his team were ready to tackle problems of greater importance for humanity, so in 2018 he registered for the thirteenth CASP competition.
然而,对于哈萨比斯来说,围棋并不是目标,而是开发更好人工智能模型的手段。在这场胜利之后,他的团队准备解决对人类更重要的问题,因此在 2018 年,他注册参加了第十三届 CASP 比赛。
An unexpected win for Demis Hassabis’ AI model
德米斯·哈萨比斯的人工智能模型意外获胜
In previous years, the protein structures that researchers predicted for CASP had achieved an accuracy of 40 per cent, at best. With their AI model, AlphaFold, Hassabis’ team reached almost 60 per cent. They won, and the excellent result took many people by surprise – it was unexpected progress, but the solution was still not good enough. For success, the prediction had to have an accuracy of 90 per cent when compared to the target structure.
在之前的几年中,研究人员为 CASP 预测的蛋白质结构的准确率最多只有 40%。而 Hassabis 的团队通过他们的 AI 模型 AlphaFold 达到了近 60%的准确率。他们获胜了,这一优秀的结果让许多人感到惊讶——这是意想不到的进展,但解决方案仍然不够好。为了成功,预测的准确率在与目标结构比较时必须达到 90%。

图 2. AlphaFold2 是如何工作的?© Johan Jarnestad/瑞典皇家科学院
Hassabis and his team continued developing AlphaFold – but, however hard they tried, the algorithm never quite went all the way. The hard truth was that they had come to a dead end. The team was tired, but one relatively new employee had decisive ideas about how the AI model could be improved: John Jumper.
哈萨比斯和他的团队继续开发 AlphaFold——但无论他们多么努力,这个算法始终未能完全实现。残酷的事实是,他们已经走到了死胡同。团队感到疲惫,但一位相对较新的员工约翰·贾姆珀对如何改进 AI 模型有着决定性的想法。
John Jumper picks up the gauntlet of biochemistry’s big challenge
约翰·贾姆珀接受生物化学重大挑战的挑战
John Jumper’s fascination with the universe was what made him start studying physics and mathematics. However, in 2008, when he started working at a company that used supercomputers to simulate proteins and their dynamics, he realised that knowledge of physics could help solve medical problems.
约翰·贾姆珀对宇宙的迷恋促使他开始学习物理和数学。然而,在 2008 年,当他开始在一家使用超级计算机模拟蛋白质及其动态的公司工作时,他意识到物理知识可以帮助解决医学问题。
Jumper took this newly acquired interest in proteins with him when, in 2011, he began his doctorate in theoretical physics. To save computer capacity – something that was in short supply at the university – he started developing simpler and more ingenious methods for simulating protein dynamics. Soon, he too picked up the gauntlet of biochemistry’s big challenge. In 2017, he had recently completed his doctorate when he heard rumours that Google DeepMind had, in great secrecy, started to predict protein structures. He sent them a job application. His experience of protein simulation meant he had creative ideas about how to improve AlphaFold so, after the team had started to tread water, he was promoted. Jumper and Hassabis co-led the work that fundamentally reformed the AI model.
贾姆珀在 2011 年开始攻读理论物理学博士时,将他新获得的对蛋白质的兴趣带了过去。为了节省计算机资源——这是大学里稀缺的东西——他开始开发更简单、更巧妙的蛋白质动态模拟方法。很快,他也接受了生物化学面临的重大挑战。2017 年,他刚完成博士学位时,听说谷歌 DeepMind 在极度保密的情况下开始预测蛋白质结构。他向他们提交了求职申请。他在蛋白质模拟方面的经验使他对如何改进 AlphaFold 有了创造性的想法,因此在团队开始停滞不前后,他得到了晋升。贾姆珀和哈萨比斯共同领导了这一根本改革 AI 模型的工作。
Astounding results with a reformed AI model
令人惊讶的改进型人工智能模型的结果
The new version – AlphaFold2 – was coloured by Jumper’s knowledge of proteins. The team also started to use the innovation behind the recent enormous breakthrough in AI: neural networks called transformers. These can find patterns in enormous amounts of data in a more flexible manner than previously, and efficiently determine what should be focused on to achieve a particular goal.
新版本——AlphaFold2——受到了 Jumper 对蛋白质的知识的影响。团队还开始使用最近在人工智能领域取得的巨大突破背后的创新:一种称为变换器的神经网络。这些网络能够以比以往更灵活的方式在大量数据中寻找模式,并有效地确定应关注的内容,以实现特定目标。
The team trained AlphaFold2 on the vast information in the databases of all known protein structures and amino acid sequences (Figure 2) and the new AI architecture started delivering good results in time for the fourteenth CASP competition.
该团队在所有已知蛋白质结构和氨基酸序列的数据库中训练了 AlphaFold2(图 2),新的人工智能架构及时开始在第十四届 CASP 竞赛中取得良好结果。
In 2020, when CASP’s organisers evaluated the results, they understood that biochemistry’s 50-year-old challenge was over. In most cases, AlphaFold2 performed almost as well as X-ray crystallography, which was astounding. When one of CASP’s founders, John Moult, concluded the competition on 4 December 2020, he asked – what now?
在 2020 年,当 CASP 的组织者评估结果时,他们意识到生物化学 50 年来的挑战已经结束。在大多数情况下,AlphaFold2 的表现几乎与 X 射线晶体学相当,这令人震惊。当 CASP 的创始人之一约翰·穆尔特在 2020 年 12 月 4 日结束比赛时,他问道——接下来怎么办?
We will return to that. Now we are going to go back in time and shine a light on another participant in CASP. Let’s present the other half of the Nobel Prize in Chemistry 2024, which deals with the art of creating new proteins from scratch.
我们稍后会回到这个话题。现在我们要回到过去,关注 CASP 中的另一个参与者。让我们介绍 2024 年诺贝尔化学奖的另一半,该奖项涉及从头开始创造新蛋白质的艺术。
A textbook about the cell makes David Baker change direction
一本关于细胞的教科书使大卫·贝克改变了方向
When David Baker started studying at Harvard University, he chose philosophy and social science. However, during a course in evolutionary biology he came across the first edition of the now classic textbook Molecular Biology of the Cell. This led to him changing his direction in life. He began to explore cell biology and eventually he became fascinated by protein structures. When, in 1993, he started as group leader at the University of Washington in Seattle, he took on biochemistry’s great challenge. Using clever experiments, he began to explore how proteins fold. This provided insights he took with him when, at the end of the 1990s, he began to develop computer software that could predict protein structures: Rosetta.
当大卫·贝克在哈佛大学学习时,他选择了哲学和社会科学。然而,在一门进化生物学课程中,他遇到了现在经典教科书《细胞的分子生物学》的第一版。这使他改变了人生方向。他开始探索细胞生物学,最终对蛋白质结构产生了浓厚的兴趣。1993 年,他在西雅图的华盛顿大学担任小组负责人时,接受了生物化学的重大挑战。通过巧妙的实验,他开始探索蛋白质如何折叠。这为他提供了见解,后来在 1990 年代末,他开始开发能够预测蛋白质结构的计算机软件:Rosetta。
Baker made his debut in the CASP competition in 1998 using Rosetta and, in comparison to other participants, it did really well. This success led to a new idea – that David Baker’s team could use the software in reverse. Instead of entering amino acid sequences in Rosetta and getting protein structures out, they should be able to enter a desired protein structure and obtain suggestions for its amino acid sequence, which would allow them to create entirely new proteins.
贝克于 1998 年在 CASP 比赛中首次亮相,使用了 Rosetta,与其他参赛者相比表现非常出色。这一成功引发了一个新想法——大卫·贝克的团队可以反向使用该软件。团队应该能够输入所需的蛋白质结构,并获得其氨基酸序列的建议,而不是在 Rosetta 中输入氨基酸序列并得到蛋白质结构,这将使他们能够创造全新的蛋白质。
Baker becomes a protein constructor
贝克成为了一名蛋白质构建者
The field of protein design – where researchers create bespoke proteins with new functions – began to take off at the end of the 1990s. In many cases, researchers tweaked existing proteins, so they could do things like breaking down hazardous substances or functioning as tools in the chemical manufacturing industry.
蛋白质设计领域——研究人员创造具有新功能的定制蛋白质——在 1990 年代末开始蓬勃发展。在许多情况下,研究人员对现有蛋白质进行了调整,使其能够执行诸如分解有害物质或作为化学制造行业工具的功能。
However, the range of natural proteins is limited. To increase the potential for obtaining proteins with entirely new functions, Baker’s research group wanted to create them from scratch. As Baker said, “If you want to build an airplane, you don’t start by modifying a bird; instead, you understand the first principles of aerodynamics and build flying machines from those principles.”
然而,天然蛋白质的种类是有限的。为了增加获得具有全新功能的蛋白质的潜力,贝克的研究小组希望从头开始创造它们。正如贝克所说:“如果你想建造一架飞机,你不会从改造一只鸟开始;相反,你要理解空气动力学的基本原理,并根据这些原理建造飞行器。”

图 3. Top7 - 第一种与所有已知现有蛋白质完全不同的蛋白质。©Terezia Kovalova/瑞典皇家科学院
A unique protein sees the light of day
一种独特的蛋白质首次被发现
The field in which entirely new proteins are constructed is called de novo design. The research group drew a protein with an entirely new structure, and then had Rosetta compute which type of amino acid sequence could result in the desired protein. To do this, Rosetta searched a database of all known protein structures, and looked for short fragments of proteins that had similarities with the desired structure. Using fundamental knowledge of proteins’ energy landscape, Rosetta then optimised these fragments and proposed an amino acid sequence.
全新蛋白质构建的领域称为 de novo 设计。研究小组绘制了一种全新结构的蛋白质,然后让 Rosetta 计算哪种氨基酸序列可以产生所需的蛋白质。为此,Rosetta 搜索了所有已知蛋白质结构的数据库,并寻找与所需结构相似的短肽片段。利用对蛋白质能量景观的基本知识,Rosetta 随后优化了这些片段并提出了一种氨基酸序列。
To investigate how successful the software was, Baker’s research group introduced the gene for the proposed amino acid sequence in bacteria that produced the desired protein. Then they determined the protein structure using X-ray crystallography.
为了研究该软件的成功程度,贝克的研究小组在产生所需蛋白质的细菌中引入了拟议氨基酸序列的基因。然后,他们使用 X 射线晶体学确定了蛋白质的结构。
It turned out that Rosetta really could construct proteins. The protein that the researchers developed, Top7, had almost exactly the structure they had designed.
结果证明,Rosetta 确实能够构建蛋白质。研究人员开发的蛋白质 Top7 几乎完全符合他们设计的结构。
Spectacular creations from Baker’s laboratory
贝克实验室的壮观创作
Top7 was a bolt from the blue for the researchers working on protein design. Those who had previously created de novo proteins had only been able to imitate existing structures. Top7’s unique structure did not exist in nature. Also, with its 93 amino acids, the protein was larger than anything previously produced using de novo design.
Top7 对从事蛋白质设计的研究人员来说是一个晴天霹雳。那些之前创造出新型蛋白质的研究者只能模仿现有的结构。Top7 独特的结构在自然界中并不存在。此外,Top7 的 93 个氨基酸使其比之前使用新型设计生产的任何蛋白质都要大。
Baker published his discovery in 2003. This was the first step in something that can only be described as an extraordinary development; a few of the many spectacular proteins created in Baker’s laboratory can be seen in Figure 4. He also released the code for Rosetta, so a global research community has continued to develop the software, finding new areas of application.
贝克在 2003 年发表了他的发现。这是一个只能被描述为非凡发展的第一步;贝克实验室中创造的众多壮观蛋白质中的一些可以在图 4 中看到。他还发布了 Rosetta 的代码,因此全球研究社区继续开发该软件,寻找新的应用领域。
It is time to tie up the loose ends of the Nobel Prize in Chemistry 2024. What now?
是时候整理 2024 年诺贝尔化学奖的收尾工作了。接下来怎么办?
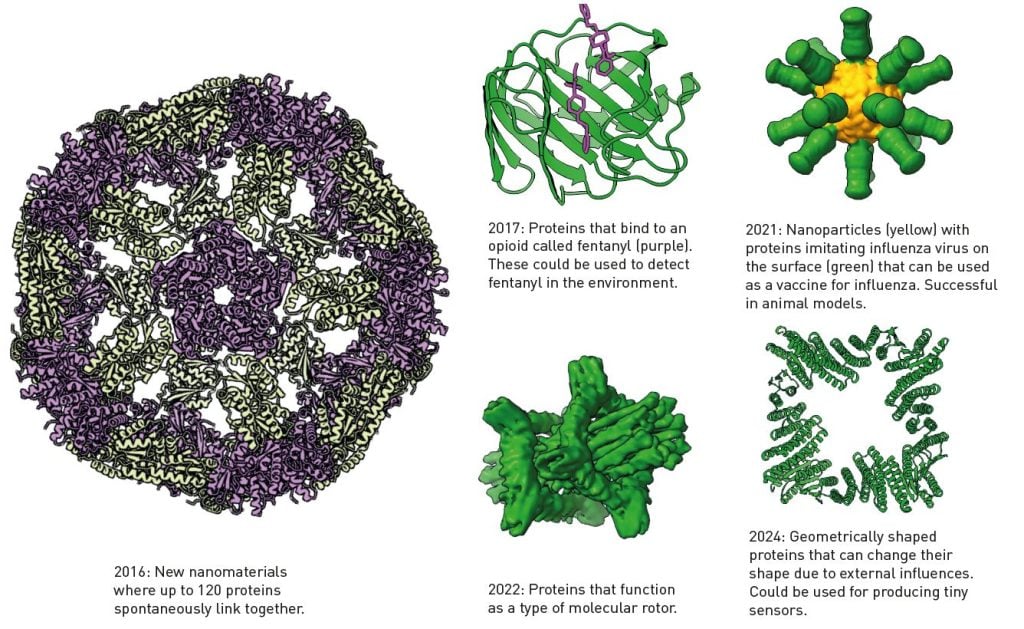
图 4. 使用贝克的程序 Rosetta 开发的蛋白质。©Terezia Kovalova/瑞典皇家科学院
Work that once took years now takes just a few minutes
曾经需要数年的工作现在只需几分钟
When Demis Hassabis and John Jumper had confirmed that AlphaFold2 really worked, they calculated the structure of all human proteins. Then they predicted the structure of virtually all the 200 million proteins that researchers have so far discovered when mapping Earth’s organisms.
当德米斯·哈萨比斯和约翰·贾姆珀确认 AlphaFold2 确实有效时,他们计算了所有人类蛋白质的结构。然后,他们预测了迄今为止研究人员在绘制地球生物时发现的近 2 亿种蛋白质的结构。
Google DeepMind has also made the code for AlphaFold2 publicly available, and anyone can access it. The AI model has become a gold mine for researchers. By October 2024, AlphaFold2 had been used by more than two million people from 190 countries. Previously, it often took years to obtain a protein structure, if at all. Now it can be done in a few minutes. The AI model is not perfect, but it estimates the correctness of the structure it has produced, so researchers know how reliable the prediction is. Figure 5 shows a few of the many examples of how AlphaFold2 helps researchers.
谷歌 DeepMind 还公开了 AlphaFold2 的代码,任何人都可以访问。该人工智能模型已成为研究人员的宝贵资源。截至 2024 年 10 月,AlphaFold2 已被来自 190 个国家的超过两百万用户使用。以前,获得蛋白质结构通常需要数年时间,甚至可能无法获得。现在,这可以在几分钟内完成。该人工智能模型并不完美,但它会估计所生成结构的正确性,因此研究人员可以了解预测的可靠性。图 5 展示了 AlphaFold2 如何帮助研究人员的众多例子中的一些。
After the 2020 CASP competition, when David Baker realised the potential of transformer-based AI models, he added one to Rosetta, which has also facilitated the de novo design of proteins. In recent years, one incredible protein creation after the other has emerged from Baker’s laboratory (Figure 4).
在 2020 年 CASP 比赛之后,当大卫·贝克意识到基于变换器的人工智能模型的潜力时,他将其添加到罗塞塔中,这也促进了蛋白质的 de novo 设计。近年来,贝克实验室不断涌现出令人惊叹的蛋白质创作(图 4)。

图 5. 使用 AlphaFold2 确定的蛋白质结构。©Terezia Kovalova/瑞典皇家科学院
Dizzying development for the benefit of humankind
为人类利益的令人眩晕的发展
Proteins’ amazing versatility as chemical tools is reflected in the vast diversity of life. That we can now so easily visualise the structure of these small molecular machines is mind boggling; it allows us to better understand how life functions, including why some diseases develop, how antibiotic resistance occurs or why some microbes can decompose plastic.
蛋白质作为化学工具的惊人多样性反映在生命的广泛多样性中。我们现在能够如此轻松地可视化这些小分子机器的结构,令人难以置信;这使我们能够更好地理解生命的运作,包括为什么某些疾病会发展、抗生素抗性是如何发生的,以及为什么某些微生物能够分解塑料。
The ability to create proteins that are loaded with new functions is just as astounding. This can lead to new nanomaterials, targeted pharmaceuticals, more rapid development of vaccines, minimal sensors and a greener chemical industry – to name just a few applications that are for the greatest benefit of humankind.
创造具有新功能的蛋白质的能力同样令人惊叹。这可以导致新的纳米材料、靶向药物、疫苗的更快速开发、微型传感器以及更环保的化学工业——仅举几个对人类最有益的应用。
Further reading 进一步阅读
Additional information on this year’s prizes, including a scientific background in English, is available on the website of the Royal Swedish Academy of Sciences, www.kva.se, and at www.nobelprize.org, where you can watch video from the press conferences, the Nobel Lectures and more. Information on exhibitions and activities related to the Nobel Prizes and the Prize in Economic Sciences is available at www.nobelprizemuseum.se.
关于今年奖项的更多信息,包括英文的科学背景,可以在瑞典皇家科学院的网站 www.kva.se 以及 www.nobelprize.org 上找到,在那里您可以观看新闻发布会、诺贝尔讲座等视频。有关诺贝尔奖和经济科学奖相关展览和活动的信息可在 www.nobelprizemuseum.se 上获取。
The Royal Swedish Academy of Sciences has decided to award the Nobel Prize in Chemistry 2024
瑞典皇家科学院决定授予 2024 年诺贝尔化学奖
with one half to
一半到
DAVID BAKER 大卫·贝克
Born 1962 in Seattle, WA, USA. PhD 1989 from University of California, Berkeley, CA, USA. Professor at University of Washington, Seattle, WA, USA and Investigator, Howard Hughes Medical Institute, USA.
出生于 1962 年,美国华盛顿州西雅图。1989 年获得加利福尼亚大学伯克利分校博士学位。现为美国华盛顿大学教授及霍华德·休斯医学研究所研究员。
“for computational protein design”
“用于计算蛋白质设计”
and the other half jointly to
和另一半共同给
DEMIS HASSABIS 德米斯·哈萨比斯
Born 1976 in London, UK. PhD 2009 from University College London, UK. CEO of Google DeepMind, London, UK.
1976 年出生于英国伦敦。2009 年获得伦敦大学学院博士学位。现任谷歌 DeepMind 首席执行官,位于英国伦敦。
JOHN JUMPER 约翰·贾姆珀
Born 1985 in Little Rock, AR, USA. PhD 2017 from University of Chicago, IL, USA. Senior Research Scientist at Google DeepMind, London, UK.
1985 年出生于美国阿肯色州小石城。2017 年获得美国伊利诺伊州芝加哥大学博士学位。现任英国伦敦谷歌 DeepMind 高级研究科学家。
“for protein structure prediction”
“用于蛋白质结构预测”
Science Editors: Peter Brzezinski, Heiner Linke, Johan Åqvist, the Nobel Committee for Chemistry
科学编辑:彼得·布热津斯基,海纳·林克,约翰·阿克维斯特,诺贝尔化学委员会
Text: Ann Fernholm 安·费恩霍尔姆
Translator: Clare Barnes 翻译者:克莱尔·巴恩斯
Illustrations: Johan Jarnestad, Terezia Kovalova
插图:约翰·贾恩斯塔德,特雷齐亚·科瓦洛娃
Editor: Vincent von Sydow
编辑:文森特·冯·西多夫
© The Royal Swedish Academy of Sciences
© 瑞典皇家科学院
Nobel Prizes and laureates
诺贝尔奖与获奖者
Six prizes were awarded for achievements that have conferred the greatest benefit to humankind. The 12 laureates' work and discoveries range from proteins' structures and machine learning to fighting for a world free of nuclear weapons.
六个奖项被授予那些为人类带来最大益处的成就。12 位获奖者的工作和发现涵盖了蛋白质结构、机器学习以及为一个无核武器的世界而奋斗等领域。
See them all presented here.
请在此查看所有内容。
Explore prizes and laureates
探索奖项和获奖者
寻找各个领域的知名奖项和获奖者,并探索诺贝尔奖的历史。