Buildouts of AI infrastructure are insatiable due to the continued improvements from fueling the scaling laws. The leading frontier AI model training clusters have scaled to 100,000 GPUs this year, with 300,000+ GPUs clusters in the works for 2025. Given many physical constraints including construction timelines, permitting, regulations, and power availability, the traditional method of synchronous training of a large model at a single datacenter site are reaching a breaking point.
AI 基础设施的建设需求不断增加,这得益于规模法则的持续改进。今年,领先的前沿 AI 模型训练集群已扩展至 100,000 个 GPU,预计到 2025 年将有超过 300,000 个 GPU 的集群在筹备中。考虑到包括建设时间、许可、法规和电力供应在内的许多物理限制,传统的在单一数据中心站点同步训练大型模型的方法正达到一个临界点。
Google, OpenAI, and Anthropic are already executing plans to expand their large model training from one site to multiple datacenter campuses. Google owns the most advanced computing systems in the world today and has pioneered the large-scale use of many critical technologies that are only just now being adopted by others such as their rack-scale liquid cooled architectures and multi-datacenter training.
谷歌、OpenAI 和 Anthropic 已经在执行计划,将其大型模型训练从一个地点扩展到多个数据中心园区。谷歌拥有当今世界上最先进的计算系统,并且在许多关键技术的大规模使用方面处于领先地位,这些技术现在才刚刚被其他公司采用,例如他们的机架规模液冷架构和多数据中心训练。
Gemini 1 Ultra was trained across multiple datacenters. Despite having more FLOPS available to them, their existing models lag behind OpenAI and Anthropic because they are still catching up in terms of synthetic data, RL, and model architecture, but the impending release of Gemini 2 will change this. Furthermore, in 2025, Google will have the ability to conduct Gigawatt-scale training runs across multiple campuses, but surprisingly Google’s long-term plans aren’t nearly as aggressive as OpenAI and Microsoft.
Gemini 1 Ultra 在多个数据中心进行了训练。尽管他们可用的 FLOPS 更多,但由于在合成数据、强化学习和模型架构方面仍在追赶,他们现有的模型落后于 OpenAI 和 Anthropic,但即将发布的 Gemini 2 将改变这一局面。此外,到 2025 年,谷歌将能够在多个校园进行千兆瓦级别的训练,但令人惊讶的是,谷歌的长期计划并没有 OpenAI 和微软那么激进。
Most firms are only just being introduced to high density liquid cooled AI chips with Nvidia’s GB200 architecture, set to ramp to millions of units next year. Google on the other hand has already deployed millions of liquid cooled TPUs accounting for more than one Gigawatt (GW) of liquid cooled AI chip capacity. The stark difference between Google’s infrastructure and their competitors is clear to the naked eye.
大多数公司刚刚开始接触高密度液冷 AI 芯片,采用 Nvidia 的 GB200 架构,预计明年将增加到数百万个单位。另一方面,谷歌已经部署了数百万个液冷 TPU,拥有超过一吉瓦(GW)的液冷 AI 芯片容量。谷歌的基础设施与其竞争对手之间的明显差异一目了然。
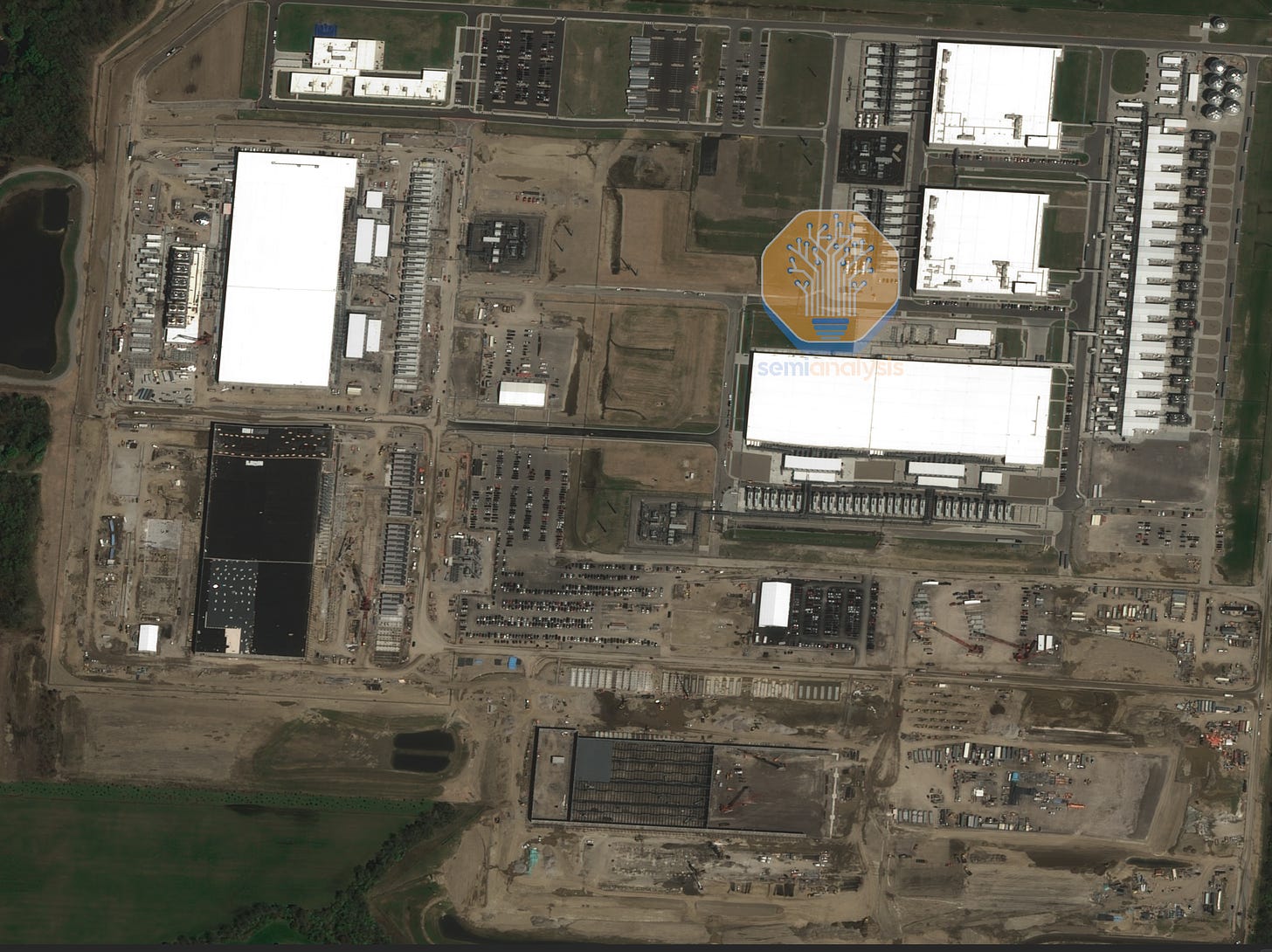
来源:SemiAnalysis 数据中心模型
The AI Training campus shown above already has a power capacity close to 300MW and will ramp up to 500MW next year. In addition to their sheer size, these facilities are also very energy efficient. We can see below the large cooling towers and centralized facility water system with water pipes connecting three buildings and able to reject close to 200MW of heat. This system allows Google to run most of the year without using chillers, enabling a 1.1 PUE (Power Usage Effectiveness) in 2023, as per the latest environmental report.
上述的人工智能训练校园已经拥有接近 300MW 的电力容量,并将在明年提升至 500MW。除了其庞大的规模,这些设施的能效也非常高。我们可以看到下面的大型冷却塔和集中设施水系统,水管连接三栋建筑,能够排放近 200MW 的热量。该系统使谷歌在大部分时间内无需使用冷却机,从而在 2023 年实现了 1.1 的 PUE(电力使用效率),根据最新的环境报告。
While the picture above only shows the facility water system, water is also delivered to the rack via a Direct-to-Chip system, with a Liquid-to-Liquid heat exchanger transferring heat from the racks to the central facility water system. This very energy-efficient system is similar to the L2L deployments of Nvidia GB200 – described in detail in our GB200 deep dive.
上面的图片仅显示了设施水系统,水还通过直接到芯片系统送到机架,液-液热交换器将热量从机架转移到中央设施水系统。这个非常节能的系统类似于 Nvidia GB200 的 L2L 部署——在我们的 GB200 深度分析中有详细描述。
On the other hand, Microsoft’s largest training cluster today, shown below, does not support liquid cooling and has about 35% lower IT capacity per building, despite a roughly similar building GFA (Gross Floor Area). Published data reveals a PUE of 1.223, but PUE calculation is advantageous to air-cooled systems as Fan Power inside the servers are not properly accounted for – that’s 15%+ of server power for an air-cooled H100 server, vs <5% for a liquid DLC-cooled server. Therefore, for each watt delivered to the chips, Microsoft requires an extra ~45%+ power for server fan power, facility cooling and other non-IT load, while Google is closer to ~15% extra load per watt of IT power. Stack on the TPU’s higher efficiency, and the picture is murky.
另一方面,微软目前最大的训练集群,如下所示,不支持液体冷却,每栋建筑的 IT 容量约低 35%,尽管建筑的建筑总面积(GFA)大致相似。发布的数据揭示了 PUE 为 1.223,但 PUE 计算对空气冷却系统有利,因为服务器内部的风扇功率没有被正确计算——对于空气冷却的 H100 服务器,这占服务器功率的 15%以上,而对于液体 DLC 冷却的服务器则低于 5%。因此,对于每瓦特提供给芯片的功率,微软需要额外约 45%以上的功率用于服务器风扇功率、设施冷却和其他非 IT 负载,而谷歌每瓦特 IT 功率的额外负载则接近 15%。再加上 TPU 更高的效率,情况就更加复杂。
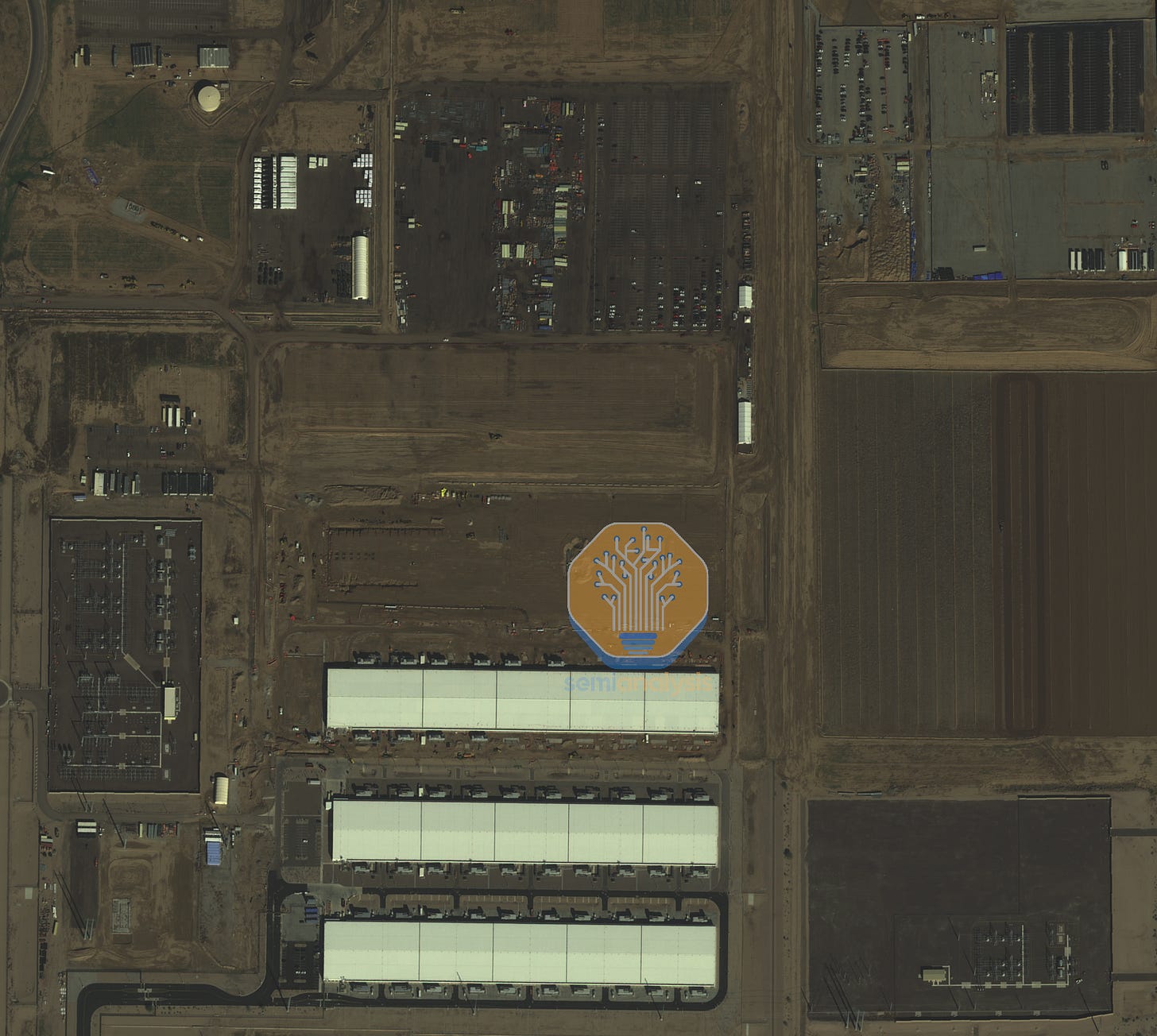
来源:SemiAnalysis 数据中心模型
In addition, to achieve decent energy efficiency in the desert (Arizona), Microsoft requires a lot of water – showing a 2.24 Water Usage Effectiveness ratio (L/kWh), way above the group average of 0.49 and Google’s average slightly above 1. This elevated water usage has garnered negative media attention, and they have been required to switch to air-cooled chillers for their upcoming datacenters in that campus, which will reduce water usage per building but further increase PUE, widening the energy efficiency gap with Google. In a future report, we’ll explore in much more detail how datacenters work and typical hyperscaler designs.
此外,为了在沙漠(亚利桑那州)实现良好的能源效率,微软需要大量水资源——其水使用效率比率为 2.24(L/kWh),远高于集团平均水平 0.49 和谷歌略高于 1 的平均水平。这种高水使用量引起了负面的媒体关注,他们被要求为该校园即将建设的数据中心切换到空气冷却的冷却器,这将减少每栋建筑的水使用量,但进一步增加 PUE,扩大与谷歌之间的能源效率差距。在未来的报告中,我们将更详细地探讨数据中心的工作原理和典型的超大规模设计。
Therefore, based on existing Datacenter reference designs, Google has a much more efficient infrastructure and can build MWs much faster, given that each building has a >50% higher capacity and requires contracting less utility power per IT load.
因此,基于现有的数据中心参考设计,谷歌拥有更高效的基础设施,并且可以更快地建设兆瓦级(MW)数据中心,因为每栋建筑的容量提高了超过 50%,并且每单位 IT 负载所需的公用电力合同更少。
Google’s AI Training Infrastructure
谷歌的人工智能训练基础设施
Google always had a unique way of building infrastructure. While their individual datacenter design is more advanced than Microsoft, Amazon, and Meta’s today, that doesn’t capture the full picture of their infrastructure advantage. Google has also been building large-scale campuses for more than a decade. Google’s Council Bluffs Iowa site, shown below is a great illustration, with close to 300MW of IT capacity on the western portion despite being numerous years old. While significant capacity is allocated to traditional workloads, we believe that the building at the bottom hosts a vast number of TPU. The eastern expansion with their newest datacenter design will further increase the AI training capacity.
谷歌一直以来都有独特的基础设施建设方式。尽管他们的单个数据中心设计今天比微软、亚马逊和 Meta 的更先进,但这并不能全面反映他们的基础设施优势。谷歌已经建设大型校园超过十年。下面展示的谷歌在爱荷华州的 Council Bluffs 站点是一个很好的例子,尽管已经有很多年历史,但西部部分的 IT 容量接近 300MW。虽然大量容量分配给传统工作负载,但我们相信底部的建筑容纳了大量 TPU。东部扩展与他们最新的数据中心设计将进一步增加 AI 训练能力。
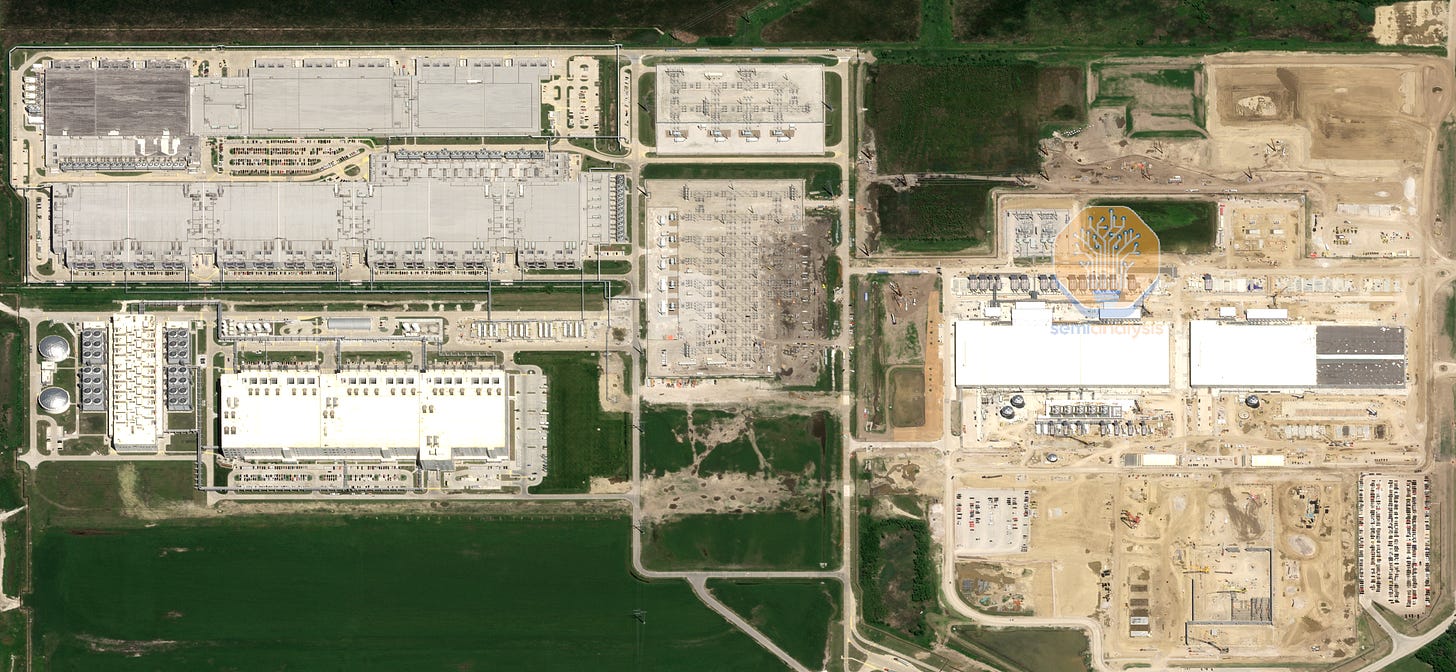
来源:SemiAnalysis 数据中心模型
Google’s largest AI datacenters are also in close proximity to each other. Google has 2 primary multi-datacenter regions, in Ohio and in Iowa/Nebraska. Today, the area around Council Bluffs is actively being expanded to more than twice the existing capacity. In addition to the campus above, Google also owns three other sites in the region which are all under construction and are all being upgraded with high bandwidth fiber networks.
谷歌最大的人工智能数据中心也彼此靠近。谷歌在俄亥俄州和爱荷华州/内布拉斯加州有两个主要的多数据中心区域。今天,关于委员会峡谷周围的区域正在积极扩展,容量将超过现有容量的两倍。除了上述校园,谷歌在该地区还拥有另外三个正在建设中的地点,所有地点都在升级高带宽光纤网络。
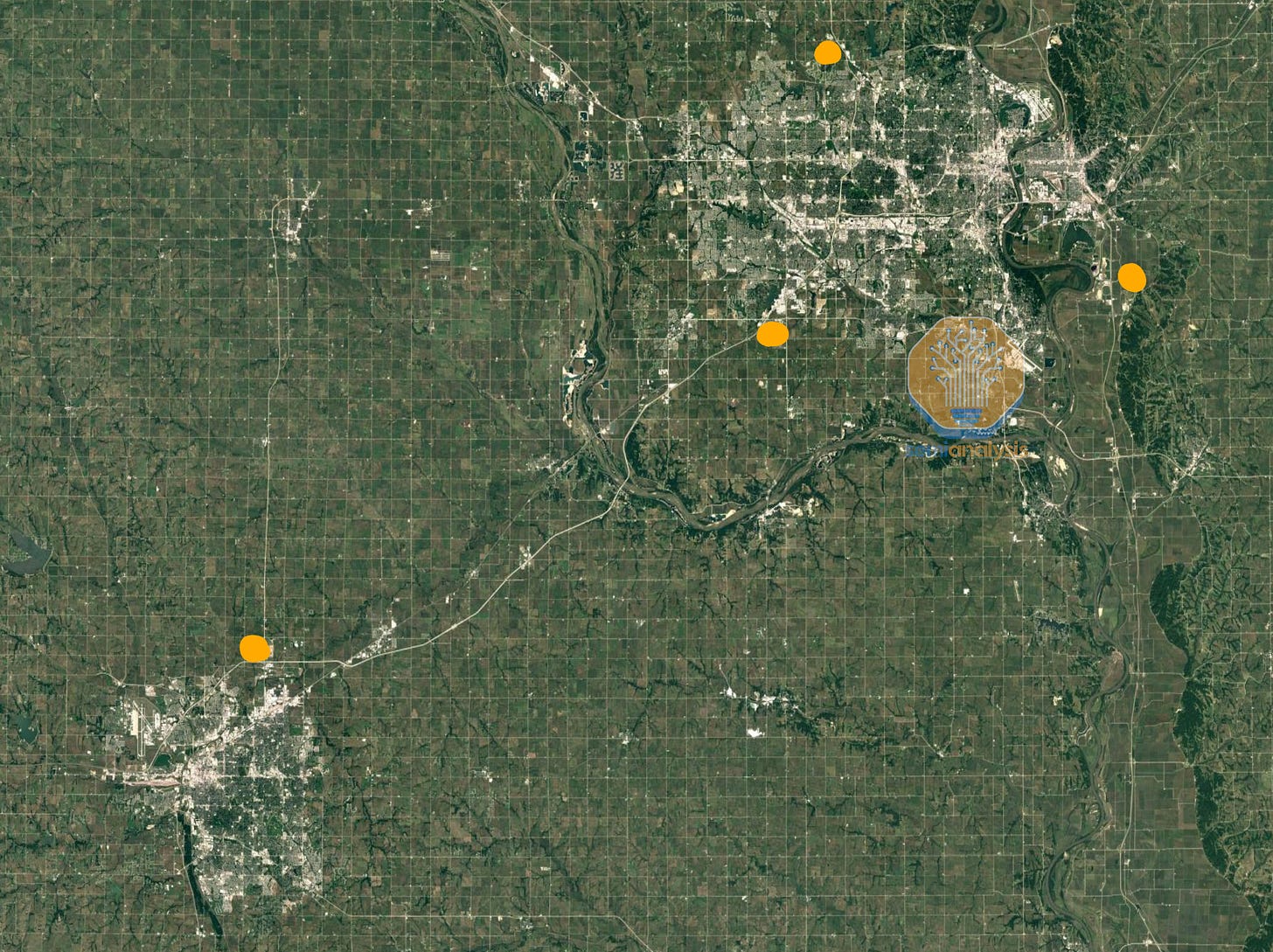
来源:SemiAnalysis 数据中心模型
There are three sites ~15 miles from each other, (Council Bluffs, Omaha, and Papillon Iowa), and another site ~50 miles away in Lincoln Nebraska. The Papillion campus shown below adds >250MW of capacity to Google’s operations around Omaha and Council Bluffs, which combined with the above totals north of 500MW of capacity in 2023, of which a large portion is allocated to TPUs.
有三个地点相距约 15 英里(Council Bluffs、Omaha 和 Papillion Iowa),还有一个地点在内布拉斯加州的林肯,距离约 50 英里。下面显示的 Papillion 校园为谷歌在 Omaha 和 Council Bluffs 的运营增加了超过 250MW 的容量,结合上述地点,2023 年的总容量超过 500MW,其中很大一部分分配给 TPU。
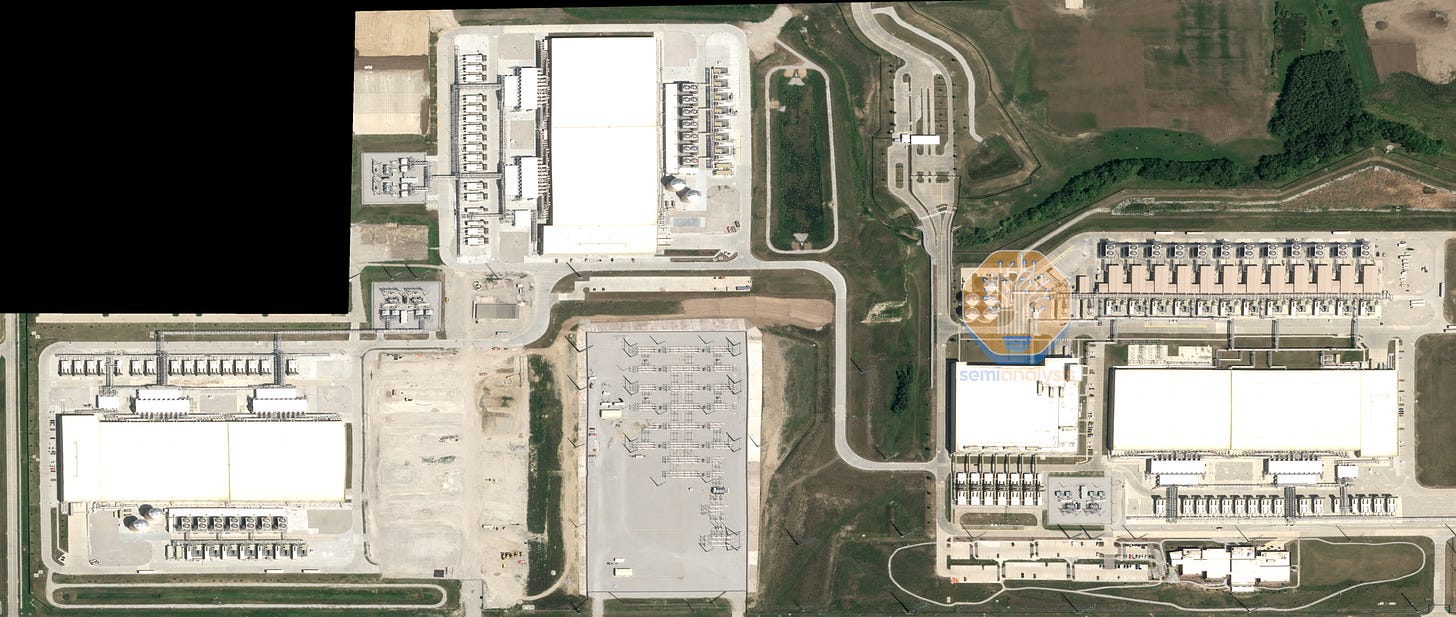
来源:SemiAnalysis 数据中心模型
The other two sites are not as large yet but are ramping up fast: combining all four campuses will form a GW-scale AI training cluster by 2026. The Lincoln datacenter that is ~50 miles away will be Google’s largest individual site.
另外两个站点目前还不够大,但正在快速扩张:到 2026 年,四个校园的结合将形成一个 GW 规模的 AI 训练集群。距离约 50 英里外的林肯数据中心将是谷歌最大的单个站点。
And Google’s massive TPU footprint does not stop here. Another upcoming GW-scale cluster is located around Columbus, Ohio - the region is following a similar leitmotif, with three campuses being developed and summing up to 1 Gigawatt by the end of 2025!
谷歌的大规模 TPU 布局并未止步于此。另一个即将到来的 GW 级集群位于俄亥俄州的哥伦布周边——该地区遵循类似的主题,正在开发三个校园,到 2025 年底总计达到 1 吉瓦!
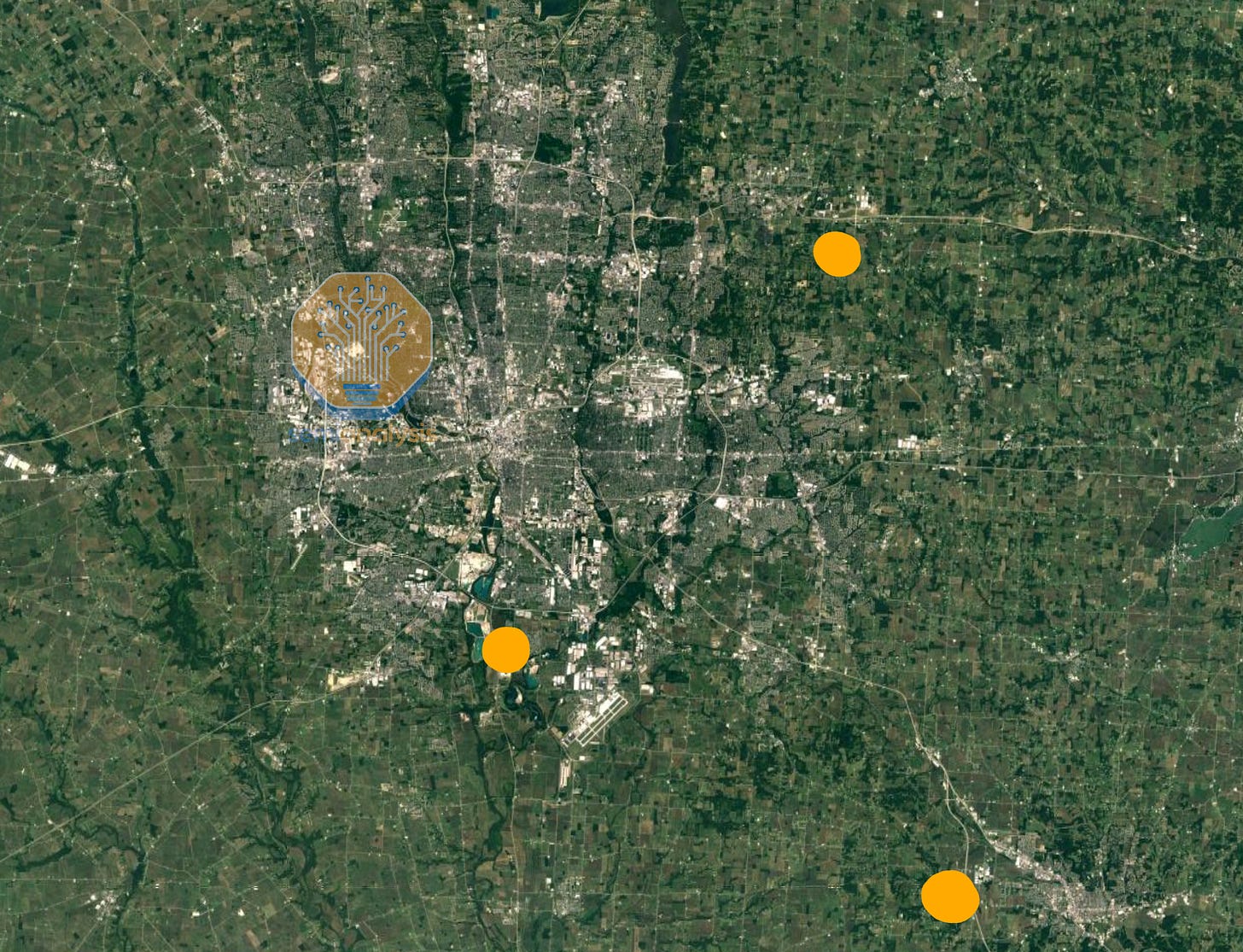
来源:SemiAnalysis 数据中心模型
The New Albany cluster, shown below, is set to become one of Google’s largest and is already hosting TPU v4, v5, v6.
新阿尔巴尼集群,如下所示,预计将成为谷歌最大的集群之一,并已在托管 TPU v4、v5、v6。
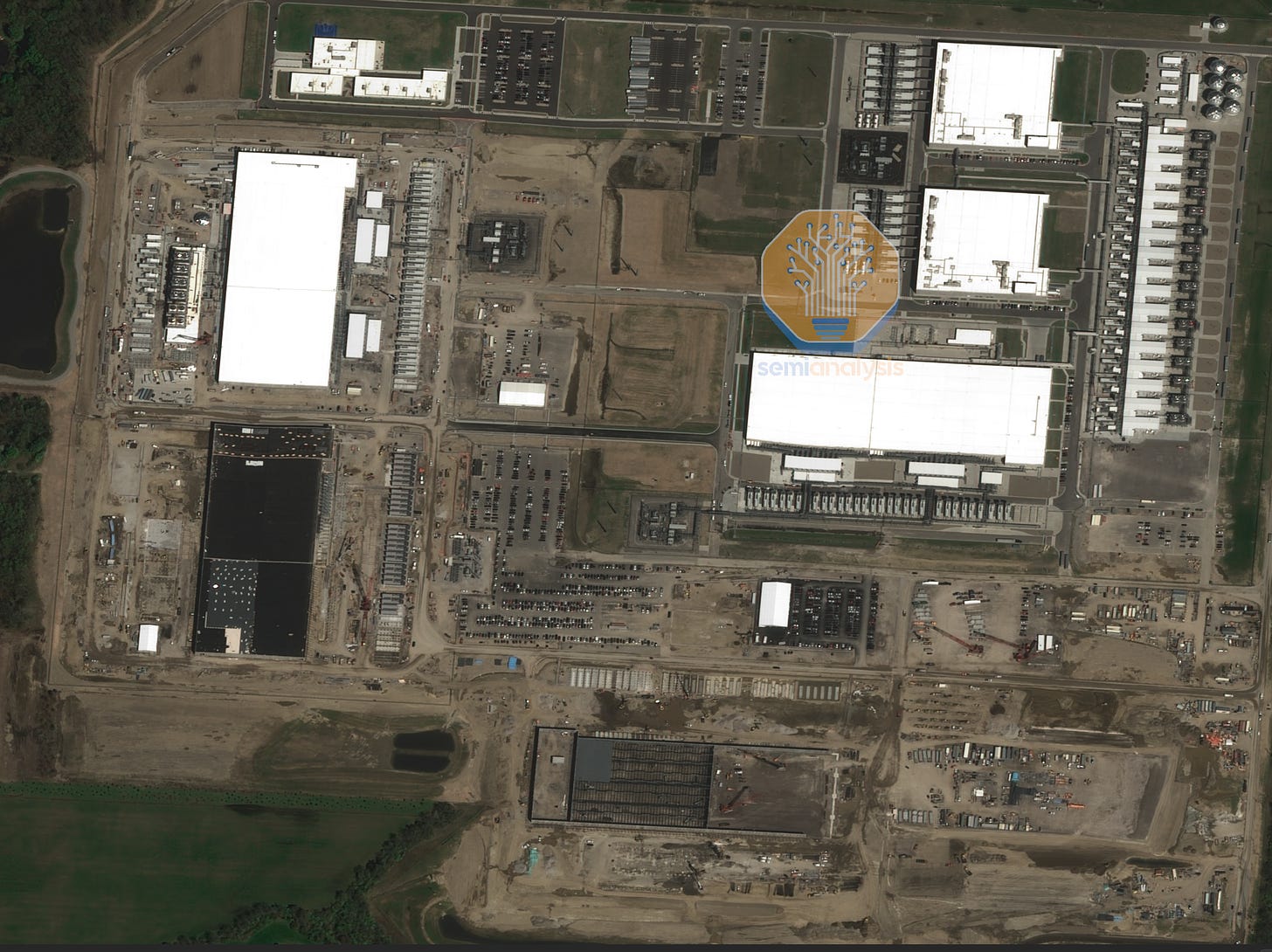
来源:SemiAnalysis 数据中心模型
The concentrated regions of Google Ohio and Google Iowa/Nebraska could also be further interconnected to deliver multiple gigawatts of power for training a single model. We have precisely detailed quarterly historical and forecasted power data of over 5,000 datacenters in the Datacenter Model. This includes status of cluster buildouts for AI labs, hyperscalers, neoclouds, and enterprise. More on the software stack and methods for multi-datacenter training later in this report.
谷歌俄亥俄州和谷歌爱荷华州/内布拉斯加州的集中区域也可以进一步互联,以为训练单个模型提供多个千瓦的电力。我们在数据中心模型中详细列出了超过 5,000 个数据中心的季度历史和预测电力数据。这包括人工智能实验室、超大规模数据中心、新云和企业的集群建设状态。关于软件堆栈和多数据中心训练的方法将在本报告后面详细介绍。
Microsoft and OpenAI Strike Back?
微软和 OpenAI 反击?
Microsoft and OpenAI are well aware of their disadvantages on infrastructure for the near term and have embarked on an incredibly ambitious infrastructure outbuild Google. They are trying to beat Google in their own game of water-cooled multi-datacenter training clusters.
微软和 OpenAI 清楚地意识到他们在基础设施方面的短期劣势,并已着手进行一项极具雄心的基础设施建设,以超越谷歌。他们试图在水冷多数据中心训练集群的游戏中击败谷歌。
Microsoft and OpenAI are constructing ultra-dense liquid-cooled datacenter campuses approaching the Gigawatt-scale and also working with firms such as Oracle, Crusoe, CoreWeave, QTS, Compass, and more to help them achieve larger total AI training and inference capacity than Google.
微软和 OpenAI 正在建设接近千兆瓦规模的超密集液冷数据中心园区,并与 Oracle、Crusoe、CoreWeave、QTS、Compass 等公司合作,帮助他们实现比谷歌更大的 AI 训练和推理能力。
Some of these campuses, once constructed, will be larger than any individual Google campus today. In fact, Microsoft’s campus in Wisconsin will be larger than all of Google’s Ohio sites combined but building it out will take some time.
这些校园建成后,将比今天任何单个的谷歌校园都要大。事实上,微软在威斯康星州的校园将比谷歌在俄亥俄州的所有场地加起来还要大,但建设需要一些时间。
Even more ambitious is OpenAI and Microsoft’s plan to interconnect various ultra large campuses together, and run a giant distributed training runs across the country. Microsoft and OpenAI will be first to a multi-GW computing system. Along with their supply chain partners they are deep into the most ambitious infrastructure buildout ever.
更具雄心的是 OpenAI 和微软计划将多个超大校园互联起来,并在全国范围内进行巨大的分布式训练。微软和 OpenAI 将率先实现多 GW 计算系统。与他们的供应链合作伙伴一起,他们正在进行有史以来最雄心勃勃的基础设施建设。
This report will detail Microsoft and OpenAI’s infrastructure buildout closer to the end. Before that it will first cover multi-campus synchronous and asynchronous training methods, stragglers, fault tolerance, silent data corruption, and various challenges associated with multi-datacenter training.
本报告将在最后详细介绍微软和 OpenAI 的基础设施建设。在此之前,它将首先涵盖多校园同步和异步训练方法、滞后者、容错、静默数据损坏以及与多数据中心训练相关的各种挑战。
Then we will explain how datacenter interconnect as well as metro and long-haul connectivity between datacenters is enabled by fiber optic telecom networks, both technology and equipment.
然后我们将解释数据中心互连以及数据中心之间的城域和长途连接是如何通过光纤电信网络(包括技术和设备)实现的。
Finally, we will explore the telecom supply chain and discuss key beneficiaries for this next leg of the AI infrastructure buildouts including which firms we believe are the most levered to this.
最后,我们将探讨电信供应链,并讨论在下一阶段人工智能基础设施建设中关键受益者,包括我们认为与此最相关的公司。
Multi-Datacenter Distributed Training
多数据中心分布式训练
Before jumping into the Microsoft OpenAI infrastructure buildouts, first a primer on distributed training. Large language models (LLMs) are primarily trained synchronously. Training data is typically partitioned into several smaller mini-batches, each processed by a separate data replica of the model running on different sets of GPUs. After processing a mini-batch, each replica calculates the gradients, then all replicas must synchronize at the end of each mini-batch processing.
在深入了解微软 OpenAI 基础设施建设之前,首先介绍一下分布式训练。大型语言模型(LLMs)主要是同步训练的。训练数据通常被划分为几个较小的迷你批次,每个批次由在不同 GPU 组上运行的模型的单独数据副本处理。在处理完一个迷你批次后,每个副本计算梯度,然后所有副本必须在每个迷你批次处理结束时进行同步。
This synchronization involves aggregating the gradients from all replicas, typically through a collective communication operation like an all-reduce. Once the gradients are aggregated, they are averaged and used to update the model's parameters in unison. This ensures that all data replicas maintain an identical set of parameters, allowing the model to converge in a stable manner. The lock-step nature of this process, where all devices wait for each other to complete before moving to the next step, ensures that no device gets too far ahead or behind in terms of the model’s state.
这种同步涉及从所有副本聚合梯度,通常通过像全归约这样的集体通信操作。一旦梯度被聚合,它们会被平均并用于统一更新模型的参数。这确保所有数据副本保持相同的参数集,使模型能够以稳定的方式收敛。这一过程的锁步特性,所有设备在进入下一步之前相互等待完成,确保没有设备在模型状态上过于领先或落后。
While synchronous gradient descent offers stable convergence, it also introduces significant challenges, particularly in terms of increased communication overhead as you scale above 100k+ chips within a single training job. The synchronous nature also means that you have strict latency requirements and must have a big pipe connecting all the chips since data exchanges happen in giant bursts.
尽管同步梯度下降提供了稳定的收敛性,但它也带来了显著的挑战,特别是在单个训练任务中,当你超过 100k+芯片时,通信开销显著增加。同步特性还意味着你有严格的延迟要求,并且必须有一个大通道连接所有芯片,因为数据交换以巨大的突发方式发生。
As you try to use GPUs from multiple regions towards the same training workload, the latency between them increases. Even at the speed of light in fiber at 208,188 km/s, the round-trip time (RTT) from US east coast to US west coast is 43.2 milliseconds (ms). In addition, various telecom equipment would impose additional latency. That is a significant amount of latency and would be hard to overcome for standard synchronous training.
当您尝试使用来自多个区域的 GPU 进行相同的训练工作负载时,它们之间的延迟会增加。即使在光纤中以 208,188 公里/秒的光速,从美国东海岸到美国西海岸的往返时间(RTT)为 43.2 毫秒(ms)。此外,各种电信设备还会增加额外的延迟。这是一个显著的延迟,对于标准的同步训练来说,很难克服。
According to Amdahl’s Law, the speedup from adding more chips to a workload has diminishing returns when there is a lot of synchronous activity. As you add more chips, and the portion of the program’s runtime that needs synchronization (i.e. corresponding to the proportion of the calculation that remains serial and cannot be parallelized) remains the same, you will reach a theoretical limit where even doubling the number of GPUs will not get you more than a 1% increase in overall throughput.
根据阿姆达尔定律,当工作负载中存在大量同步活动时,增加更多芯片所带来的加速效应会出现递减收益。随着您添加更多芯片,而程序运行时间中需要同步的部分(即对应于仍然是串行且无法并行化的计算比例)保持不变,您将达到一个理论极限,即使将 GPU 的数量翻倍,整体吞吐量的增加也不会超过 1%。
In addition to the theoretical limits of scaling more GPUs towards a single workload described by Amdahl’s Law, there are also the practical challenges of Synchronous Gradient Descent such as stragglers. When just one chip is slower by 10%, it causes the entire training run to be slower by 10%. For example, in the diagram below, from step 7,500 to step 19,000, ByteDance saw their MFU slowly decrease as, one by one, more chips within the workload became slightly slower and the entire workload became straggler-bound.
除了阿姆达尔定律所描述的将更多 GPU 扩展到单一工作负载的理论限制外,还有同步梯度下降的实际挑战,例如滞后者。当只有一个芯片的速度慢了 10%时,整个训练过程的速度也会慢 10%。例如,在下图中,从第 7,500 步到第 19,000 步,字节跳动看到他们的 MFU 缓慢下降,因为工作负载中的更多芯片一个接一个地变得稍微慢一些,整个工作负载变得受滞后者限制。
After identifying and removing the stragglers, they restarted the training workload from a checkpoint, increasing MFU back to a normal level. As you can see, MFU went from 40% to 30%, a 25% percentage decrease. When you have 1 million GPUs, a 25% decrease in MFU is the equivalent of having 250k GPUs running idle at any given time, an equivalent cost of over $10B in IT capex alone.
在识别并移除滞后者后,他们从一个检查点重新启动了训练工作负载,将 MFU 恢复到正常水平。如您所见,MFU 从 40%降至 30%,下降了 25%。当您拥有 100 万个 GPU 时,MFU 下降 25%相当于有 25 万个 GPU 在任何时候处于闲置状态,这仅在 IT 资本支出方面的成本就超过 100 亿美元。
Fault Tolerant Training 容错训练
Fault Tolerant training is an essential part of all distributed systems. When millions of computing, memory, and storage elements are working, there will always be failures or even just silicon lottery in terms of performance differences between various “identical” systems. Systems are designed to deal with this. Counterintuitively in the world’s largest computing problem, machine learning training, the exact opposite approach is used.
容错训练是所有分布式系统的重要组成部分。当数百万个计算、内存和存储单元在工作时,总会出现故障,甚至在各种“相同”系统之间的性能差异上也会有硅彩票。系统的设计旨在应对这一点。与世界上最大的计算问题——机器学习训练——的直觉相反,采用了完全相反的方法。
All chips must work perfectly because if even one GPU fails out of 100k GPUs, this GPU will cause all 100k GPU to restart from checkpoint, leading to an insane amount of GPU idle time. With fault tolerant training, when a single GPU fails, only a few other GPUs will be affected, the vast majority continuing to run normally without having to restart from a model weights checkpoint. Open models such as LLAMA 3.1 have had significant cost and time burned due to this.
所有芯片必须完美运行,因为如果在 10 万块 GPU 中有一块 GPU 故障,这块 GPU 将导致所有 10 万块 GPU 从检查点重新启动,从而导致大量 GPU 闲置时间。通过容错训练,当一块 GPU 故障时,只有少数其他 GPU 会受到影响,绝大多数 GPU 将继续正常运行,而无需从模型权重检查点重新启动。像 LLAMA 3.1 这样的开放模型因此消耗了大量的成本和时间。
Nvidia’s InfiniBand networking also has this same potentially flawed principle in that every packet must be delivered in the exact same order. Any variation or failure leads to a retransmit of data. As mentioned in the 100,000 GPU cluster report, failures from networking alone measure in minutes not hours.
Nvidia 的 InfiniBand 网络也有这个潜在缺陷的原则,即每个数据包必须以完全相同的顺序交付。任何变化或失败都会导致数据的重传。正如在 10 万个 GPU 集群报告中提到的,仅网络故障的时间以分钟计,而不是小时。
The main open-source library that implements fault tolerant training is called TorchX (previously called TorchElastic), but it has the significant drawbacks of not covering the long tail of failure cases and not supporting 3D parallelism. This has led to basically every single large AI lab implementing their own approach to fault tolerant training systems.
主要实现容错训练的开源库称为 TorchX(之前称为 TorchElastic),但它有显著的缺点,即未覆盖长尾故障案例,并且不支持 3D 并行性。这导致几乎每个大型 AI 实验室都实施了自己的容错训练系统方法。
As expected, Google, the leader in fault tolerance infrastructure, has the best implementation of fault tolerant training through Borg and Pathways. These libraries cover of the greatest number of corner cases and are part of a tight vertical integration: Google is designing their own training chips, building their own servers, writing their own infra code, and doing model training too. This is similar to building cars where the more vertically integrated you are, the more quickly you can deal with root causing manufacturing issues and solving them. Google’s Pathways system from a few years ago is a testament to their prowess, which we will describe later in this report.
正如预期的那样,谷歌作为容错基础设施的领导者,通过 Borg 和 Pathways 实现了最佳的容错训练。这些库涵盖了最多的边缘案例,并且是紧密垂直整合的一部分:谷歌正在设计自己的训练芯片,构建自己的服务器,编写自己的基础代码,并进行模型训练。这类似于制造汽车,垂直整合程度越高,越能迅速处理根本的制造问题并解决它们。谷歌几年前的 Pathways 系统证明了他们的实力,我们将在本报告后面进行描述。
In general, fault tolerance is one of the most important aspects to address in scaling clusters of 100k+ GPUs towards a single workload. Nvidia is way behind Google on reliability of their AI systems, which is why fault tolerance is repeatedly mentioned in NVIDIA’s job descriptions…
一般来说,容错是将 100k+ GPU 集群扩展到单一工作负载时需要解决的最重要方面之一。Nvidia 在其 AI 系统的可靠性方面远远落后于 Google,这就是为什么容错在 NVIDIA 的职位描述中反复提到的原因……
Tolerance infrastructure in CPU-land is generally a solved problem. For example, Google’s in house database, called Spanner, runs all of Google’s production services including Youtube, Gmail, and Stadia (RIP) among others, and is able to distribute and scale across the globe while being fault tolerant with respect to storage server and NVMe disk failures. Hundreds of NVMe disks fail per hour in Google datacenters, yet to the end customer and internally, the performance and usability of Spanner stays the same.
在 CPU 领域,容错基础设施通常是一个已解决的问题。例如,谷歌内部的数据库 Spanner 支持谷歌的所有生产服务,包括 YouTube、Gmail 和 Stadia(安息),并能够在全球范围内分布和扩展,同时对存储服务器和 NVMe 磁盘故障具有容错能力。在谷歌的数据中心,每小时有数百个 NVMe 磁盘发生故障,但对最终客户和内部而言,Spanner 的性能和可用性保持不变。
Another example of fault tolerance in traditional CPU workloads on Large Cluster is MapReduce. MapReduce is a style of modelling where users can “map” a data sample by processing it and “reduce” multiple data samples into an aggregated value. For example, counting how many letter “W’s” are in an essay is a great theoretical workload for map-reduce: map each word, the map will output how many letter “W”s are in each data sample, and “reduce” will aggregate the number of “W”s from all the samples. MapReduce can implement Fault Tolerance by detecting which CPUs workers are broken and re-execute failed map and reduce tasks on another CPU worker node.
另一个在大型集群上传统 CPU 工作负载中容错的例子是 MapReduce。MapReduce 是一种建模风格,用户可以通过处理数据样本来“映射”数据,并将多个数据样本“归约”为一个聚合值。例如,计算一篇文章中有多少个字母“W”是一个很好的理论工作负载:映射每个单词,映射将输出每个数据样本中有多少个字母“W”,而“归约”将聚合所有样本中的“W”的数量。MapReduce 可以通过检测哪些 CPU 工作节点出现故障并在另一个 CPU 工作节点上重新执行失败的映射和归约任务来实现容错。
A significant portion of fault tolerance research and systems in CPU land have been developed by Jeff Dean, Sanjay Ghemawat, and the many other world class distributed systems experts at Google. This expertise in creating robust, reliable systems will be one of Google’s competitive advantages as ML training gets larger and requires better fault tolerance ML training systems.
在 CPU 领域,故障容忍研究和系统的很大一部分是由 Jeff Dean、Sanjay Ghemawat 以及谷歌的许多世界级分布式系统专家开发的。这种创建强大、可靠系统的专业知识将是谷歌的竞争优势之一,因为机器学习训练变得越来越大,并且需要更好的故障容忍机器学习训练系统。
Generally, GPU failure follows a bathtub shape curve where most of the failures happen towards the beginning (i.e. infant mortality failures) and near the end of a cluster’s lifespan. This is why cluster-wide burn-in is extremely important. Unfortunately, due to their goal of trying to squeeze the most money out of a cluster’s lifespan, a significant proportion of AI Neoclouds do not properly burn in their cluster, leading to an extremely poor end user experience.
通常,GPU 故障呈现出一个浴缸形状的曲线,其中大多数故障发生在开始阶段(即婴儿死亡率故障)和集群生命周期的末尾。这就是为什么集群范围内的烧机测试极为重要的原因。不幸的是,由于他们试图从集群的生命周期中榨取最多利润,许多 AI Neoclouds 并没有正确地进行集群烧机测试,导致最终用户体验极差。
In contrast, at hyperscalers and big AI labs, most clusters will be burnt in at both high temperatures and rapidly fluctuating temperatures for a significant amount of time to ensure that all the infant mortality failures are past and have shifted into the random failure phrase. Adequate burn in time must be balanced against using too much of the useful life of GPUs and transceivers once they are past early issues.
相反,在超大规模数据中心和大型人工智能实验室,大多数集群将在高温和快速波动的温度下进行长时间的烧机测试,以确保所有的早期故障都已过去,并转入随机故障阶段。必须平衡足够的烧机时间与在 GPU 和收发器早期问题解决后过度消耗其有效使用寿命之间的关系。
The wear out failures phase is when components fail at end of life due to fatigue. Often from rapid fluctuation between medium and high temperatures over a 24/7 usage period. Transceivers in particular suffer from high wear and tear due to severe thermal cycling.
磨损失效阶段是指组件因疲劳在使用寿命结束时发生故障。通常是由于在 24/7 使用期间中高温之间的快速波动。尤其是收发器因严重的热循环而遭受高磨损。
In CPU land, it is common to migrate Virtual Machines (VMs) between physical hosts when the physical host hosting the VM is showing signs of an increased error rate. Hyperscalers have even figured out how to live migration VMs between physical hosts without the user end even noticing that it has been migrated. This is generally done by copying pages of memory in the background and then, when the user’s application slows down for a split second, the VM will be switched rapidly onto the second, normally functioning physical host.
在 CPU 领域,当承载虚拟机(VM)的物理主机出现错误率增加的迹象时,通常会在物理主机之间迁移虚拟机。超大规模云服务商甚至已经找到了在物理主机之间进行实时迁移虚拟机的方法,而用户端甚至没有注意到迁移的发生。这通常是通过在后台复制内存页面来完成的,然后,当用户的应用程序在瞬间变慢时,虚拟机会迅速切换到第二个正常运行的物理主机上。
There is a mainstream Linux software package called CRIU (Checkpoint/Restore In Userspace) that is used in major container engines such as Docker, Podman and LXD. CRIU enables migrating containers and applications between physical hosts and even freezes and checkpoints the whole process state to a storage disk. For a long time, CRIU was only available on CPUs and AMD GPUs as Nvidia refused to implement it until this year.
有一个主流的 Linux 软件包叫做 CRIU(用户空间中的检查点/恢复),它被用于主要的容器引擎,如 Docker、Podman 和 LXD。CRIU 使得在物理主机之间迁移容器和应用程序成为可能,甚至可以将整个进程状态冻结并检查点到存储磁盘。长期以来,CRIU 仅在 CPU 和 AMD GPU 上可用,因为 Nvidia 拒绝实施,直到今年。
With GPU CRIU checkpointing now available on Nvidia GPUs from the beginning of 2024, one can now migrate the CPU process state, memory content and GPU processes from one physical host to another in a far more streamlined manner.
随着 2024 年初 Nvidia GPU 上可用的 GPU CRIU 检查点功能,现在可以以更流畅的方式将 CPU 进程状态、内存内容和 GPU 进程从一个物理主机迁移到另一个物理主机。
In Microsoft’s Singularity Cluster Manager paper the authors described their method of using CRIU for transparent migration of GPU VMs. Singularity is also designed from the ground up to allow for global style scheduling and management of GPU workloads. This system has been used for Phi-3 training (1024 H100s) and many other models. This was Microsoft playing catchup with Google’s vertically integrated Borg cluster manager.
在微软的 Singularity Cluster Manager 论文中,作者描述了他们使用 CRIU 进行 GPU 虚拟机透明迁移的方法。Singularity 也是从零开始设计的,以允许对 GPU 工作负载进行全球风格的调度和管理。该系统已用于 Phi-3 训练(1024 个 H100)和许多其他模型。这是微软在追赶谷歌的垂直集成 Borg 集群管理器。
Unfortunately, due to the importance of fault tolerant training, publishing of methods has effectively stopped. When OpenAI and others tell the hardware industry about these issues, they are very vague and high level so as to not reveal any of their distributed systems tricks. To be clear, these techniques are more important than model architecture as both can be thought of as compute efficiency.
不幸的是,由于容错训练的重要性,方法的发布实际上已经停止。当 OpenAI 和其他公司向硬件行业提及这些问题时,他们的表述非常模糊和高层次,以避免透露他们的分布式系统技巧。明确来说,这些技术比模型架构更重要,因为两者都可以被视为计算效率。
Another common issue is Silent Data Corruption (SDC), which leads computers to inadvertently cause silent errors within the results processed, without any alert to users or administrators. This is a very difficult problem to solve as silent literally means the error is unnoticeable. These silent errors can be trivial in many cases, but they can also cause outputs to be distorted into NaNs (“Not A Number”) or the output gradient to be extremely large. As shown in the gradient norm graph below from Google’s Jeff Dean, some SDCs can be easily identified visually when charted as gradient norm spikes up, but there are other SDCs undetectable by this method.
另一个常见问题是静默数据损坏(SDC),这会导致计算机在处理结果时无意中产生静默错误,而用户或管理员没有任何警报。这是一个非常难以解决的问题,因为静默字面上意味着错误是不可察觉的。这些静默错误在许多情况下可能是微不足道的,但它们也可能导致输出扭曲为 NaN(“不是一个数字”)或输出梯度极大。如下面谷歌的杰夫·迪恩所示的梯度范数图所示,一些 SDC 在绘制为梯度范数尖峰时可以很容易地通过视觉识别,但还有其他 SDC 无法通过这种方法检测。
There are also gradient norm spikes that are not caused by hardware SDCs and are in fact caused by a big batch of data or hyperparameters like learning rate and initialization schemes not being properly tuned. All companies running GPU clusters regularly experience SDCs, but it is the generally small and medium Neoclouds that are unable to quickly identify and fix them due to limited resources.
还有一些梯度范数的峰值并不是由硬件 SDC 引起的,而实际上是由于一大批数据或超参数(如学习率和初始化方案)没有得到适当调整所导致的。所有运行 GPU 集群的公司都会定期经历 SDC,但通常是资源有限的小型和中型 Neoclouds 无法快速识别和修复这些问题。
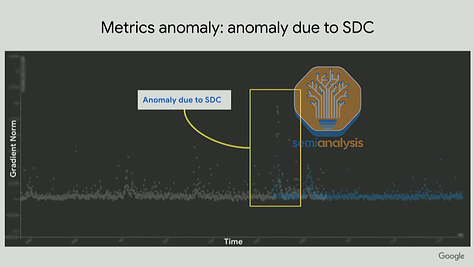
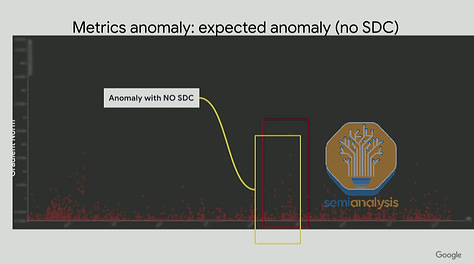
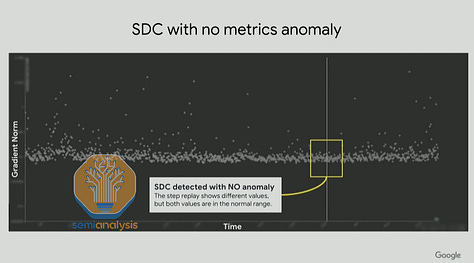
For Nvidia GPUs, there is a tool called DCGMI Diagnostics that helps diagnose GPU errors such as SDCs. It helps catch a good chunk of common SDCs but unfortunately misses a lot of corner cases that result in numerical errors and performance issues.
对于 Nvidia GPU,有一个名为 DCGMI Diagnostics 的工具,可以帮助诊断 GPU 错误,例如 SDC。它能够捕捉到相当一部分常见的 SDC,但不幸的是,错过了许多导致数值错误和性能问题的边缘情况。
Something we experienced in our own testing of H100s from various Neoclouds was that DCGMI diagnostic level 4 was passing, but NVSwitch’s Arithmetic Logic Unit (ALU) was not working properly, leading to performance issues and wrong all-reduce results when utilizing the NVLS NCCL algorithm. We will dive much deeper into our benchmarking findings in an upcoming NCCL/RCCL collective communication article.
我们在对来自不同 Neoclouds 的 H100 进行测试时经历的一个问题是,DCGMI 诊断级别 4 通过了,但 NVSwitch 的算术逻辑单元 (ALU) 工作不正常,导致性能问题和在使用 NVLS NCCL 算法时出现错误的全归约结果。我们将在即将发布的 NCCL/RCCL 集体通信文章中深入探讨我们的基准测试发现。
Google’s Pathways, in contrast, excels at identifying and resolving SDCs. Due to the vertical integration of Google’s infrastructure and training stack, they are able to easily identify SDC checks as epilogue and prologue before starting their massive training workloads.
谷歌的 Pathways 则在识别和解决 SDC 方面表现出色。由于谷歌基础设施和训练堆栈的垂直整合,他们能够轻松识别 SDC 检查作为开始其大规模训练工作负载之前的尾声和序幕。
Asynchronous training used to be a widespread training technique. In 2012, Jeff Dean, the famous 100x engineer from Google Brain, published a paper called Distbelief where he describes both asynchronous (“Async”) and synchronous (“Sync”) gradient descent techniques for training deep learning models on a cluster of thousands of CPU cores. The system introduced a global “parameters server” and was widely used in production to train Google’s autocompletion, search and ad models.
异步训练曾经是一种广泛使用的训练技术。2012 年,谷歌大脑的著名 100 倍工程师杰夫·迪恩发表了一篇名为《Distbelief》的论文,描述了用于在数千个 CPU 核心集群上训练深度学习模型的异步(“Async”)和同步(“Sync”)梯度下降技术。该系统引入了一个全球“参数服务器”,并在生产中广泛用于训练谷歌的自动补全、搜索和广告模型。
This parameter server style training worked very well for models at the time. However, due to convergence challenges with newer model architectures, everyone just simplified their training by moving back to full synchronous gradient descent. All current and former frontier-class models such as GPT-4, Claude, Gemini, and Grok are all using synchronous gradient descent. But to continue scaling the number of GPUs used in a training run, we believe that there is currently a shift back to asynchronous gradient descent.
这种参数服务器风格的训练在当时对模型非常有效。然而,由于新模型架构的收敛挑战,大家都通过回归到全同步梯度下降来简化训练。所有当前和以前的前沿级模型,如 GPT-4、Claude、Gemini 和 Grok,都在使用同步梯度下降。但为了继续扩大训练运行中使用的 GPU 数量,我们认为目前正在转向异步梯度下降。
Training Strategies 培训策略
In Amdahl’s Law, one way of getting around the diminishing returns when adding more chips is to decrease the number of global syncs you need between programs and allow more of the workload to operate (semi)-independently as a percentage of wall time clock. As you can imagine, this maps well to multi-campus, multi-region and cross-continent training as there is a hierarchy of latency and bandwidth between various GPUs.
在阿姆达尔定律中,克服增加更多芯片时收益递减的一种方法是减少程序之间所需的全局同步次数,并允许更多的工作负载以墙钟时间的百分比(半)独立运行。可以想象,这与多校园、多区域和跨洲训练非常契合,因为不同 GPU 之间存在延迟和带宽的层次结构。
Between buildings within a campus, which are very close together (less than 1km), you have very low latency and very high bandwidth and thus are able to synchronize more often. In contrast, when you are within a region (less than 100km), you may have a lot of bandwidth, but the latency is higher, and you would want to synchronize less often. Furthermore, it is acceptable to have different numbers of GPUs between each campus as it is quite easy to load balance between them. For instance, if Campus A has 100k GPUs and Campus B has only 75k GPUs, then Campus B’s batch size would probably be about 75% of Campus A's batch size, then when doing the syncs, you would take a weighted average across the different campuses.
在校园内的建筑之间,如果距离非常近(少于 1 公里),则延迟非常低,带宽非常高,因此能够更频繁地进行同步。相反,当你处于一个区域内(少于 100 公里)时,可能有很多带宽,但延迟较高,因此你会希望减少同步的频率。此外,在每个校园之间拥有不同数量的 GPU 是可以接受的,因为在它们之间进行负载均衡相对容易。例如,如果校园 A 有 10 万个 GPU,而校园 B 只有 7.5 万个 GPU,那么校园 B 的批量大小可能约为校园 A 批量大小的 75%,在进行同步时,你会在不同的校园之间取加权平均值。
This principle can be applied between multiple regions and cross-continents where latency is higher, and as such, you should sync even less. Effectively – there is a hierarchy of sync’ing.
该原则可以应用于多个地区和跨洲际的情况,在这些地方延迟较高,因此您应该更少地进行同步。实际上,存在一个同步的层级结构。
To use an analogy this is akin to how you tend to see friends that are closer to you in terms of distance more often than your friends in other cities on the same coast, and you tend to see your friends on the same coast more often than your friends in cities on other continents.
使用类比来说,这就像你倾向于更频繁地见到距离你更近的朋友,而不是同一海岸上其他城市的朋友,你也倾向于比见到其他大陆城市的朋友更频繁地见到同一海岸的朋友。
Moreover, another benefit of hierarchy synchronous gradient descent (SGD) is that it helps mitigate stragglers as most stragglers usually appear during a couple of steps but then return to their normal performance, so the fewer syncs there are, the fewer opportunities there are for stragglers to disrupt the sync process during their episodes of abnormal performance. Since there is no global sync at every iteration, the effects of stragglers are less prominent. Hierarchal SGD is a very common innovation for multi-datacenter training in the near term.
此外,层次同步梯度下降(SGD)的另一个好处是它有助于减轻滞后者的问题,因为大多数滞后者通常只在几个步骤中出现,但随后会恢复到正常表现,因此同步次数越少,滞后者在异常表现期间干扰同步过程的机会就越少。由于在每次迭代中没有全局同步,滞后者的影响不那么明显。层次 SGD 是近期多数据中心训练中非常常见的创新。
Another promising method is to revisit the use of asynchronous parameter servers as discussed in Jeff Dean’s 2012 DistBelief paper. Each replica of the model processes its own batch of the tokens and every couple of steps, each replica will exchange data with the parameter servers and update the global weights. This is like git version control where every programmer works on their own task for a couple of days before merging it into the master (now called main) branch. A naïve implementation of this approach would likely create convergence issues, but OpenAI will be able to solve update issues in exchanging data from the local model replica into the parameter using various optimizer innovations.
另一种有前景的方法是重新审视异步参数服务器的使用,正如杰夫·迪恩在 2012 年《DistBelief》论文中所讨论的那样。模型的每个副本处理自己的批次数据,每隔几步,每个副本将与参数服务器交换数据并更新全局权重。这就像 git 版本控制,每个程序员在自己的任务上工作几天,然后再合并到主分支(现在称为 main)。这种方法的简单实现可能会导致收敛问题,但 OpenAI 将能够通过各种优化器创新解决从本地模型副本到参数的更新问题。
MetaAI’s Branch-Train-Merge paper describes a similar idea where you branch from an existing LLM (master branch) then train on the subset of the dataset before merging it back into the master branch. We believe that learnings from this approach will be incorporated into multi-campus training techniques that companies such as OpenAI will end up using. The main challenge with Branch-Train-Merge and other similar approaches is that merging is not a solved problem for modern LLMs when it comes to classes of models such as GPT3 175B or GPT4 1.8T. More engineering resources will need to be poured into managing merges and updating the master branch in order to maintain convergence.
MetaAI 的 Branch-Train-Merge 论文描述了一个类似的想法,即从现有的LLM(主分支)分支,然后在数据集的子集上进行训练,然后再将其合并回主分支。我们相信,这种方法的学习将被纳入多校园训练技术中,像 OpenAI 这样的公司最终会使用。Branch-Train-Merge 和其他类似方法的主要挑战在于,对于现代LLMs来说,合并并不是一个解决的问题,尤其是在像 GPT3 175B 或 GPT4 1.8T 这样的模型类别中。需要投入更多的工程资源来管理合并和更新主分支,以保持收敛。
To extend this into a hierarchy approach, we need to also have tiers of parameter servers where data is exchanged between model replicas and the closest parameters servers and also between parameters servers. At the lowest level, individual model replicas communicate with their closest parameter servers, performing updates more frequently to ensure faster convergence and synchronization within local groups.
为了将其扩展为层次化方法,我们还需要有参数服务器的层级,其中数据在模型副本和最近的参数服务器之间以及参数服务器之间进行交换。在最低层级,单个模型副本与其最近的参数服务器进行通信,更频繁地执行更新,以确保在本地组内更快的收敛和同步。
These local parameter servers will be grouped into higher tiers where each tier aggregates and refines the updates from the lower levels before propagating them upwards. Due to the immense number of GPUs involved, parameter servers will probably need to hold the master weights in FP32. This is similar to how Nvidia’s recommended FP8 training server holds the master weights in FP32 so that it doesn’t overflow from many GPUs accumulating. However, before doing the matrix multiply the training servers will downcast to FP8 for efficiency. We believe that this recipe will still hold true where the master weights in the parameter server will be FP32 but the actual calculations will be performed in FP8 or even lower such as MX6.
这些本地参数服务器将被分组到更高的层级,每个层级在向上传播之前聚合和精炼来自下层的更新。由于涉及的 GPU 数量庞大,参数服务器可能需要以 FP32 格式保存主权重。这类似于 Nvidia 推荐的 FP8 训练服务器以 FP32 格式保存主权重,以避免多个 GPU 累积时溢出。然而,在进行矩阵乘法之前,训练服务器将降级为 FP8 以提高效率。我们相信这个方案仍然适用,即参数服务器中的主权重为 FP32,但实际计算将以 FP8 甚至更低的格式(如 MX6)进行。
To achieve multi-campus training, Google currently uses a powerful sharder called MegaScaler that is able to partition over multiple pods within a campus and multiple campuses within a region using synchronous training with Pathways. MegaScaler has provided Google a strong advantage in stability and reliability when scaling up the amount of chips contributing towards a single training workload.
为了实现多校园培训,谷歌目前使用一种强大的分片器,称为 MegaScaler,它能够在一个校园内和一个区域内的多个校园之间对多个节点进行分区,使用与 Pathways 的同步培训。MegaScaler 为谷歌在扩大贡献于单一培训工作负载的芯片数量时提供了稳定性和可靠性的强大优势。
This could be a crutch for them as the industry moves back towards asynchronous training. MegaScaler is built atop the principle of synchronous-style training where each data replica communicates with all other data replicas to exchange data. It may be difficult for them to add asynchronous training to MegaScaler and may require a massive refactor or even starting a new greenfield project. Although Pathways is built with asynchronous dataflow in mind, in practice, all current production use cases of Pathways are fully asynchronous SGD style training. With that said, Google obviously has the capabilities to redo this software stack.
这可能是他们的一个支撑,因为行业正在回归到异步训练。MegaScaler 建立在同步式训练的原则之上,其中每个数据副本与所有其他数据副本进行通信以交换数据。对于他们来说,将异步训练添加到 MegaScaler 可能会很困难,可能需要大规模重构,甚至是启动一个新的绿地项目。尽管 Pathways 是考虑到异步数据流而构建的,但实际上,Pathways 的所有当前生产用例都是完全异步的 SGD 风格训练。话虽如此,谷歌显然有能力重新构建这个软件堆栈。
There are two main limitations when networking datacenters across regions: bandwidth and latency. We generally believe that longer term the limiting factor will be the latency due to speed of light in glass, not bandwidth. This is due to the cost of laying down fiber cables between campus and between regions is mostly the cost of permitting and trenching and not the fiber cable itself. Thus laying 1000 fiber pairs between say Phoenix and Dallas will only be slightly higher cost than laying down 200 fiber pairs. With that said, the industry operates in a regulatory framework and timescales in which fiber pairs cannot be laid in an instant, therefore strategies for reducing bandwidth are still very critical.
在跨区域网络数据中心时,有两个主要限制:带宽和延迟。我们通常认为,从长远来看,限制因素将是由于光在玻璃中的传播速度而导致的延迟,而不是带宽。这是因为在校园和区域之间铺设光纤电缆的成本主要是许可和挖沟的费用,而不是光纤电缆本身的费用。因此,在凤凰城和达拉斯之间铺设 1000 对光纤的成本仅比铺设 200 对光纤稍高。尽管如此,行业仍然在一个监管框架和时间表中运作,光纤对不能瞬间铺设,因此减少带宽的策略仍然非常关键。
We believe the models that will be trained on this multi-campus, multi-region training cluster will be on the order of magnitude of 100T+. Between AZs within a region, we believe that growing to around 5Pbit/s between campus sites within a region is a reasonable assumption of what they can scale to within the near future, while 1Pbit/s is a reasonable amount of bandwidth between regions. If the cross-datacenter bandwidth is that truly that high, exchanging weights between campus sites is not a major bottleneck for training as it only takes 0.64 seconds at line rate. When exchanging 400TeraBytes (4Bytes = param) of weights, only taking 0.64 seconds is very good considering how much time it will take for every couple of compute steps.
我们相信,在这个多校园、多区域的训练集群上训练的模型将达到 100T 以上的数量级。在一个区域内的可用区之间,我们认为在校园站点之间达到约 5Pbit/s 是一个合理的假设,考虑到它们在不久的将来可以扩展到这个水平,而在区域之间 1Pbit/s 是一个合理的带宽。如果跨数据中心的带宽确实如此之高,那么在校园站点之间交换权重对于训练来说并不是一个主要瓶颈,因为在线路速率下只需 0.64 秒。当交换 400TeraBytes(4Bytes = 参数)的权重时,仅需 0.64 秒是非常好的,考虑到每几步计算所需的时间。
While Nvidia offers an InfiniBand fabric networking switch called MetroX within 40kms, no AI lab uses it, only a couple of non-AI HPC clusters that span multiple campuses within 10km. Furthermore, it only has 2x100Gbps per chassis versus the quite mature ecosystem of metro <40km ethernet solutions. As such even Microsoft, who uses InfiniBand heavily, uses Ethernet between datacenters.
尽管 Nvidia 提供了一种名为 MetroX 的 InfiniBand 光纤网络交换机,覆盖范围为 40 公里,但没有 AI 实验室使用它,只有少数非 AI 的高性能计算集群在 10 公里内跨多个校园。此外,它每个机箱仅有 2 个 100Gbps 的带宽,而成熟的 40 公里以内以太网解决方案生态系统则相对完善。因此,即使是大量使用 InfiniBand 的微软,在数据中心之间也使用以太网。
From Gigabits to Terabits: Modulation and Multiplexing
从千兆位到太兆位:调制与复用
Networks within datacenters (i.e. Datacom) today typically focus on delivering speeds of up to 400Gbps per end device (i.e. per GPU) over a fiber link, with the transition to 800Gbps for AI usage to be well underway next year driven by Nvidia’s transition to Connect-X8 Network Interface Cards (NICs).
数据中心内的网络(即 Datacom)通常专注于通过光纤连接为每个终端设备(即每个 GPU)提供高达 400Gbps 的速度,明年将推动 Nvidia 向 Connect-X8 网络接口卡(NICs)的过渡,预计将顺利过渡到 800Gbps 以用于人工智能。
In contrast, telecom networks take communications needs for multiple devices and servers within one facility, and aggregate this onto a smaller number of fibers that run at far greater speeds. While datacom transceivers running 800 Gbps will often utilize only up to 100 Gbps per fiber pair (DR8), requiring multiple separate fiber pairs, telecom applications already fit in excess of 20-40Tbps on just one single-mode fiber pair for submarine cables and many terrestrial and metro deployments.
相反,电信网络将一个设施内多个设备和服务器的通信需求汇聚到更少的光纤上,这些光纤以更高的速度运行。虽然运行在 800 Gbps 的数据信道收发器通常每对光纤仅使用高达 100 Gbps(DR8),需要多个独立的光纤对,但电信应用在单模光纤对上已经能够支持超过 20-40Tbps,适用于海底电缆以及许多陆地和城市部署。
Greater bandwidth is achieved by a combination of
通过组合实现更大的带宽
Higher order modulation schemes, delivering more bits per symbol on a given wavelength.
更高阶调制方案,在给定波长上每个符号传递更多比特。Dense Wave Division Multiplexing (DWDM), which combines multiple wavelengths of light onto a single fiber.
密集波分复用(DWDM),将多个光波长合并到单根光纤上。
On the modulation front, Datacom typically uses VCSEL and EML based transceivers that are capable of PAM4 modulation, an intensity modulation scheme (i.e. Intensity Modulated Direct Detection – IMDD optics) which is achieved by using four different levels to signal, encoding two bits of data per symbol.
在调制方面,Datacom 通常使用基于 VCSEL 和 EML 的收发器,这些收发器能够进行 PAM4 调制,这是一种强度调制方案(即强度调制直接检测 - IMDD 光学),通过使用四个不同的级别来信号化,每个符号编码两个数据位。
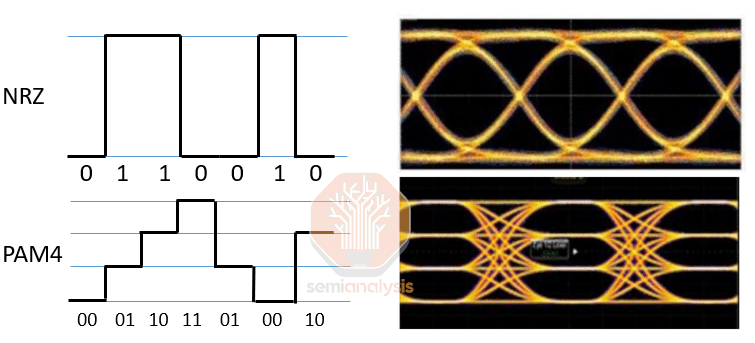
Higher speeds are achieved by either increasing the rate at which symbols are sent (measured in Gigabaud or Gbd) or increasing the number of bits per symbol. For example, a 400G SR8 transceiver could transmit symbols at 26.6 Gbd and use PAM4 to achieve 2 bits per symbol, for a total of 50 Gbps per fiber pair. Combine 8 fiber pairs into one connector and that reaches 400 Gbps overall. Reaching 800Gbps overall could be achieved by increasing the symbol rate to 53.1 Gbd while still using PAM4 across 8 lanes. However, doubling the symbol rate is often a more difficult challenge than using higher order modulation schemes.
更高的速度可以通过增加符号发送的速率(以千兆波特或 Gbd 为单位)或增加每个符号的位数来实现。例如,400G SR8 收发器可以以 26.6 Gbd 的速率发送符号,并使用 PAM4 实现每个符号 2 位,从而每对光纤总共达到 50 Gbps。将 8 对光纤组合成一个连接器,总速率可达到 400 Gbps。要实现 800 Gbps 的总速率,可以将符号速率提高到 53.1 Gbd,同时在 8 条通道上仍使用 PAM4。然而,双倍增加符号速率通常比使用更高阶的调制方案更具挑战性。
16-Quadrature Amplitude Modulation (or 16-QAM) is one such scheme that is widely used in ZR/ZR+ optics and telecom applications. It works by not only encoding four different amplitudes of signal waves, but also using two separate carrier waves that can each have four different amplitudes and are out of phase with each other by 90 degrees, for a total of 16 different possible symbols, delivering 4 bits per symbol. This is further extended by implementing dual polarization, which utilizes another set of carrier waves, with one set of carrier waves on a horizontal polarization state and the other on a vertical polarization state, for 256 possible symbols, achieving 8 bits. Most 400ZR/ZR+ and 800ZR/ZR+ transceivers only support up to DP-16QAM, but dedicated telecom systems (with a larger form factor) running on good quality fiber can support up to DP-64QAM for 12 bits per symbol.
16-四相幅度调制(或 16-QAM)是一种广泛应用于 ZR/ZR+光学和电信应用的方案。它不仅通过编码四种不同的信号波幅度来工作,还使用两种独立的载波波,分别具有四种不同的幅度,并且相位相差 90 度,从而总共可以产生 16 种不同的符号,每个符号传递 4 位信息。这通过实现双极化进一步扩展,利用另一组载波波,其中一组载波处于水平极化状态,另一组处于垂直极化状态,从而实现 256 种可能的符号,达到 8 位信息。大多数 400ZR/ZR+和 800ZR/ZR+收发器仅支持 DP-16QAM,但专用电信系统(具有更大外形因素)在优质光纤上可以支持 DP-64QAM,达到每个符号 12 位信息。
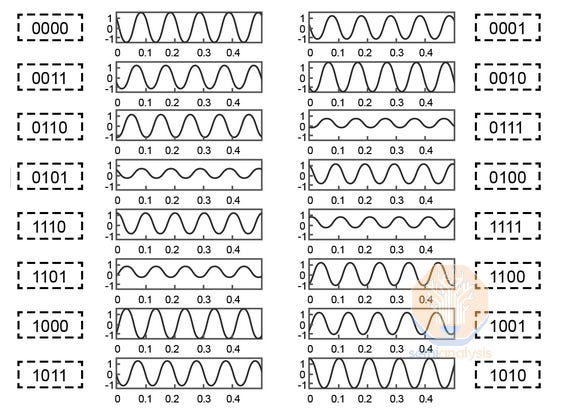
16-QAM 中 16 种不同的可能波形。来源:EverythingRF
To implement modulation schemes using different phases, coherent optics (not to be confused with Coherent the company) are required. Light is considered coherent when the light waves emitted by the source are all in phase with one another – this is important in implementing phase-based modulation schemes because an inconsistent (non-coherent) light source would result in inconsistent interference, making recovery of a phase modulated signal impossible.
要实现使用不同相位的调制方案,需要相干光学(不要与公司 Coherent 混淆)。当光源发出的光波彼此同相时,光被认为是相干的——这在实施基于相位的调制方案时非常重要,因为不一致的(非相干)光源会导致不一致的干涉,使得恢复相位调制信号变得不可能。
Coherent optics require the use of a coherent Digital Signal Processor (DSP) capable of processing higher order modulation schemes, as well as a tunable laser and a modulator, though in the case of 400ZR, silicon photonics are often used to achieve lower cost. Note the tunable laser is very expensive as well and as such, there are attempts to use cheaper O-band lasers in coherent-lite.
相干光学需要使用能够处理高阶调制方案的相干数字信号处理器(DSP),以及可调激光器和调制器,但在 400ZR 的情况下,通常使用硅光子学以实现更低的成本。请注意,可调激光器也非常昂贵,因此有尝试在相干轻型中使用更便宜的 O 波段激光器。
ZR/ZR+ optics are an increasingly popular transceiver type that use coherent optics and are designed specifically for datacenter interconnect, delivering much greater bandwidth per fiber pair and achieving a far greater reach of 120km to 500km. They also typically come in an OSFP or QSFP=DD form factor - the same as is commonly used for datacom applications - meaning they can plug directly into the same networking switches used in datacom.
ZR/ZR+ 光学是越来越受欢迎的收发器类型,采用相干光学,专门为数据中心互连设计,提供每对光纤更大的带宽,并实现 120 公里到 500 公里的更大传输距离。它们通常采用 OSFP 或 QSFP-DD 形状因素——与数据通信应用中常用的相同——这意味着它们可以直接插入用于数据通信的相同网络交换机。
Traditional telecom systems can be used for datacenter interconnect, but this requires a much more complicated chain of telecom equipment occupying more physical space in the datacenter compared to ZR/ZR+ pluggables, which can plug directly into a networking port on either end, sidestepping several telecom devices.
传统电信系统可以用于数据中心互连,但这需要一条更复杂的电信设备链,占用比 ZR/ZR+ 插件更多的物理空间,后者可以直接插入任一端的网络端口,绕过多个电信设备。
Higher order modulation schemes enable more bandwidth per fiber pair, 8x more in the case of DP-16QAM, as compared to Intensity Modulated Direct Detection (IMDD) transceivers using PAM4. Long reach still has fiber limitations though, so Dense Wave Division Multiplexing (DWDM) can also be used to enable even more bandwidth per fiber pair. DWDM works by combining multiple wavelengths of light into one fiber pair. In the below example, 76 wavelengths on the C band (1530nm to 1565nm) and 76 wavelengths on the L band (1565nm to 1625nm) are multiplexed together onto the same fiber.
更高阶调制方案使每对光纤能够提供更多带宽,在 DP-16QAM 的情况下,比使用 PAM4 的强度调制直接检测(IMDD)收发器多 8 倍。然而,长距离传输仍然存在光纤限制,因此密集波分复用(DWDM)也可以用来进一步增加每对光纤的带宽。DWDM 通过将多种波长的光组合到一对光纤中来工作。在下面的示例中,76 个 C 波段(1530nm 到 1565nm)波长和 76 个 L 波段(1565nm 到 1625nm)波长被复用到同一根光纤上。
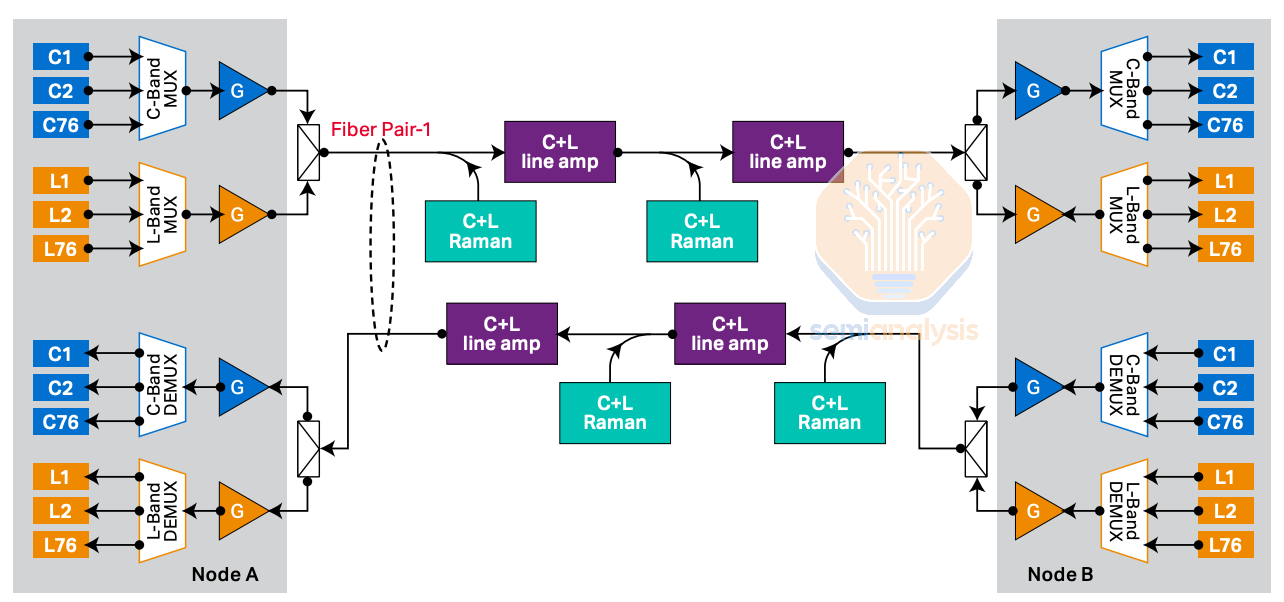
If 800Gbps per wavelength can be deployed on this system – this could yield up to 121.6Tbps for a single fiber pair. Submarine cables will typically maximize the number of wavelengths used, while some deployments might use less than 16 wavelengths, though it is not unheard of to have deployments using 96 wavelengths, with current typical deployments targeting 20-60 Tbps per fiber pair.
如果每个波长可以在该系统上部署 800Gbps,这将为单个光纤对提供高达 121.6Tbps 的带宽。海底电缆通常会最大化使用的波长数量,而某些部署可能使用少于 16 个波长,尽管使用 96 个波长的部署并不罕见,目前典型的部署目标是每个光纤对 20-60 Tbps。
Many deployments start by lighting up only a few wavelengths of light on the C-band and expand along with customer demand by lighting up more of the C-band and eventually the L-band, enabling existing fibers to be upgraded massively in speed over time.
许多部署开始时仅在 C 波段上点亮少数几个波长,并随着客户需求的增加而扩展,点亮更多的 C 波段,最终到达 L 波段,从而使现有光纤的速度在一段时间内大幅提升。
Hyperscalers’ Telecom Network Deployments
超大规模企业的电信网络部署
Most US metros still have an abundance of fiber that can be lit up and harnessed, and the massive bandwidth required by AI datacenter interconnect is a perfect way to sweat this capacity. In submarine cables, consortiums often only deploy 8-12 pairs of fiber as deployment cost scales with number of fiber pairs due to physical cable and deployment. In terrestrial cables, most of the cost is in labor and equipment (and right of way in some urban areas) to dig up the trenches as opposed to the physical fiber, so companies tend to lay hundreds if not thousands of pairs when digging up terrestrial routes in metro areas.
大多数美国大都市仍然拥有丰富的光纤资源,可以被点亮和利用,而人工智能数据中心互联所需的大带宽正是充分利用这些容量的完美方式。在海底电缆中,财团通常只部署 8-12 对光纤,因为部署成本随着光纤对数的增加而增加,这与物理电缆和部署有关。在陆地电缆中,大部分成本来自于人工和设备(在某些城市地区还包括通行权),用于挖掘沟渠,而不是物理光纤,因此公司在挖掘城市地区的陆地线路时往往会铺设数百甚至数千对光纤。
Training across oceans will be significantly more difficult than training across land.
跨越海洋的训练将比跨越陆地的训练困难得多。
A typical fiber optics business case might assume a considerable number of fiber pairs left fallow for future demand. And it is not just metros, but generally any major road, transmission line, railway, or piece of infrastructure tends to have fiber optic cables running alongside – anyone building infrastructure will tend to deploy fiber alongside as a side business as it attracts minimal incremental cost if you’re going to have trenching crews on site anyways.
一个典型的光纤业务案例可能假设有相当数量的光纤对留作未来需求。而且不仅仅是大城市,通常任何主要道路、传输线、铁路或基础设施旁边都会有光纤电缆铺设——任何建设基础设施的人都会倾向于将光纤作为附带业务进行部署,因为如果你已经有挖沟队伍在现场,增加的成本几乎可以忽略不计。
When it comes to hyperscaler telecom networks, the preference is to build their own networks as opposed to working with telecom providers, working directly with equipment vendors and construction companies for long haul, metro, and datacenter interconnect needs.
当谈到超大规模电信网络时,首选是建立自己的网络,而不是与电信供应商合作,而是直接与设备供应商和建筑公司合作,以满足长途、城市和数据中心互联的需求。
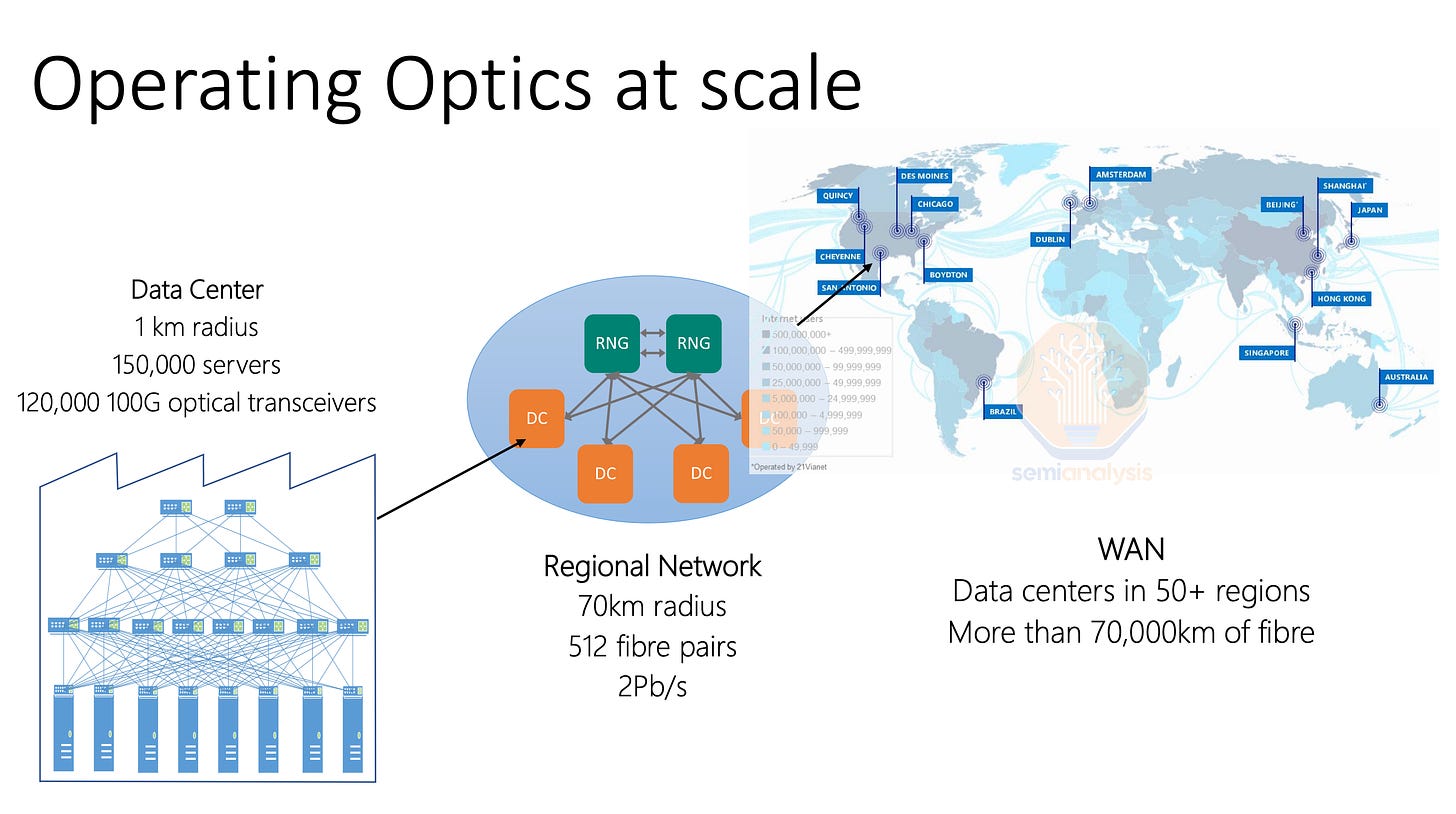
Datacenter interconnect, connecting two datacenters less than about 50km apart in a point-to-point network, is typically built by laying down thousands of fiber optic pairs. The hyperscaler can plug ZR transceivers into network switches inside each of the two distant datacenters, and either tune the transceivers to different wavelengths of light then combine up to 64 transceivers onto a single fiber pair using a passive multiplexer (i.e. a DWDM link), reaching up to 25.5 Tbps per fiber pair if using 400ZR, or simply plug each ZR transceiver into its own fiber pair.
数据中心互联,连接两个相距不到约 50 公里的点对点网络的数据中心,通常通过铺设数千对光纤来构建。超大规模云服务商可以将 ZR 收发器插入两个远程数据中心内部的网络交换机中,或者将收发器调谐到不同的光波长,然后使用无源复用器(即 DWDM 链路)将多达 64 个收发器组合到单个光纤对上,如果使用 400ZR,每个光纤对的传输速率可达到 25.5 Tbps,或者简单地将每个 ZR 收发器插入其自己的光纤对。
More elaborate telecom systems also implementing DWDM can be used to multiplex many more ZR optics signals onto fewer number of fiber pairs and enable more than just a point-to-point network, but this would require a few racks of space for telecom equipment to house routers, ROADMs, and multiplexers/demultiplexers needed for DWDM.
更复杂的电信系统也可以实现 DWDM,能够将更多的 ZR 光学信号复用到更少的光纤对上,并支持不仅仅是点对点网络,但这需要几排电信设备的空间来容纳路由器、ROADM 以及 DWDM 所需的复用器/解复用器。
Since most of the cost is in digging up the trench for the optics, most hyperscalers find it easier to deploy a lot more fiber pairs than needed, saving space within the data hall and avoiding a more complicated telecom deployment. They would typically only resort to deploying extensive telecom systems for short distances if they are deploying fiber in locations that have constraints in obtaining physical fiber capacity, which can be the case outside the United States, when hyperscalers might be forced onto as little as 2-4 fiber pairs in metros with scarce fiber availability.
由于大部分成本在于挖掘光纤的沟槽,大多数超大规模数据中心发现部署更多的光纤对他们来说更容易,从而节省数据中心内的空间,并避免更复杂的电信部署。通常,他们只有在部署光纤的地点在获取物理光纤容量方面存在限制时,才会选择在短距离内部署广泛的电信系统,这种情况在美国以外的地区可能会发生,当超大规模数据中心可能被迫在光纤稀缺的城市中仅使用 2-4 对光纤。
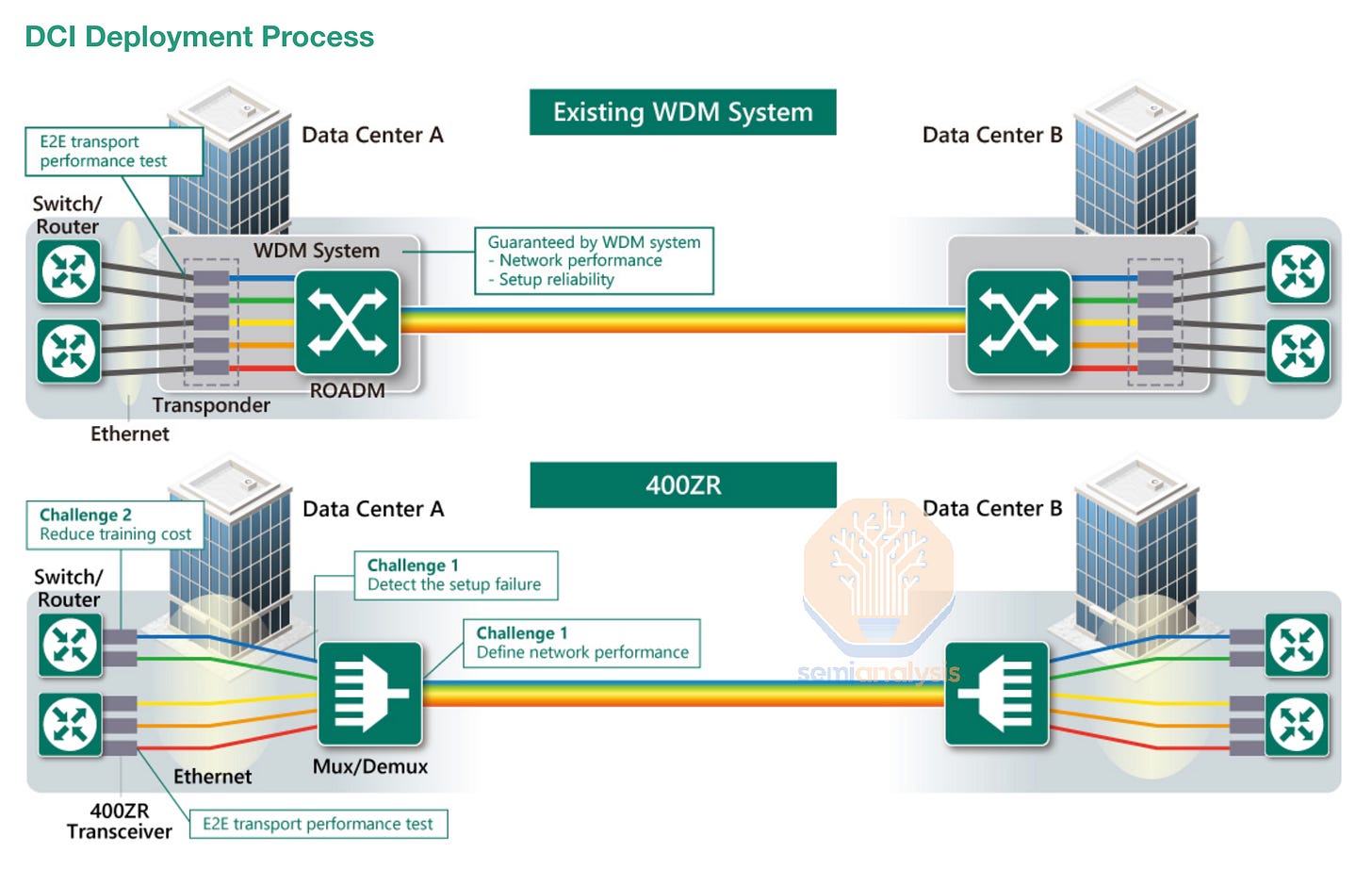
For long-haul networks however, hyperscalers will need to employ a full suite of telecom products that are very distinct from products used in datacom. A typical long-haul network will at least require a few basic systems: Transponders, DWDM Multiplexers/Demultiplexers, Routers, Amplifiers, Gain Equalizers, and Regenerator Sites, and in most but not all cases, ROADMs (Reconfigurable Optical Add/Drop Multiplexers) and WSSs (Wavelength Selective Switches).
对于长途网络,超大规模云计算公司需要使用一整套与数据通信产品截然不同的电信产品。典型的长途网络至少需要几个基本系统:转发器、DWDM 复用器/解复用器、路由器、放大器、增益均衡器和再生站点,在大多数但并非所有情况下,还需要 ROADMs(可重配置光添加/删除复用器)和 WSSs(波长选择开关)。
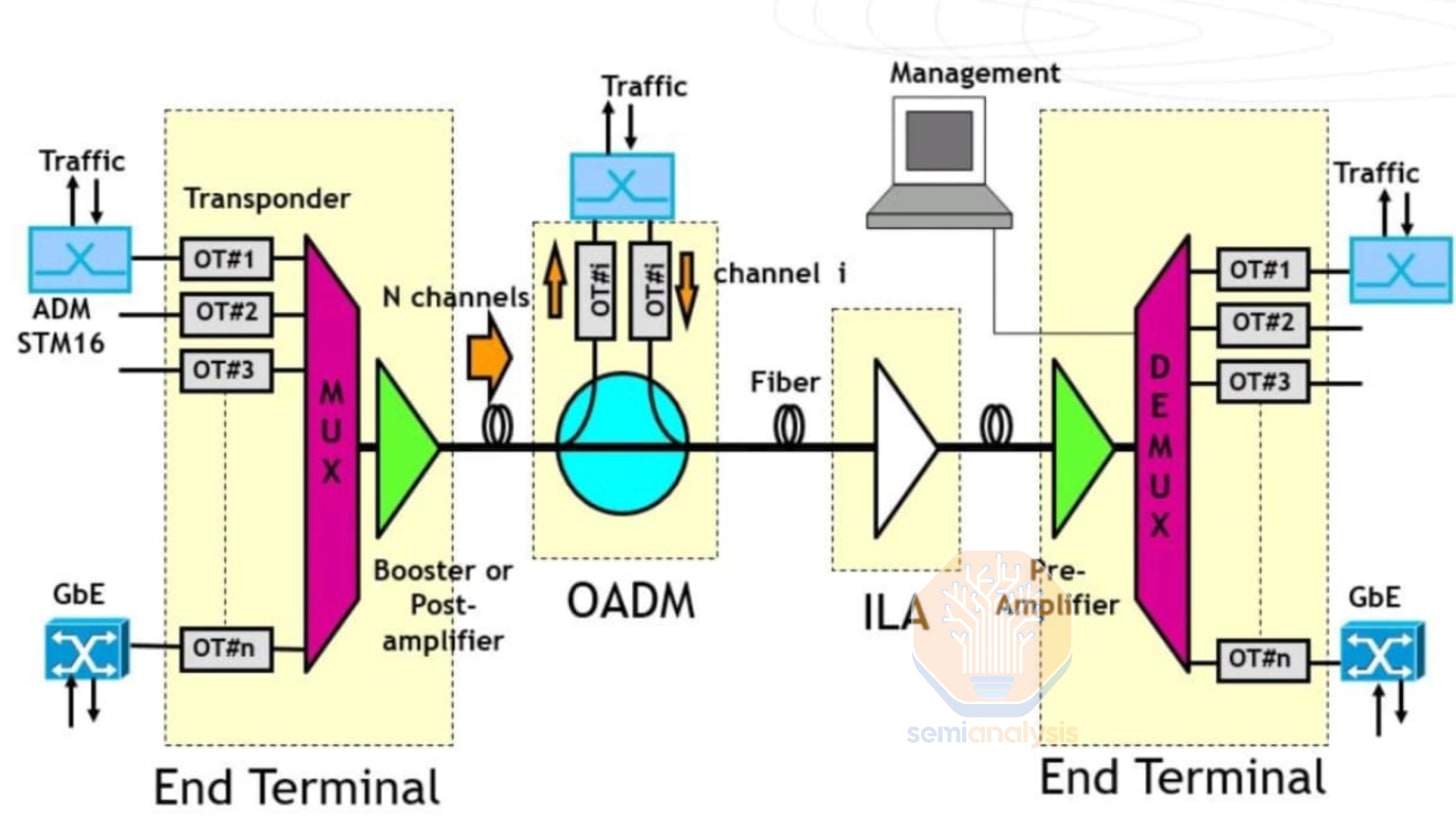
A transponder provides a similar function to a transceiver in the telecom space but is much more expensive and operates at higher power levels. One side transmits/receives into the actual telecom network (line side), with the other offering many possible combinations of ports to connect to client devices (client side) within that location. For example, a transponder might offer 800Gbps on the line side, and 4 ports of 200Gbps optical or electric on the client side, but there are innumerable combinations of port capacities and electrical/optical that customers can choose from. The client side could connect to routers or switches within a datacenter, while the line side will connect to Multiplexers to combine many transponders’ signals using DWDM and potentially ROADMs to allow optical switching for network topologies more complicated than simple point-to-point connectivity.
一个转发器在电信领域提供的功能类似于收发器,但价格更高,功率水平也更高。一侧在实际电信网络中进行传输/接收(线路侧),另一侧则提供多种可能的端口组合,以便在该位置连接到客户端设备(客户端侧)。例如,一个转发器可能在线路侧提供 800Gbps,在客户端侧提供 4 个 200Gbps 的光电端口,但客户可以选择无数种端口容量和电气/光学组合。客户端可以连接到数据中心内的路由器或交换机,而线路侧将连接到多路复用器,以使用 DWDM 结合多个转发器的信号,并可能使用 ROADM 进行光交换,以支持比简单点对点连接更复杂的网络拓扑。
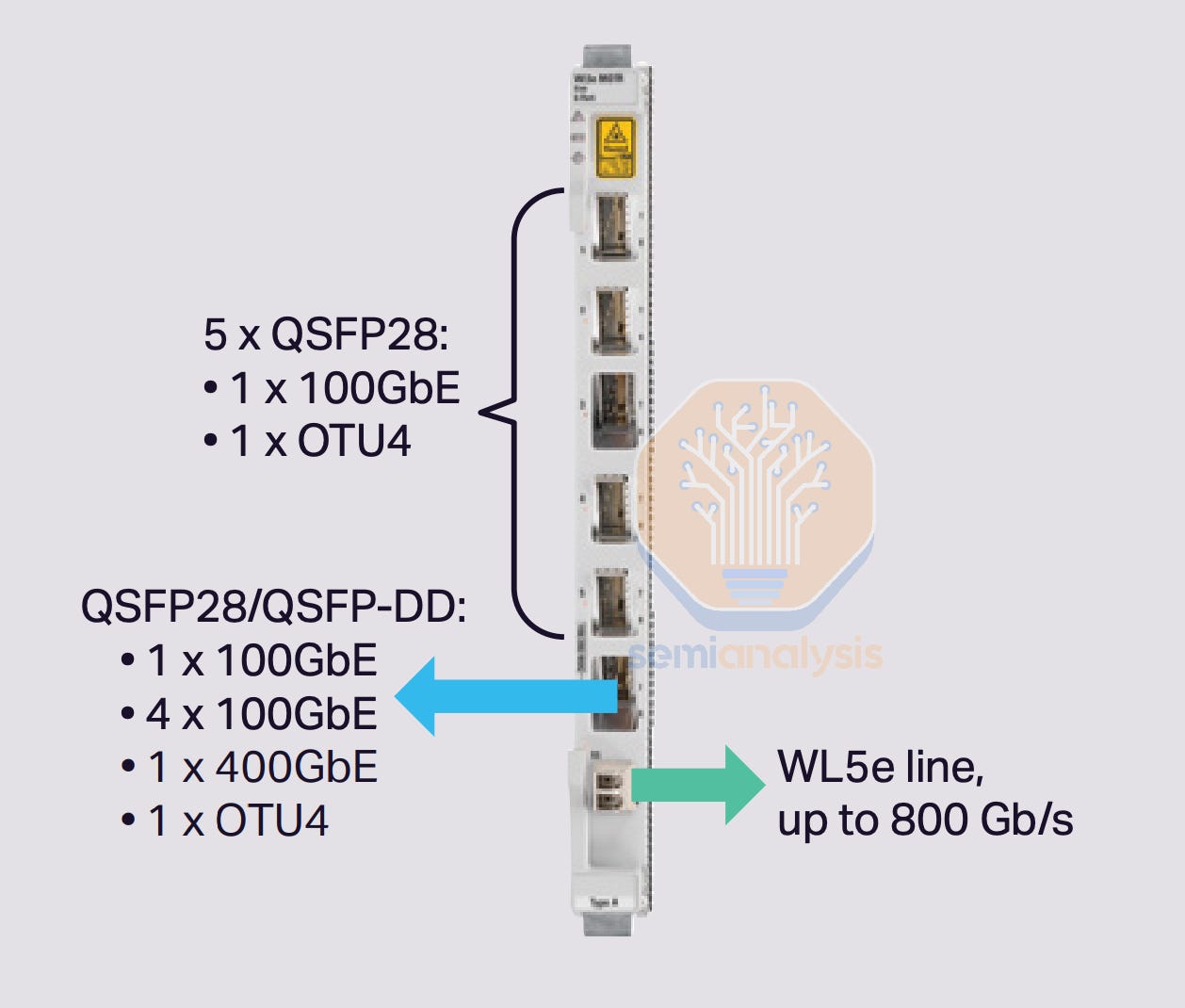
典型的应答器。来源:Ciena
DWDM works using a multiplexer and demultiplexer (mux/demux) that takes slightly different wavelengths of light signals from each transponder and combines it onto one fiber optic pair. Each transponder is tunable and can dial in specific wavelengths of light for the multiplexing onto that same fiber pair. When using a ROADM, transponders will typically connect to a colorless mux/demux, and from there to a Wavelength Selective Switch (WSS), allowing the ROADM to dynamically tune transponders to specific wavelengths in order to optimize for various network objectives.
DWDM 使用一个多路复用器和解复用器(mux/demux),将来自每个收发器的略微不同波长的光信号组合到一对光纤上。每个收发器都是可调的,可以拨入特定的光波长以进行多路复用到同一光纤对上。当使用 ROADM 时,收发器通常会连接到无色多路复用器/解复用器,然后再连接到波长选择开关(WSS),允许 ROADM 动态调谐收发器到特定波长,以优化各种网络目标。
Optical amplifiers are needed to combat the attenuation of light signals over long distances on fiber. Amplifiers are placed every 60-100km on the fiber route and can amplify the optical signal directly without having to convert the optical signal to an electrical signal. A gain equalizer is needed every after three amplifiers to ensure that different wavelengths of light traveling at different speeds are equalized to avoid errors. In some very long-haul deployments of thousands of kilometers, regeneration is needed, which involves taking the optical signal off into electronics, reshaping and retiming the signal, and retransmitting it using another set of transponders.
光放大器是为了应对光信号在光纤长距离传输中的衰减而需要的。放大器每 60-100 公里放置一次,可以直接放大光信号,而无需将光信号转换为电信号。每三个放大器后需要一个增益均衡器,以确保以不同速度传播的不同波长的光信号被均衡,以避免错误。在一些数千公里的超长距离部署中,需要进行再生,这涉及将光信号转换为电子信号,重塑和重新定时信号,并使用另一组转发器重新发送。
If the network connects more than two points together and has multiple stops where traffic is added or received at, then a ROADM (Reconfigurable Optical Add/Drop Multiplexer) is needed. This device can optically add or drop specific wavelengths of light at a given part of the network without having to offload any of the signals to an electrical form for any processing or routing. Wavelengths that are to be transmitted or received by a given location can be added or dropped from the main fiber network, while others that do not carry traffic to that location can travel through the ROADM unimpeded. ROADMs also have a control plane and it can actively discover and monitor the network state, understanding which channels on the fiber network are free, channel signal to noise ratio, reserved wavelengths, and as discussed above, can control transponders, tuning the line side to the appropriate wavelength.
如果网络连接超过两个点并且有多个停靠点用于添加或接收流量,则需要一个 ROADM(可重构光添加/删除复用器)。该设备可以在网络的特定部分光学地添加或删除特定波长的光,而无需将任何信号转换为电信号进行处理或路由。要在特定位置传输或接收的波长可以从主光纤网络中添加或删除,而不向该位置传输流量的其他波长可以不受阻碍地通过 ROADM。ROADM 还具有控制平面,可以主动发现和监控网络状态,了解光纤网络上哪些通道是空闲的、通道信号与噪声比、保留的波长,并且如上所述,可以控制转发器,将线路侧调谐到适当的波长。
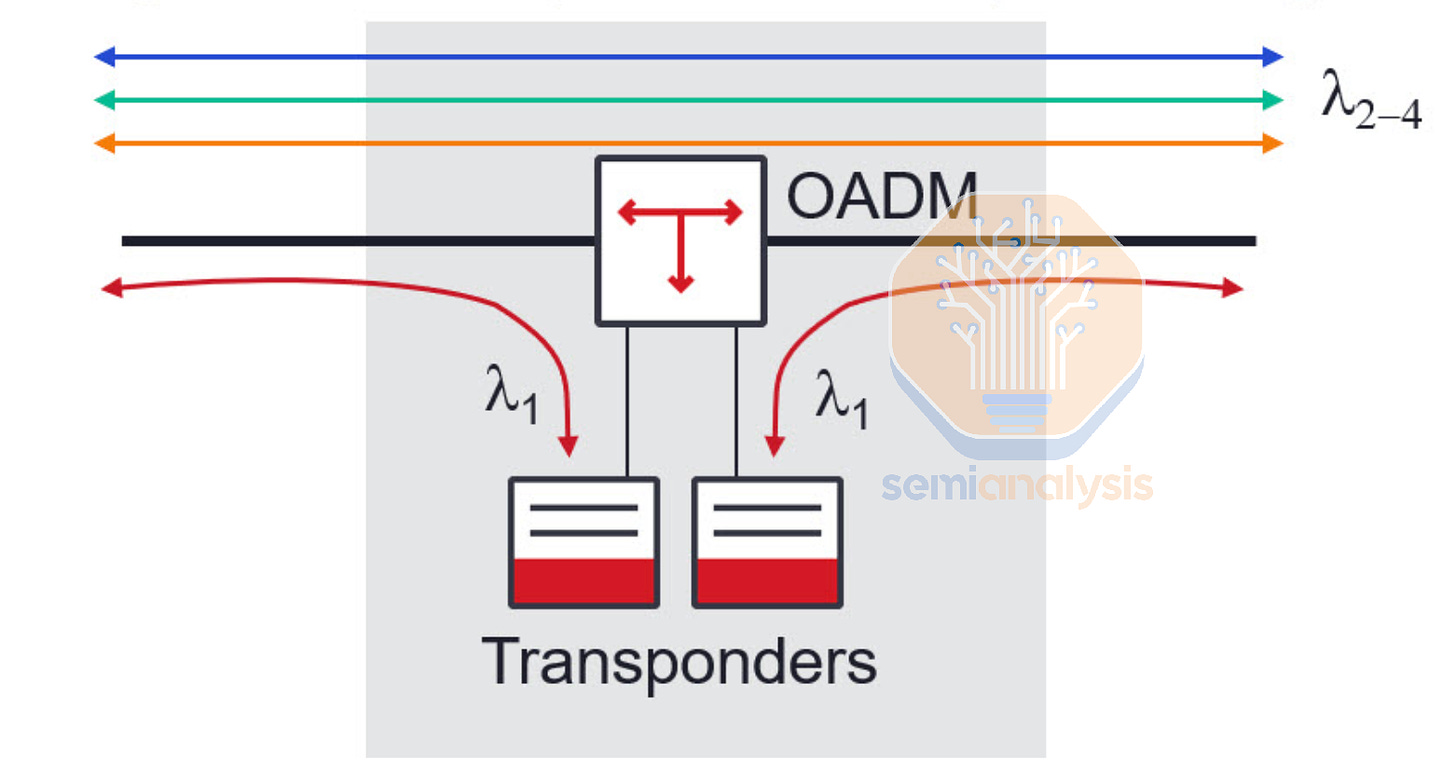
These various components are typically combined together in a modular chassis that could look something like this:
这些不同的组件通常组合在一个模块化机箱中,可能看起来像这样:
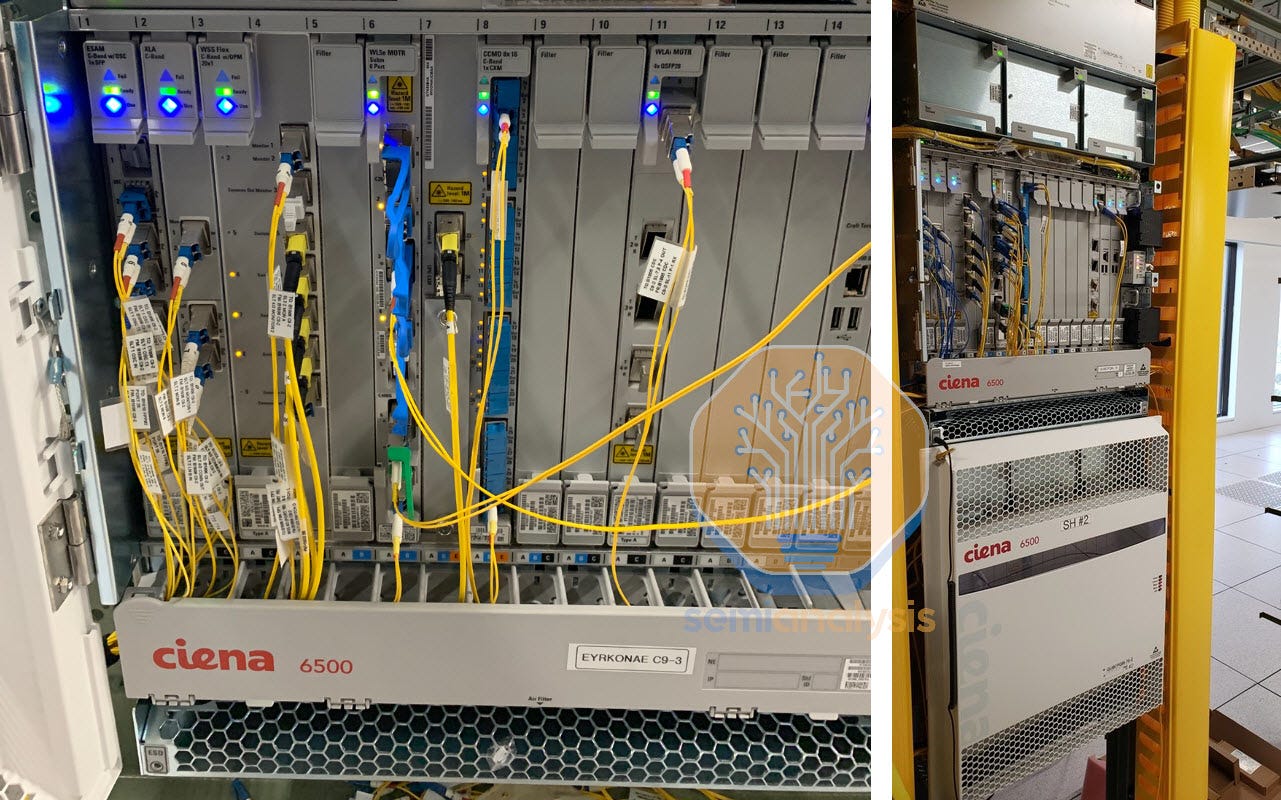
来源:光连接新闻
Ciena, Nokia, Infinera and Cisco are a few major global suppliers of telecom systems and equipment, while Lumentum, Coherent, Fabrinet and Marvell provide various subsystems and active components to these major suppliers. Much of the strength for the component players so far has been seen in ZR/ZR+ optics for datacenter interconnect, but as hyperscalers and other operators have to get serious about training beyond adjacent data centers, they could potentially significantly hike their spending on much higher ASP telecom equipment and systems.
Ciena、诺基亚、Infinera 和思科是一些主要的全球电信系统和设备供应商,而 Lumentum、Coherent、Fabrinet 和 Marvell 向这些主要供应商提供各种子系统和主动组件。到目前为止,组件厂商的实力主要体现在数据中心互联的 ZR/ZR+ 光学上,但随着超大规模数据中心和其他运营商必须认真考虑超出相邻数据中心的培训,他们可能会显著增加对更高平均售价电信设备和系统的支出。
Demand for telecom equipment from non-cloud customers also appears to have troughed and could enter a recovery phase of the cycle soon – boosting the fortunes of various telecom suppliers.
来自非云客户的电信设备需求似乎也已触底,并可能很快进入周期的复苏阶段——这将提升各类电信供应商的前景。
Next, let’s discuss the ambitious multi-datacenter training plans of OpenAI and Microsoft as well as the winners in the telecom space for this massive buildout.
接下来,让我们讨论 OpenAI 和微软雄心勃勃的多数据中心培训计划,以及在这次大规模建设中电信领域的赢家。
How OpenAI and Microsoft Plan to Beat Google
OpenAI 和微软如何计划击败谷歌
As explained previously, Microsoft’s standard design, shown below, has a density disadvantage compared to Google’s. While square footage of each of their buildings is roughly equivalent, MW capacity is lower. Google’s facilities also have a lower PUE, meaning that a higher share of power contracted with the utility that can be supplied to IT Equipment and an even lower share that goes to the chip versus associated networking, CPUs, fans, etc. Therefore, while Microsoft also has experience building large campuses, they tend to take longer to build and are generally smaller than Google’s.
如前所述,微软的标准设计,如下所示,与谷歌相比在密度上存在劣势。尽管他们每栋建筑的面积大致相当,但 MW 容量较低。谷歌的设施还具有更低的 PUE,这意味着与公用事业公司签订的电力合同中,能够提供给 IT 设备的电力比例更高,而用于芯片的比例则更低,相较于相关的网络、CPU、风扇等。因此,尽管微软在建设大型园区方面也有经验,但它们的建设时间往往更长,且通常比谷歌的园区要小。
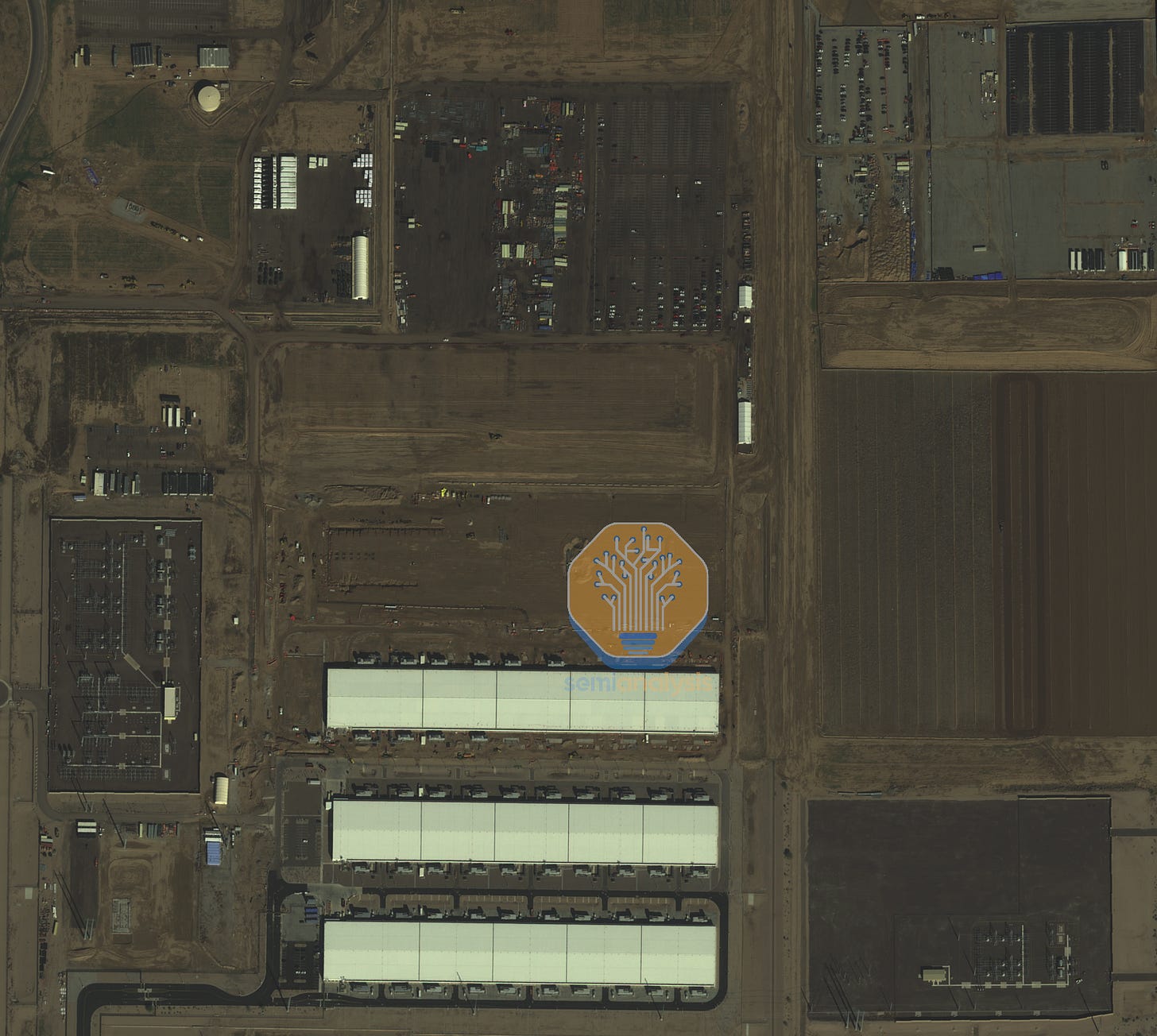
来源:SemiAnalysis 数据中心模型
Microsoft’s largest AI training region is in Phoenix – of which the above site is and will remain the largest portion, with an expansion to 10 buildings underway. Leveraging various sites where permitting has already been approved, Microsoft will in total self-build 24 datacenters with the reference design shown above. To further increase capacity, Microsoft’s leasing activity around Phoenix is very aggressive and will further contribute significantly to its footprint in the area. Not all these datacenters will be for training.
微软最大的人工智能训练区域位于凤凰城——上述地点是并将继续是最大的部分,目前正在扩建至 10 栋建筑。利用已获得许可的多个地点,微软将总共自建 24 个数据中心,采用上述参考设计。为了进一步增加容量,微软在凤凰城周围的租赁活动非常积极,将进一步显著增加其在该地区的足迹。并非所有这些数据中心都将用于训练。
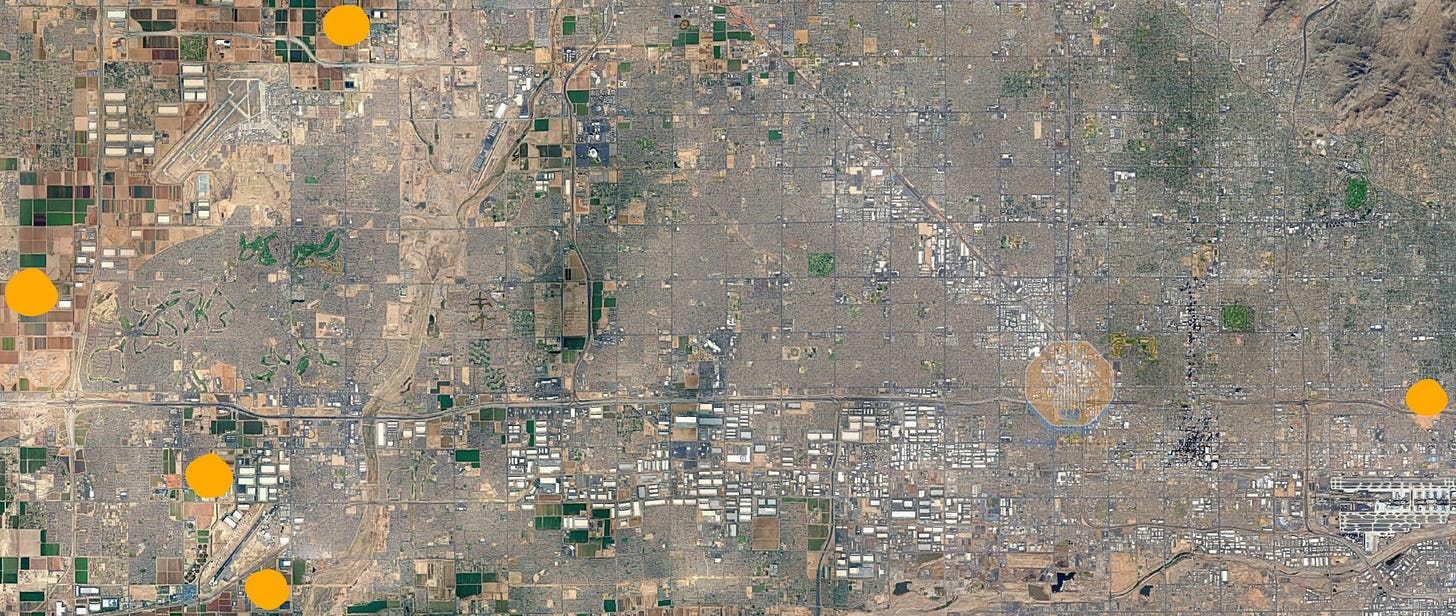
来源:SemiAnalysis 数据中心模型
But to beat Google at scale, Microsoft and OpenAI cannot rely on Microsoft’s old datacenter design. They are massively increasing the density of greenfield facilities via self-build in Milwaukee as well as across the US through partners such as Compass, QTS, Crusoe, Oracle, CoreWeave. In Milwaukee and Atlanta (via QTS), Microsoft is building the world’s most powerful single buildings, 100% liquid-cooled for next-gen AI hardware - more details here. The Wisconsin self-build mega-campus will be the single largest datacenter campus in Microsoft or Google’s entire footprint once fully constructed. Meta also has very aggressive single site plans underway.
但要在规模上击败谷歌,微软和 OpenAI 不能依赖微软旧的数据中心设计。他们正在通过自建在密尔沃基以及通过合作伙伴如 Compass、QTS、Crusoe、Oracle、CoreWeave 在美国各地大幅提高新建设施的密度。在密尔沃基和亚特兰大(通过 QTS),微软正在建设世界上最强大的单一建筑,100%液冷以支持下一代 AI 硬件 - 更多细节请见此处。威斯康星州的自建超级校园一旦完全建成,将成为微软或谷歌整个业务中最大的单一数据中心校园。Meta 也在进行非常激进的单一站点计划。
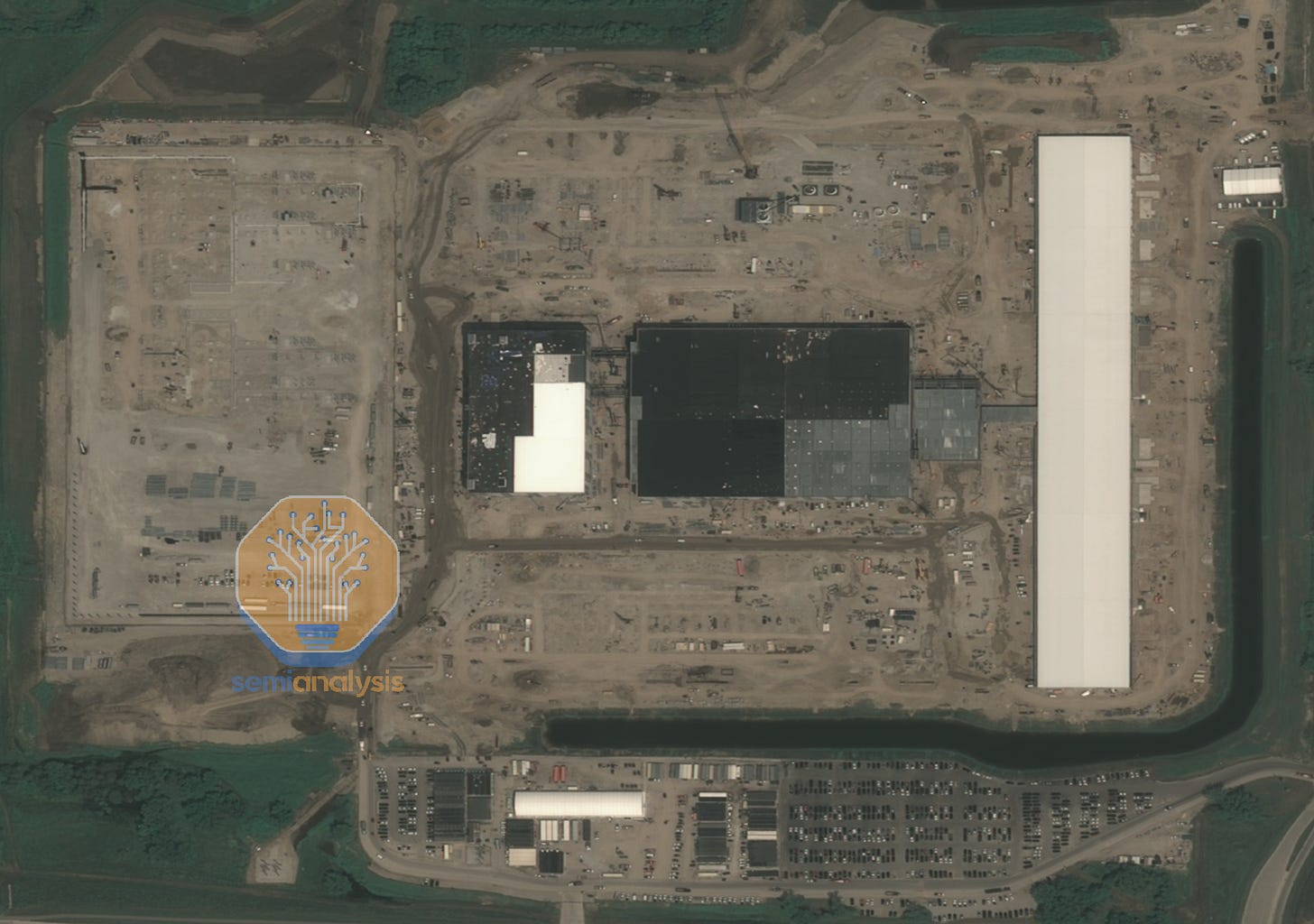
来源:SemiAnalysis 数据中心模型
Above is just a small snapshot of part of the grounds, but it is expanding rapidly.
以上只是场地的一小部分快照,但它正在迅速扩展。
Another portion of Microsoft and OpenAI’s giant infrastructure is Texas, through partnerships with Oracle + Crusoe, and CoreWeave + Core Scientific in Abilene and Denton.
微软和 OpenAI 庞大基础设施的另一部分位于德克萨斯州,通过与 Oracle + Crusoe,以及 CoreWeave + Core Scientific 在阿比林和登顿的合作。
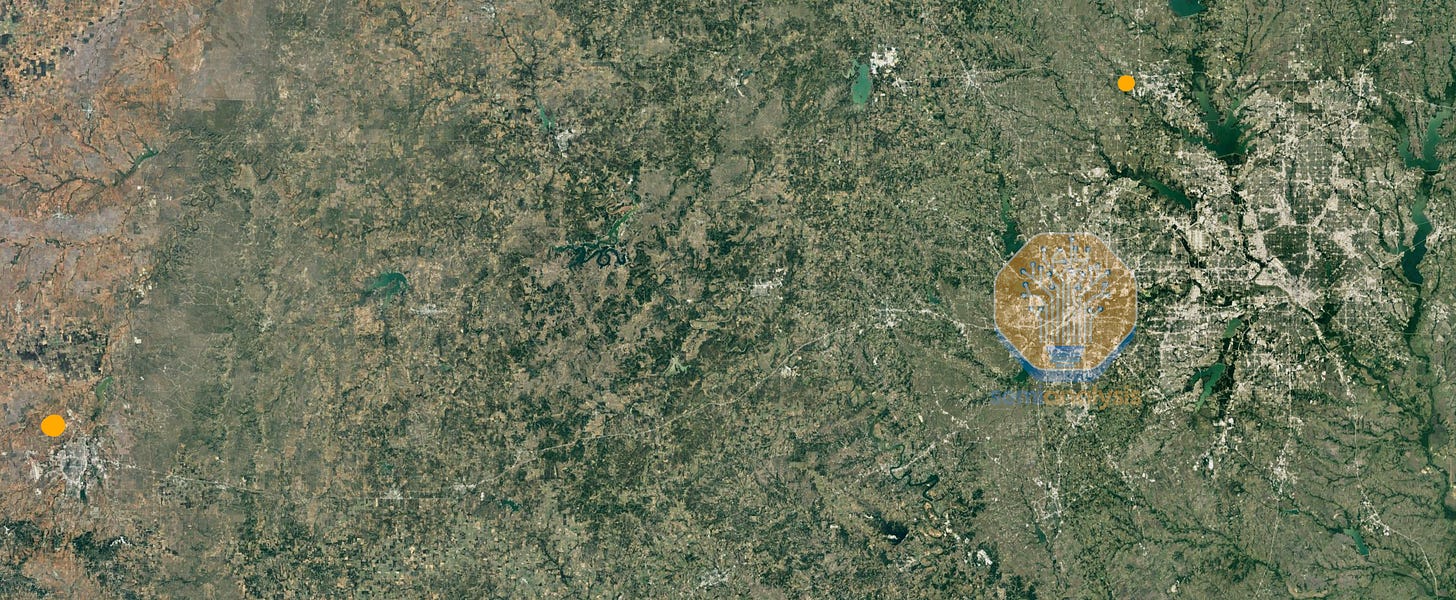
来源:SemiAnalysis 数据中心模型
Funnily, in the quest to build out AI clusters, Microsoft is engaging with crypto mining, with CoreWeave leasing an existing Core Scientific mining facility, and Oracle a Crusoe campus, who was also heavily in the crypto space historically. Bitcoin miners are used to high-density and high-power datacenters, and many of them have contracted large amounts of electricity as illustrated by the below data from Core Scientific’s 10-K, showing 1.2GW of contracted capacity across multiple sites. Timelines for building brand new datacenters are far longer than repurposing crypto sites.
有趣的是,在构建人工智能集群的过程中,微软正在参与加密货币挖矿,CoreWeave 租赁了现有的 Core Scientific 挖矿设施,而 Oracle 则租用了一个 Crusoe 校园,后者在历史上也与加密货币领域有着密切的联系。比特币矿工习惯于高密度和高功率的数据中心,他们中的许多人已经签订了大量电力合同,如下方 Core Scientific 的 10-K 数据所示,显示在多个地点的合同容量为 1.2GW。建设全新数据中心的时间远长于改造加密货币场所。
The company is massively pivoting towards datacenter colocation for AI and has struck a large deal with CoreWeave, covering 382MW of IT Power with a relatively short delivery timeline. CoreWeave will acquire the GB200 GPUs and rent them to Microsoft for OpenAI’s usage.
该公司正在大规模转向数据中心托管以支持人工智能,并与 CoreWeave 达成了一项大规模交易,涵盖 382MW 的 IT 电力,交付时间相对较短。CoreWeave 将收购 GB200 GPU 并将其租赁给微软供 OpenAI 使用。
We believe that the most significant site will be the mine located in Denton, Texas.
我们相信,最重要的地点将是位于德克萨斯州登顿的矿山。
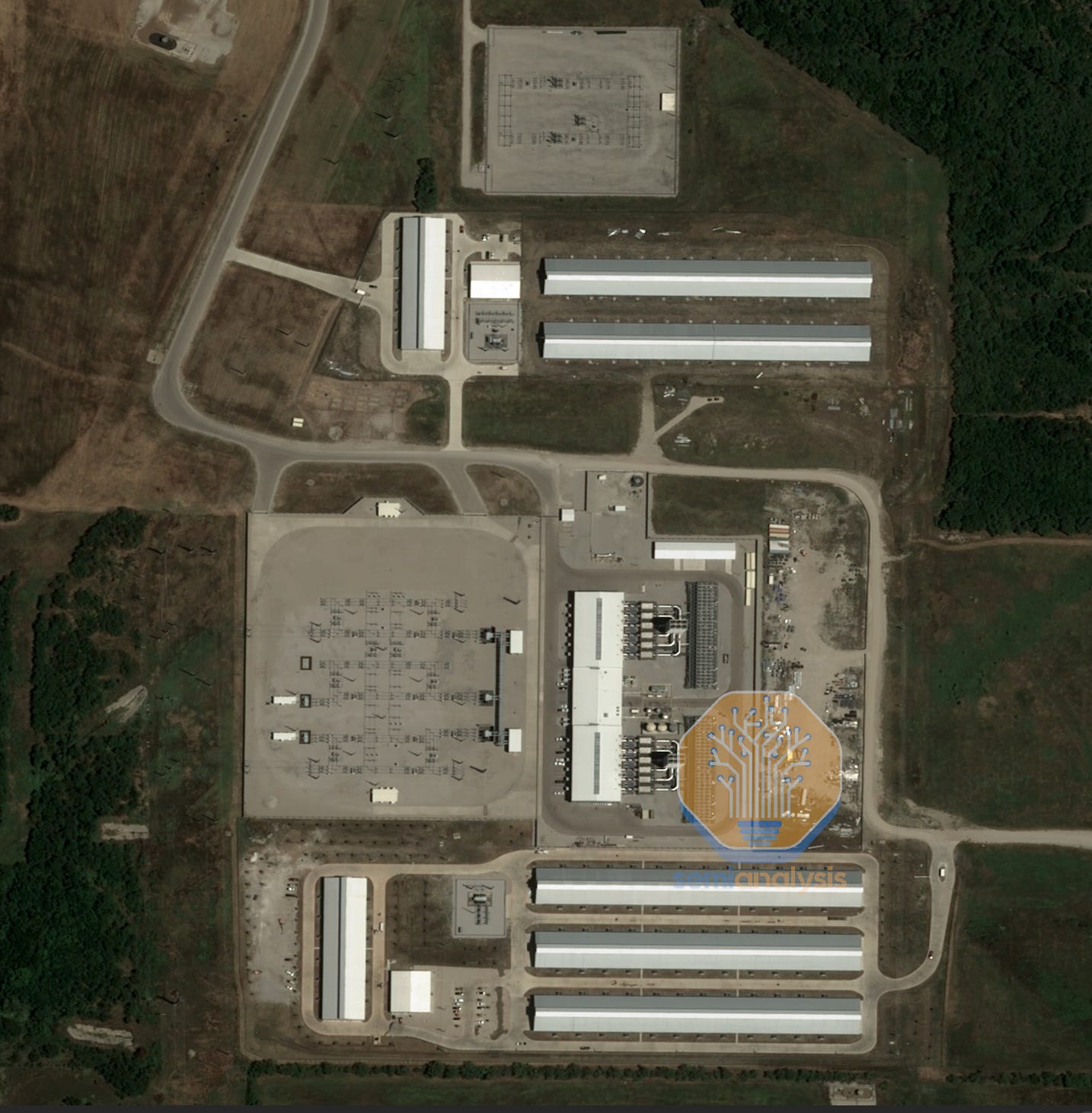
来源:SemiAnalysis 数据中心模型
Much like X.AI with their on-site generators, this datacenter also has plenty of power infrastructure. The site has a 225MW natural power plant sitting in the middle of all the crypto mines. The crypto mines will be torn down and heavily modified, replaced by datacenter-grade electrical and cooling systems. That said, this site will still be tremendously inefficient versus self-build datacenters with a PUE above 1.3.
The other significant campus is being developed by Crusoe in Abilene, Texas. The company, recognized for its innovative Flared Gas mining sites in North Dakota and Wyoming, is building a standard Gigawatt scale datacenter and leasing the first portion of it to Oracle who is then filling it with GPUs/networking and renting it out to OpenAI. Via real-time low-resolution satellites, we can see below how fast the campus has expanded. We have precise detailed quarterly historical and forecasted power data of over 5,000 datacenters in the Datacenter Model. This includes status of cluster buildouts for AI labs, hyperscalers, neoclouds, and enterprise.
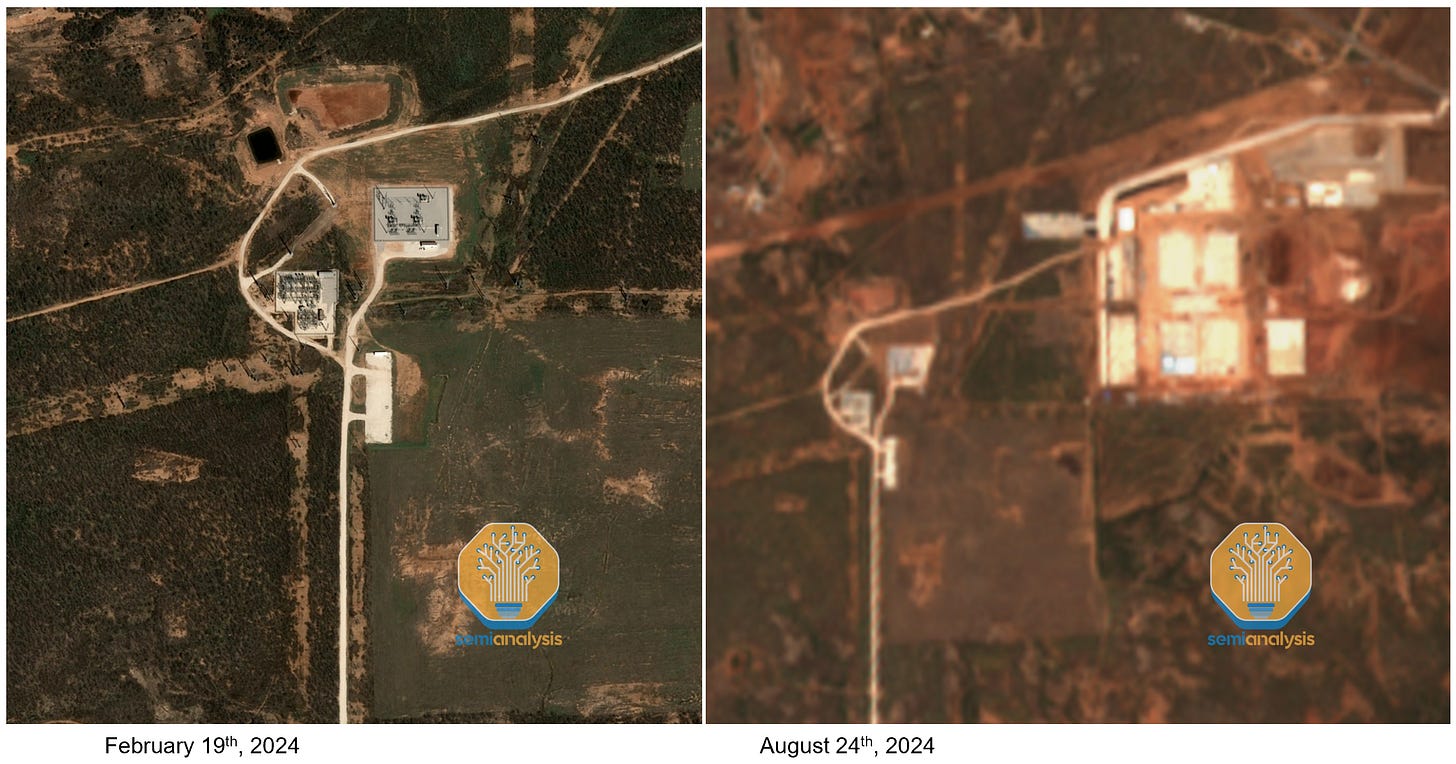
来源:SemiAnalysis 数据中心模型
There are also several other extremely large datacenters going up in other parts of the US such as below. We won’t walk through every single one on the newsletter for brevity, and competitive analysis, but the point is clear:
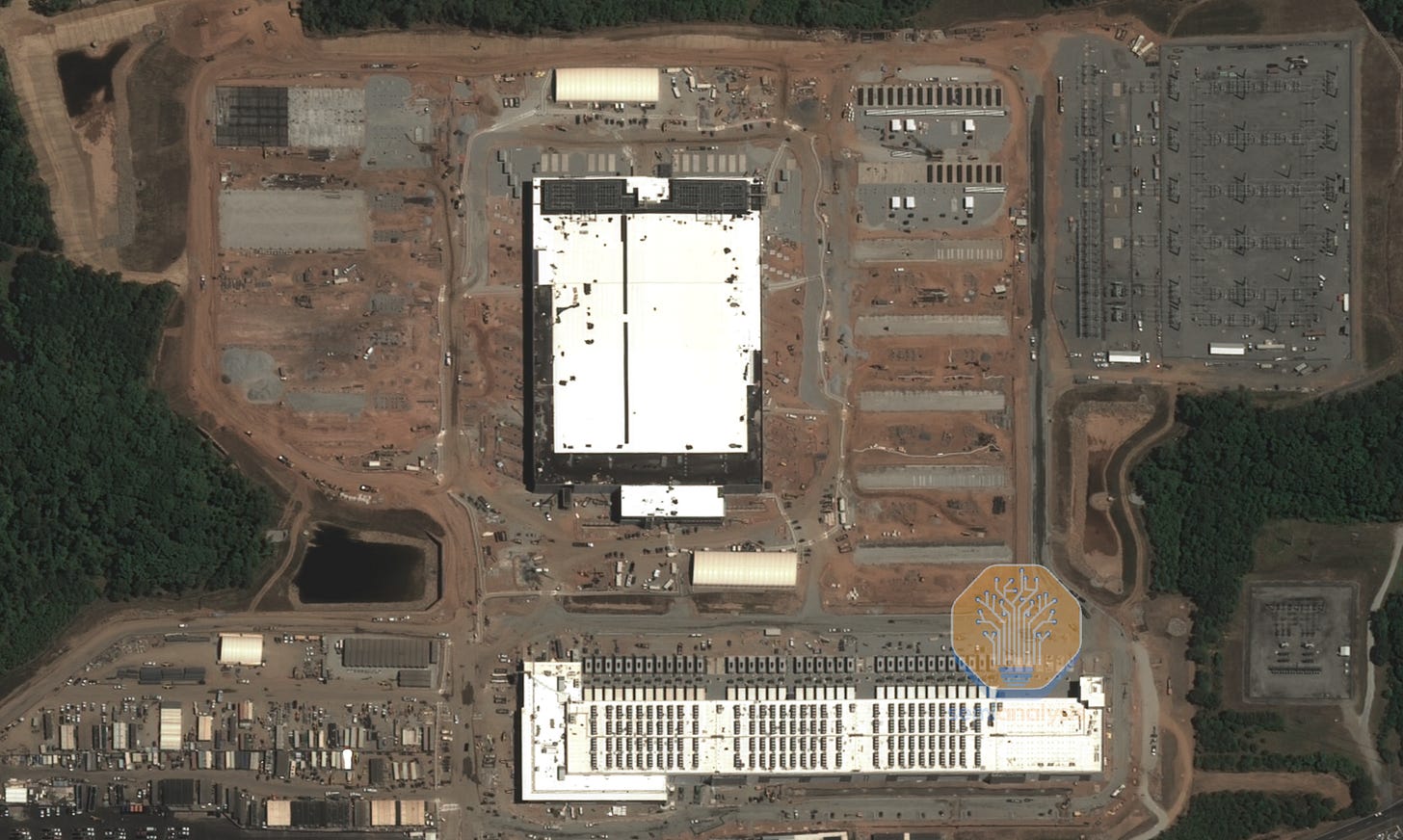
来源:SemiAnalysis 数据中心模型
Through a combination of ambitious self-build plans, aggressive leasing, large partnership and innovative ultra-dense designs, Microsoft will lead the AI training market by scale, with multiple GW-scale clusters.
The Multi-Gigawatt Monster Cluster
多吉瓦怪兽集群
Microsoft is looking to interconnect these multiple campuses to form a multi-GW monster training cluster. Fiber companies Lumen Technologies and Zayo are contracted and give us a few hints.
On July 24th, they announced a deal with Microsoft to interconnect multiple datacenters. A few days after, Lumen signed an agreement with Corning to reserve 10% of their capacity for the next two years. We think a few more similar deals are coming and that this could greatly expand Corning’s business.
Lumen Technologies (NYSE: LUMN) today announced it has secured $5 billion in new business driven by major demand for connectivity fueled by AI. Large companies across industry sectors are seeking to secure fiber capacity quickly, as this resource becomes increasingly valuable and potentially limited, due to booming AI needs. In addition, Lumen is in active discussions with customers to secure another $7 billion in sales opportunities to meet the increased customer demand.”
Lumen Technologies is a large telecom company with many segments, of which the most significant is Enterprise. Lumen works directly with businesses to address their connectivity needs, relying on its large fiber network, shown below.
As previously explained, this business suffers from a capacity utilization problem, with an extremely large amount of leased or owned fiber deployed but sitting idle – known as Dark Fiber, of which Lumen is one of the largest vendors in the US, alongside Zayo, AT&T and Crown Castle.
如前所述,该业务面临产能利用问题,部署了大量租赁或自有的光纤,但处于闲置状态——称为暗光纤,其中 Lumen 是美国最大的供应商之一,其他包括 Zayo、AT&T 和 Crown Castle。
The enterprise telecom business has also been challenging, as many enterprises have shifted their traffic to run over the internet due to falling internet pricing, which has hurt demand for MPLS (Multiprotocol Label Switching – a mainstay enterprise product that provides data connectivity between distant offices), leading to challenging pricing and underutilization of capacity resources. At the same time, the buyers of telecom capacity have become much more consolidated due to the rise of hyperscalers, and to make matters worse, these cloud-scale players prefer building their own telecom networks. This has meant that there is quite a bit of idle fiber capacity with many fibers pairs lit with far fewer wavelengths than possible and running on much older modulation schemes and slower data rates. There would be a huge opportunity to upgrade capacity should there be some impetus like a jump in customer demand, as seems possible due to AI training requirements.
企业电信业务也面临挑战,因为许多企业由于互联网价格下降而将流量转移到互联网,这影响了 MPLS(多协议标签交换 - 一种提供远程办公室之间数据连接的主要企业产品)的需求,导致定价困难和产能资源的闲置。同时,由于超大规模云服务商的崛起,电信容量的买家变得更加集中,更糟糕的是,这些云规模的参与者更倾向于建立自己的电信网络。这意味着许多光纤对的闲置容量相当可观,点亮的光纤对的波长远低于可能的数量,并且运行在更旧的调制方案和较慢的数据速率上。如果出现一些推动因素,比如客户需求的激增(由于 AI 训练需求似乎有可能出现),将会有巨大的升级容量的机会。
Maintaining such a large infrastructure requires vast capital expenditures, and like many of its peers, Lumen suffers from a cash-flow issue and a large debt burden. The company had close to $20B of financial debt and hardly generates any Free Cash Flow, with a stable to declining revenue growth trend.
维持如此庞大的基础设施需要巨额的资本支出,像许多同行一样,Lumen 面临现金流问题和巨大的债务负担。该公司的金融债务接近 200 亿美元,几乎没有产生任何自由现金流,收入增长趋势稳定或下降。
The rise of multi-campus AI Training changes the equation, with massive bandwidth requirements. Microsoft is the key customer behind the $5B deal, with another $7B deal that could be in the works.
多校园人工智能培训的兴起改变了局面,带来了巨大的带宽需求。微软是这笔 50 亿美元交易背后的关键客户,还有一笔可能正在进行中的 70 亿美元交易。
The reason that a company like Lumen landed such a deal (and why it is also mentioning that there is potentially $7B worth of deals that could come) is due to their extensive unutilized fiber network. All that idle capacity and existing routes allow hyperscalers to build large-scale, ultra-high-bandwidth networks in a cost-efficient manner. Possibly even more important is time to market: leveraging that infrastructure accelerates what would otherwise take various years, especially if digging ad-hoc tunnels was required.
像 Lumen 这样的公司能够达成如此交易(并且提到可能还有价值 70 亿美元的交易)是因为他们拥有广泛的未利用光纤网络。所有这些闲置的容量和现有的线路使得超大规模公司能够以成本高效的方式构建大规模、超高带宽的网络。可能更重要的是市场时间:利用这些基础设施加速了本来需要数年的过程,尤其是在需要挖掘临时隧道的情况下。
For Lumen, the economics of the $5B deals are as follows:
对于 Lumen 来说,50 亿美元交易的经济情况如下:
The commercial structure is the IRU (Indefeasible Right of Use), standard in the fiber industry, and essentially akin to a capitalized lease. The typical duration of these agreements is 20 years.
商业结构是不可撤销使用权(IRU),这是光纤行业的标准,基本上类似于资本化租赁。这些协议的典型期限为 20 年。85-90% of the deal value is related to infrastructure, while the remaining portion is operations and maintenance, and power and hosting.
交易价值的 85-90%与基础设施相关,其余部分则与运营和维护以及电力和托管有关。Lumen estimates a 30-35% cash margin on the deal, meaning a pre-tax profit of ~$1.5B.
Lumen 预计该交易的现金利润率为 30-35%,这意味着税前利润约为 15 亿美元。A large portion of Infrastructure will trigger cash prepayments over the first 3-4 years of the contract, while the remaining portion will ramp as milestones are achieved and be charged as an annual fee over the life of the contract.
基础设施的大部分将在合同的前 3-4 年内触发现金预付款,而剩余部分将在里程碑达成时逐步增加,并在合同的整个生命周期内作为年度费用收取。
The deal triggered a massive upgrade to Lumen’s annual Free Cash Flow guidance, despite rising CAPEX and OPEX (lowered EBITDA) also related to the deal.
这笔交易引发了 Lumen 年度自由现金流指引的大幅升级,尽管与该交易相关的资本支出和运营支出(息税折旧摊销前利润下降)也在上升。
This could be just the start. There will be a meaningful ramp in telecom next year, and this sleepy telecom player is clearly guiding to a big boost to revenues. Optic companies are starting to perk up at the opportunity, but we believe that the actual impact will surprise investors and companies in the space alike. Take the entire market for switches, routing, and WAN, it’s only $75B, so $5-10B of incremental investment from just one player will move the needle.
这可能只是个开始。明年电信行业将会有显著的增长,而这个沉寂的电信参与者显然在指引收入的大幅提升。光纤公司开始对这个机会感到兴奋,但我们相信,实际影响将会让投资者和行业内的公司感到惊讶。整个交换机、路由和广域网市场仅为 750 亿美元,因此仅来自一个参与者的 50-100 亿美元的增量投资将会产生显著影响。
We believe there is a wave of more than $10B of telecom capex specifically for multi-datacenter training coming. That is all incremental. At the same time, the telecom market is troughing right now at a cyclical low. This is a new incremental driver paired with a cyclical rebound.
我们相信,针对多数据中心培训的电信资本支出将超过 100 亿美元。这都是增量支出。同时,电信市场目前正处于周期性低谷。这是一个新的增量驱动因素,伴随着周期性反弹。
Companies that Benefit 受益的公司
In addition to Corning and Lumen who we have directly discussed, Fabrinet also has strength in datacenter interconnect products, in particular its 400ZR product lines, which reached 10% of optical revenue in 4Q of the fiscal year ended June 2024. Fabrinet has significant telecom exposure well beyond just 400ZR, with telecom making up nearly 40% of total revenue in its 4Q FY24. It also has a strong datacom transceiver portfolio, manufacturing Nvidia’s 800G transceiver used to connect GPUs within the compute fabric / back-end network.
除了我们直接讨论的康宁和 Lumen,Fabrinet 在数据中心互连产品方面也具有优势,特别是其 400ZR 产品线,在截至 2024 年 6 月的财年第四季度达到了光学收入的 10%。Fabrinet 在电信领域的曝光率远超 400ZR,电信在其 2024 财年第四季度的总收入中占近 40%。它还拥有强大的数据通信收发器产品组合,制造用于连接计算架构/后端网络中 GPU 的 Nvidia 800G 收发器。
The continued ramp in ZR optics is a key reason the company expects sequential growth in the telecom business into the following quarter. Fabrinet will benefit from ZR optics growing from ~10% of revenue to over 20% of revenue in time. Fabrinet is the only dedicated contract manufacturer for optical systems and components, the “TSMC” in this industry comparison, and benefits primarily from volume and has a strong competitive position.
ZR 光学的持续增长是公司预计电信业务在下一个季度实现环比增长的关键原因。Fabrinet 将从 ZR 光学的收入占比从约 10%增长到超过 20%中受益。Fabrinet 是光学系统和组件的唯一专门合同制造商,在这个行业中被比作“台积电”,主要受益于产量,并拥有强大的竞争地位。
Cisco is Fabrinet’s second largest customer after Nvidia, at 13% of sales in FY24, and along with other telecom clients, give Fabrinet significant torque coming from the telecom side of their business. Moreover, they just landed Ciena as a customer, which is an indication of the ramp up of volumes that Ciena could see next year. In the past, Lumentum and Infinera have also been greater than 10% customers and a turnaround at those companies will also help drive telecom revenue growth.
思科是 Fabrinet 的第二大客户,仅次于 Nvidia,占 FY24 销售额的 13%。与其他电信客户一起,给 Fabrinet 带来了来自电信业务的显著推动。此外,他们刚刚获得了 Ciena 作为客户,这表明 Ciena 明年可能会看到的业务量增长。过去,Lumentum 和 Infinera 也曾是超过 10%的客户,这些公司的转机也将有助于推动电信收入的增长。
Lumentum is another telecom-exposed company that is expecting a meaningful sequential quarter on quarter improvement in revenue primarily driven by stronger demand for components for ZR/ZR+ optics, ROADM and C+L band products.
Lumentum 是另一家与电信相关的公司,预计在收入方面将实现有意义的季度环比改善,主要受对 ZR/ZR+ 光学组件、ROADM 和 C+L 波段产品需求增强的推动。
Coherent, unlike Lumentum, offered a more tepid outlook, expecting the telecom market to remain weak overall in the near term despite calling out strength in its 400ZR+ transceivers business. Coherent has continued to suffer from ongoing telecom equipment inventory issues, with a decline in telecom revenue of 6% quarter on quarter and 38% year on year. However, their forward guidance gives credence that the bottom in telecom is in sight. Despite Coherent’s heritage as a successor company to Finisar, its current telecom exposure has gotten quite small as a percent of revenue due to the amalgamation of various non-optical businesses – we estimate telecom stands at only 13% of total revenue in the latter half of the fiscal year ended June 2024. We think Lumentum has a better business mix than Coherent, and a much better capital structure. On the other hand, Coherent’s debt could juice equity returns should it continue to deliver on datacom growth and land some growth in telecom.
科赫伦(Coherent)与 Lumentum 不同,提供了更为温和的前景,预计尽管其 400ZR+收发器业务表现强劲,但电信市场在短期内整体仍将保持疲软。科赫伦持续受到电信设备库存问题的困扰,电信收入环比下降 6%,同比下降 38%。然而,他们的前瞻性指引表明电信市场的底部即将到来。尽管科赫伦作为 Finisar 的继承公司,其当前的电信业务在收入中所占比例已大幅缩小,主要是由于各种非光学业务的合并——我们估计在截至 2024 年 6 月的财年下半年,电信业务仅占总收入的 13%。我们认为 Lumentum 的业务组合优于科赫伦,资本结构也更佳。另一方面,如果科赫伦继续在数据通信增长上取得进展并在电信领域实现一些增长,其债务可能会提升股本回报。
Ciena and Cisco are both telecom heavyweights that not only produce much of the traditional telecom equipment such as line cards/transponders, routers, mux/demux and ROADMs. Cisco has a broader portfolio of products along with a few software businesses, while Ciena has more of a pure play exposure to telecom equipment. Infinera is also telecom exposed but will shortly be folded into the Nokia mothership in a pending acquisition.
Ciena 和思科都是电信巨头,不仅生产许多传统电信设备,如线路卡/收发器、路由器、复用/解复用器和光路开关(ROADM)。思科的产品组合更广泛,还有一些软件业务,而 Ciena 则更专注于电信设备。Infinera 也与电信相关,但将在即将进行的收购中被纳入诺基亚母公司。
Ciena in our view has the most exposure of the Telecom OEMs as they are much more focused specifically on the telecom network hardware introduced above. Ciena has been calling out strength from cloud customers, and now they are starting to see huge orders for next year’s buildouts, having specifically called out orders directly relate to AI traffic demands in their 2Q of FY24 earnings call. Though its mainstay is traditional telecom network equipment (Transponders, ROADMs, etc) as opposed to datacenter interconnect, it highlighted 18 400ZR+ and 800ZR+ order wins, with many of a strategic nature. ZR optics are an additive opportunity to Ciena’s business given its exposure has mostly been on metro and long-haul networks as opposed to data center interconnect. We believe that they have a very strong position across all of these market segments, and that telecom networks designed to facilitate AI training will have a much higher link density. There's potential for both a content and volume gain story at Ciena, and of all the telecom OEM players, they have most proportional exposure to AI telecom network buildouts across all ranges and deployments.
在我们看来,Ciena 在电信 OEM 中暴露的风险最大,因为他们更加专注于上述提到的电信网络硬件。Ciena 一直在强调来自云客户的强劲需求,现在他们开始看到明年建设的巨大订单,特别是在 2024 财年第二季度的财报电话会议中提到与 AI 流量需求直接相关的订单。尽管其主要业务是传统电信网络设备(转发器、光路交换机等),而不是数据中心互联,但他们强调赢得了 18 个 400ZR+和 800ZR+的订单,其中许多具有战略性质。ZR 光学是 Ciena 业务的一个附加机会,因为他们的曝光主要集中在城域网和长途网络,而不是数据中心互联。我们相信,他们在所有这些市场细分中都具有非常强的地位,旨在促进 AI 训练的电信网络将具有更高的链路密度。Ciena 有潜力在内容和数量上实现增长,在所有电信 OEM 参与者中,他们在 AI 电信网络建设方面的相对曝光率最高,涵盖所有范围和部署。
Cisco highlighted double digit orders growth in hyperscale customer revenue in the second half of the fiscal year ended July 2024, offsetting weakness in service provider (i.e. telecom operators). It has also reached $1B of AI orders, mostly Ethernet and optics related products, and expects an additional $1B of orders related to AI in FY25. Although there was no mention of ZR optics or datacenter interconnect in detail this past quarter, Cisco had purchased Acacia in 2021 to be well positioned in Coherent DSPs and ZR optics, among other products, and in theory should have had exposure, but they have hardly mentioned any opportunities from ZR optics in the past few earnings calls. The other consideration is Cisco’s large revenue base of $53.8B (FY24 full year revenue). So, any surge in AI datacenter interconnect related demand will be far less impactful to revenue on a percentage basis than other telecom OEMs or component/system suppliers.
思科在截至 2024 年 7 月的财年下半年强调了超大规模客户收入的双位数订单增长,抵消了服务提供商(即电信运营商)的疲软。它还达成了 10 亿美元的人工智能订单,主要是与以太网和光学相关的产品,并预计在 2025 财年将有额外的 10 亿美元与人工智能相关的订单。尽管在过去一个季度中没有详细提及 ZR 光学或数据中心互连,思科在 2021 年收购了 Acacia,以便在相干数字信号处理器和 ZR 光学等产品中占据良好位置,理论上应该有相关的曝光,但在过去几次财报电话会议中几乎没有提到 ZR 光学的任何机会。另一个考虑因素是思科的庞大收入基础为 538 亿美元(2024 财年全年收入)。因此,任何与人工智能数据中心互连相关需求的激增在百分比上对收入的影响将远低于其他电信 OEM 或组件/系统供应商。
Last is Marvell. Marvell’s acquisition of Inphi bought them a dominating position in PAM4 DSPs as well as a portfolio of Coherent DSPs (Deneb, Orion and Canopus).
最后是 Marvell。Marvell 收购 Inphi 使其在 PAM4 DSPs 中占据了主导地位,并拥有了一系列相干 DSP(Deneb、Orion 和 Canopus)。
We think coherent DSPs were a modest part of the Inphi / Marvell business historically compared to PAM4. No longer. Marvell’s ZR optics business is not only anchored by its Coherent DSP portfolio but also its COLORZ, COLORZ 400ZR/ZR+ and COLORZ 800ZR/ZR+ data center interconnect transceivers. This ZR business is on the path to grow considerably and become quite meaningful even when compared to the sizeable PAM4 business. ZR transceivers have far higher ASPs than IMDD transceivers and will continue to see considerable volume growth.
我们认为,相较于 PAM4,历史上相干 DSP 在 Inphi / Marvell 业务中占比 modest。现在情况不同了。Marvell 的 ZR 光学业务不仅依赖于其相干 DSP 产品组合,还包括 COLORZ、COLORZ 400ZR/ZR+和 COLORZ 800ZR/ZR+数据中心互连收发器。这个 ZR 业务正在快速增长,并且在与规模庞大的 PAM4 业务相比时变得相当重要。ZR 收发器的平均销售价格远高于 IMDD 收发器,并将继续看到显著的销量增长。
Marvell has a stronger competitive advantage in this product and already had a very promising go to market for the COLORZ family with a sizeable win at a major hyperscaler and multiple years of meaningful volume shipment into that customer that continues to grow considerably and Marvell has added multiple additional customers for that product. This impact will far outweigh any potential near-term LRO issues, and Marvell has the most direct revenue growth at the highest margins from this theme.
Marvell 在该产品上具有更强的竞争优势,并且已经为 COLORZ 系列制定了非常有前景的市场推广策略,在一家主要的超大规模客户那里获得了可观的胜利,并且与该客户的多年的重要出货量持续显著增长,Marvell 还为该产品增加了多个额外客户。这一影响将远远超过任何潜在的短期 LRO 问题,Marvell 在这一主题中拥有最直接的收入增长和最高的利润率。
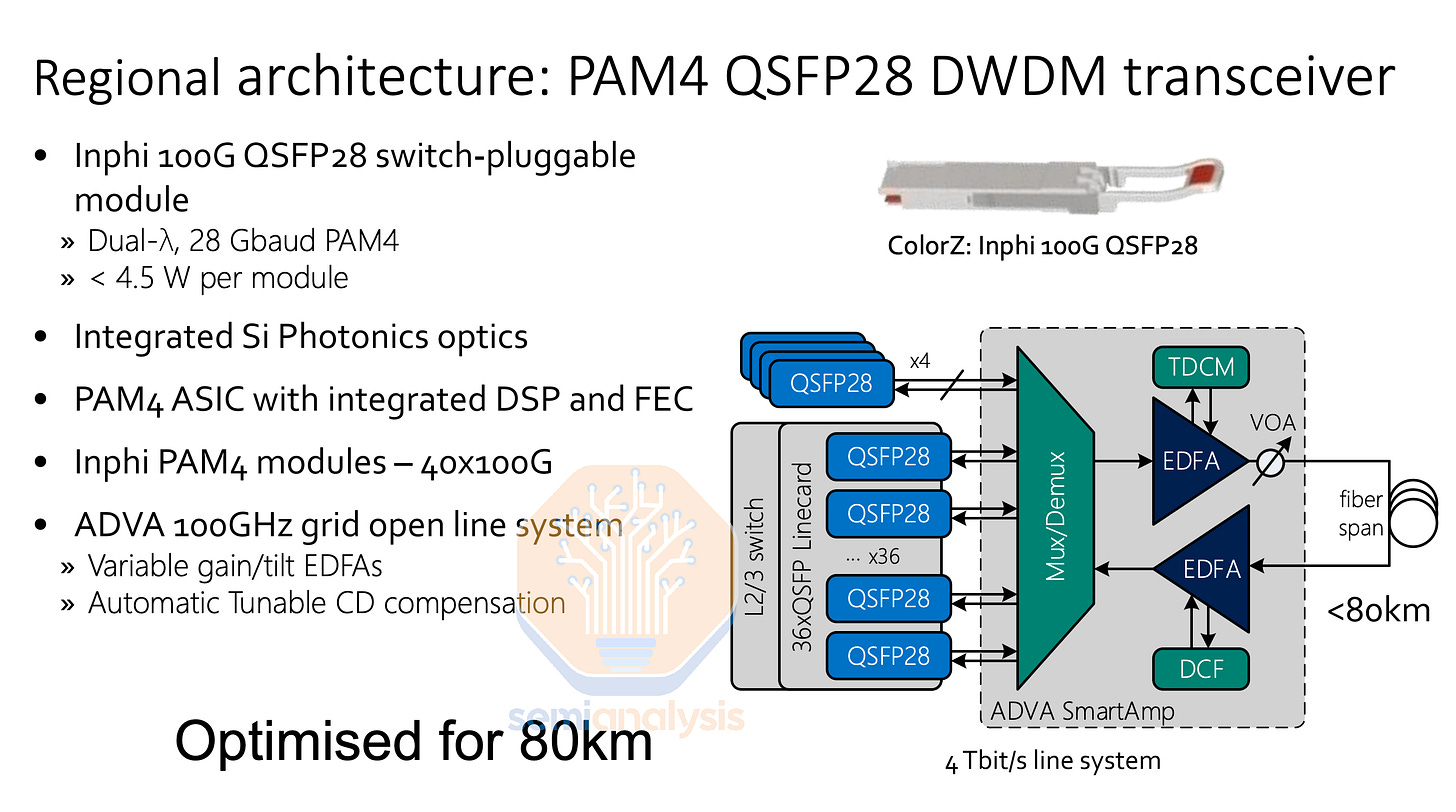