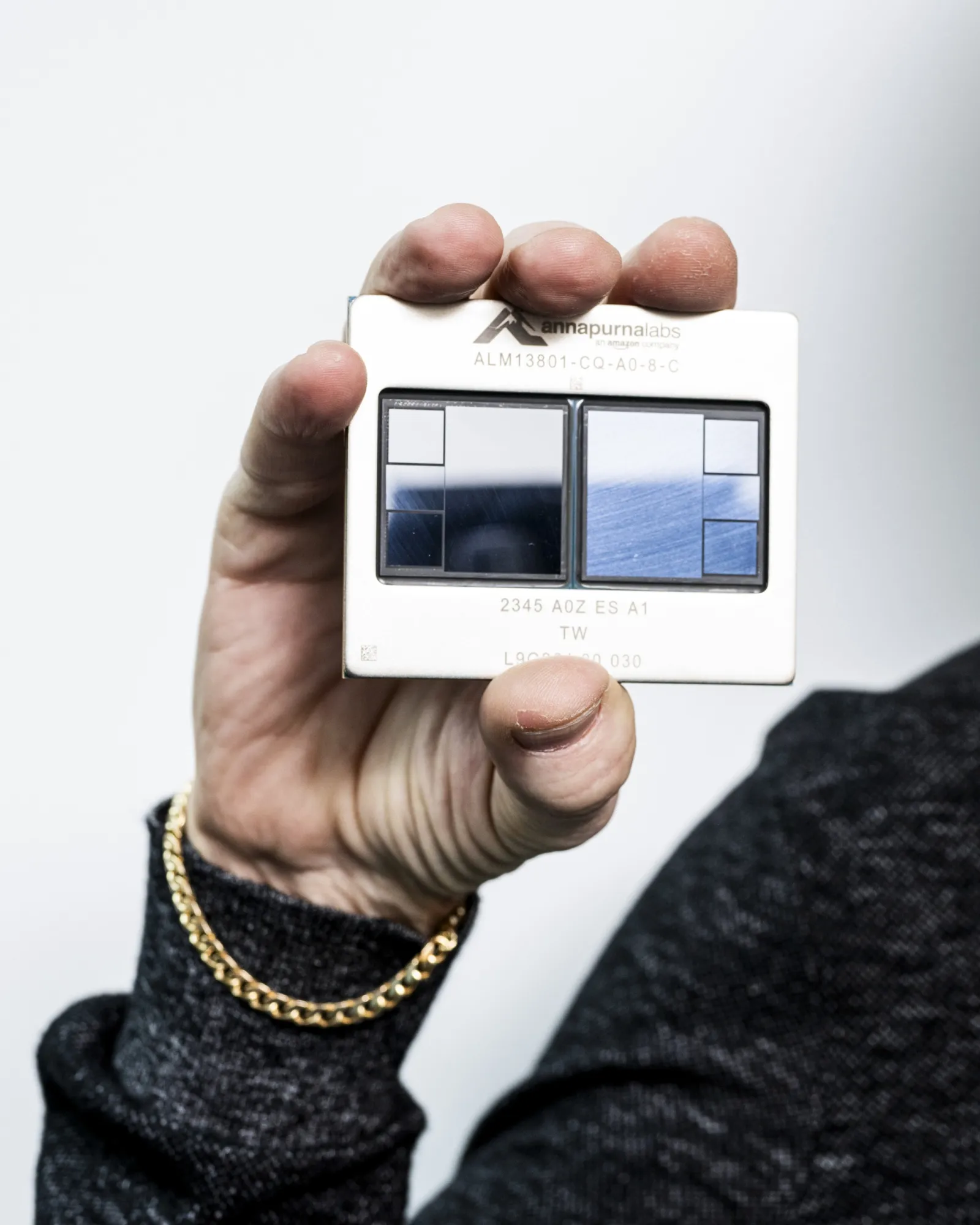
James Hamilton holding the Trainium2 chip.
Photographer: Chona Kasinger/Bloomberg詹姆斯·汉密尔顿持有 Trainium2 芯片。
摄影师:Chona Kasinger/彭博社Amazon’s Moonshot Plan to Rival Nvidia in AI Chips
亚马逊的登月计划在人工智能芯片领域与英伟达竞争
The cloud computing giant won’t dislodge the incumbent anytime soon but is hoping to reduce its reliance on the chipmaker.
这家云计算巨头不会很快取代现有企业,但希望减少对芯片制造商的依赖。
In a bland north Austin neighborhood dominated by anonymous corporate office towers,
亚马逊的实用工程实验室有一排长工作台,俯瞰着德克萨斯州首府蓬勃发展的郊区。这个地方有点乱。印刷电路板、冷却风扇、电缆和网络设备散落在不同组装状态的工作站周围,其中一些沾满了用于将芯片连接到防止其过热的组件的导热膏。你希望在初创公司中看到一种自力更生的氛围,而不是在市值超过 2 万亿美元的公司中。Amazon.com Inc. engineers are toiling away on one of the tech industry’s most ambitious moonshots: loosening Nvidia Corp.’s grip on the $100-billion-plus market for artificial intelligence chips.
在奥斯汀北部一个平淡无奇的社区,周围都是匿名的公司办公楼,亚马逊公司的工程师们正在努力实现科技行业最雄心勃勃的登月计划之一:放松英伟达公司对价值超过 1000 亿美元的人工智能市场的控制。芯片。
Amazon’s utilitarian engineering lab contains rows of long work benches overlooking the Texas capital’s mushrooming suburbs. The place is kind of a mess. Printed circuit boards, cooling fans, cables and networking gear are strewn around workstations in various states of assembly, some muddied with the thermal paste used to connect chips to the components that keep them from overheating. There’s a bootstrapping vibe you’d expect to see at a startup not a company with a market cap exceeding $2 trillion.
亚马逊的实用工程实验室有一排长工作台,俯瞰着德克萨斯州首府蓬勃发展的郊区。这个地方有点乱。印刷电路板、冷却风扇、电缆和网络设备散落在不同组装状态的工作站周围,其中一些沾满了用于将芯片连接到防止其过热的组件的导热膏。你希望在初创公司中看到一种自力更生的氛围,而不是在市值超过 2 万亿美元的公司中。
The engineers who work here think nothing of running to Home Depot for a drill press and are happy to learn subjects outside their area of expertise if doing so will speed things up. Years into a scramble to create machine learning chips from scratch, they have found themselves on the hook to roll out an Nvidia fighter as quickly as they can. This is not about raw horsepower. It’s about building a simple, reliable system that can quickly turn Amazon data centers into humongous AI machines.
在这里工作的工程师认为去家得宝购买钻床没什么大不了的,并且很乐意学习他们专业领域之外的科目,如果这样做可以加快速度的话。多年来,他们一直在努力从头开始制造机器学习芯片,但他们发现自己陷入了尽快推出 Nvidia 战斗机的困境。这与原始马力无关。它是关于构建一个简单、可靠的系统,可以快速将亚马逊数据中心转变为巨大的人工智能机器。
位于奥斯汀的亚马逊实用工程实验室的工程师。摄影师:Sergio Flores/彭博社
你希望在初创公司中看到一种自力更生的氛围,而不是在市值超过 2 万亿美元的公司中。摄影师:Sergio Flores/彭博社
Rami Sinno, a gregarious Lebanese-born engineer who has worked in the chip industry for decades, is in charge of chip design and testing. He helped create the first two generations of Amazon AI semiconductors and is now rushing to get the latest iteration, Trainium2, running reliably in data centers by the end of the year. “What keeps me up at night is, how do I get there as quickly as possible,” Sinno says.
Rami Sinno 是一位出生于黎巴嫩的工程师,在芯片行业工作了几十年,负责芯片设计和测试。他帮助创建了前两代亚马逊人工智能半导体,现在正忙着让最新版本 Trainium2 于今年年底前在数据中心可靠运行。 “让我彻夜难眠的是,我如何尽快到达那里,”辛诺说。
In the past two years, Nvidia has transformed from a niche chipmaker to the main supplier of the hardware that enables generative AI, a distinction that has made the company the world’s largest by market value. Nvidia processors cost tens of thousands of dollars apiece and, thanks to overwhelming demand, are hard to get hold of. Last week, after reporting earnings, the chipmaker told investors that demand for its latest hardware will outstrip supply for several quarters — deepening the crunch.
在过去的两年里,英伟达已经从一家小众芯片制造商转变为支持生成式人工智能的硬件的主要供应商,这一优势使该公司成为全球市值最大的公司。 Nvidia 处理器每颗售价数万美元,而且由于需求旺盛,很难买到。上周,在公布财报后,这家芯片制造商告诉投资者,对其最新硬件的需求将在几个季度内超过供应,从而加剧了紧缩状况。
Nvidia’s biggest customers — cloud providers like Amazon Web Services, Microsoft Corp.’s Azure and Alphabet Inc.’s Google Cloud Platform — are eager to reduce their reliance on, if not replace, Nvidia chips. All three are cooking up their own silicon, but Amazon, the largest seller of rented computing power, has deployed the most chips to date.
Nvidia 最大的客户——亚马逊网络服务、微软公司的 Azure 和 Alphabet Inc. 的谷歌云平台等云提供商——渴望减少甚至取代 Nvidia 芯片的依赖。这三个公司都在开发自己的芯片,但最大的租赁计算能力销售商亚马逊迄今为止部署了最多的芯片。
In many ways, Amazon is ideally situated to become a power in AI chips. Fifteen years ago, the company invented the cloud computing business and then, over time, started building the infrastructure that sustains it. Reducing its reliance on one incumbent after another, including Intel Corp., Amazon ripped out many of the servers and network switches in its data centers and replaced them with custom-built hardware. Then, a decade ago, James Hamilton, a senior vice president and distinguished engineer with an uncanny sense of timing, talked Jeff Bezos into making chips.
从很多方面来说,亚马逊都具备成为人工智能芯片巨头的理想条件。十五年前,该公司发明了云计算业务,然后随着时间的推移,开始构建支撑云计算业务的基础设施。为了减少对英特尔公司等现有企业的依赖,亚马逊拆除了数据中心的许多服务器和网络交换机,并用定制硬件取而代之。然后,十年前,高级副总裁兼杰出工程师詹姆斯·汉密尔顿(James Hamilton)以不可思议的时机感说服杰夫·贝佐斯(Jeff Bezos)制造芯片。
When OpenAI’s ChatGPT kicked off the generative AI age two years ago, Amazon was widely considered an also-ran, caught flat-footed and struggling to catch up. It has yet to produce its own large language model that is seen as competitive with the likes of ChatGPT or Claude, built by Anthropic, which Amazon has backed to the tune of $8 billion. But the cloud machinery Amazon has built — the custom servers, switches, chips — has positioned Chief Executive Officer Andy Jassy to open an AI supermarket, selling tools for businesses that want to use models built by other outfits and chips for companies that train their own AI services.
两年前,当 OpenAI 的 ChatGPT 拉开了生成式 AI 时代的序幕时,亚马逊被广泛认为是一个失败者,措手不及,奋力追赶。它尚未产生自己的大型语言模型,该模型被认为可以与 Anthropic 构建的 ChatGPT 或 Claude 等语言模型竞争,亚马逊已为其提供了 80 亿美元的支持。但亚马逊打造的云机器——定制服务器、交换机、芯片——让首席执行官安迪·贾西(Andy Jassy)开设了一家人工智能超市,为那些想要使用其他公司构建的模型的企业销售工具,为那些训练自己的公司销售芯片。人工智能服务。
After almost four decades in the business, Hamilton knows taking Amazon’s chip ambitions to the next level won’t be easy. Designing reliable AI hardware is hard. Maybe even harder is writing software capable of making the chips useful to a wide range of customers. Nvidia gear can smoothly handle just about any artificial intelligence task. The company is shipping its next-generation chips to customers, including Amazon, and has started to talk up the products that will succeed them a year from now. Industry observers say Amazon isn’t likely to dislodge Nvidia anytime soon.
在从事该行业近四十年后,汉密尔顿知道将亚马逊的芯片雄心提升到一个新的水平并不容易。设计可靠的人工智能硬件很困难。也许更困难的是编写能够使芯片对广泛客户有用的软件。 Nvidia 设备可以顺利处理几乎任何人工智能任务。该公司正在向包括亚马逊在内的客户运送下一代芯片,并开始讨论一年后将接替他们的产品。行业观察人士表示,亚马逊不太可能很快取代英伟达。
詹姆斯·汉密尔顿表示,亚马逊可以与英伟达“面对面”竞争。摄影师:Chona Kasinger/彭博社
Still, time and again, Hamilton and Amazon’s teams of engineers have demonstrated their capacity to solve big technical problems on a tight budget. “Nvidia is a very, very competent company doing excellent work, and so they’re going to have a good solution for a lot of customers for a long time to come,” Hamilton says. “We’re strongly of the view that we can produce a part that competes with them toe to toe.”
尽管如此,汉密尔顿和亚马逊的工程师团队还是一次又一次地证明了他们在预算紧张的情况下解决重大技术问题的能力。 “Nvidia 是一家非常非常有能力的公司,工作非常出色,因此他们将在未来很长一段时间内为很多客户提供良好的解决方案,”汉密尔顿说。 “我们坚信我们可以生产出与他们面对面竞争的零件。”
Hamilton joined Amazon in 2009 after stints at International Business Machines Corp. and Microsoft. An industry icon who got his start repairing luxury cars in his native Canada and commuted to work from a 54-foot boat, Hamilton signed on at an auspicious time. Amazon Web Services had debuted three years earlier, singlehandedly creating an industry for what came to be known as cloud computing services. AWS would soon start throwing off gobs of cash, enabling Amazon to bankroll a number of big bets.
汉密尔顿在国际商业机器公司和微软工作过一段时间后,于 2009 年加入亚马逊。行业偶像汉密尔顿在家乡加拿大开始修理豪华汽车,并乘坐一艘 54 英尺长的船上下班,他在一个有利的时机签约了。亚马逊网络服务于三年前推出,独自创建了一个后来被称为云计算服务的行业。 AWS 很快就会开始投入大量现金,使亚马逊能够为许多大赌注提供资金。
Back then, Amazon built its own data centers but equipped them with servers and network switches made by other companies. Hamilton spearheaded an effort to replace them with custom hardware, starting with servers. Since Amazon would be buying millions of them, Hamilton reckoned he could lower costs and improve efficiency by tailoring the devices for his growing fleet of data centers and leaving out features that AWS didn’t need.
当时,亚马逊建立了自己的数据中心,但配备了其他公司制造的服务器和网络交换机。汉密尔顿带头努力用定制硬件取代它们,从服务器开始。由于亚马逊将购买数百万台设备,汉密尔顿认为,他可以通过为不断增长的数据中心群定制设备并省略 AWS 不需要的功能来降低成本并提高效率。
The effort was successful enough that Jassy — then running AWS — asked what else the company might design in-house. Hamilton suggested chips, which were gobbling up more and more tasks that had previously been handled by other components. He also recommended that Amazon use the energy-efficient Arm architecture that powers smartphones, a bet that the technology’s ubiquity, and developers’ growing familiarity with it, could help Amazon displace the Intel chips that had long powered server rooms around the world.
这项工作非常成功,当时负责 AWS 运营的 Jassy 询问该公司还可以在内部设计什么。汉密尔顿建议使用芯片,因为芯片正在吞噬越来越多以前由其他组件处理的任务。他还建议亚马逊使用为智能手机提供动力的节能 Arm 架构,并押注该技术的普及以及开发人员对其日益熟悉,可以帮助亚马逊取代长期以来为全球服务器机房提供动力的英特尔芯片。
“All paths lead to us having a semiconductor design team,” he wrote in a proposal presented to Bezos in August 2013. A month later, Hamilton, who likes to hang out with startups and customers in the late afternoon, met Nafea Bshara for a drink at Seattle’s Virginia Inn pub.
他在 2013 年 8 月提交给贝索斯的提案中写道:“所有的道路都让我们拥有一个半导体设计团队。”一个月后,喜欢在下午晚些时候与初创公司和客户闲逛的汉密尔顿与 Nafea Bshara 会面,进行了一次会谈。在西雅图的Virginia Inn酒吧喝酒。
An Israeli chip industry veteran who relocated to the San Francisco Bay area in the early 2000s, Bshara co-founded Annapurna Labs, which he named for the Nepalese peak. (Bshara and a co-founder had intended to summit the mountain before founding the startup. But investors were eager for them to get to work, and they never made the trip.)
Bshara 是一位以色列芯片行业资深人士,于 2000 年代初搬到旧金山湾区,与他人共同创立了 Annapurna Labs,并以尼泊尔山峰命名。 (Bshara 和一位联合创始人在创立这家初创公司之前就打算登顶这座山。但投资者渴望他们开始工作,但他们从未成行。)
The stealthy startup set out to build chips for data centers at a time when most of the industry was fixated on mobile phones. Amazon commissioned processors from Annapurna and, two years later, acquired the startup for a reported $350 million. It was a prescient move.
当大多数行业都专注于移动电话时,这家隐秘的初创公司开始为数据中心制造芯片。亚马逊委托安纳布尔纳峰的处理器,并在两年后以据称 3.5 亿美元的价格收购了这家初创公司。这是一个有先见之明的举动。
一块电路板。摄影师:Sergio Flores/彭博社
Bshara and Hamilton started small, a reflection of their shared appreciation for utilitarian engineering. Back then, each data center server reserved a portion of its horsepower to run control, security and networking features. Annapurna and Amazon engineers developed a card, called Nitro, that vacuumed those functions off the server entirely, giving customers access to its full power.
Bshara 和 Hamilton 从小规模做起,这反映了他们对功利工程的共同欣赏。当时,每台数据中心服务器都会保留一部分马力来运行控制、安全和网络功能。 Annapurna 和亚马逊工程师开发了一种名为 Nitro 的卡,它可以完全从服务器上清除这些功能,让客户能够充分利用其功能。
Later, Annapurna brought Hamilton’s Arm general-purpose processor to life. Called Graviton, the product operated more cheaply than rival Intel gear and made Amazon one of the 10 biggest customers of Taiwan Semiconductor Manufacturing Co., the titan that produces chips for much of the industry.
后来,Annapurna 将 Hamilton 的 Arm 通用处理器带入了生活。该产品名为 Graviton,其运行成本比竞争对手英特尔的设备更便宜,并使亚马逊成为台积电的 10 大客户之一,台积电是为该行业的大部分产品生产芯片的巨头。
Amazon brass had by then grown confident Annapurna could excel even in unfamiliar areas. “You’ll find a lot of companies are very good in CPU, or very good in networking,” Bshara says. “It’s very rare to find the teams that are good in two or three or four different domains.”
亚马逊高层当时已经相信,即使在不熟悉的领域,安纳布尔纳峰也能表现出色。 “你会发现很多公司在 CPU 方面非常出色,或者在网络方面非常出色,”Bshara 说道。 “很难找到在两个、三个或四个不同领域表现出色的团队。”
While Graviton was in development, Jassy asked Hamilton what other things Amazon might make itself. In late 2016, Annapurna deputized four engineers to explore making a machine learning chip. It was another timely bet: A few months later, a group of Google researchers published a seminal paper proposing a process that would make generative AI possible.
在 Graviton 开发过程中,贾西问汉密尔顿,亚马逊还可以自己制造什么东西。 2016年底,安纳普尔纳公司委托四名工程师探索制造机器学习芯片。这是另一个及时的赌注:几个月后,谷歌的一组研究人员发表了一篇开创性的论文,提出了一个使生成式人工智能成为可能的过程。
The paper, titled “Attention is All You Need,” introduced transformers, a software design principle that helps artificial intelligence systems identify the most important pieces of training data. It became the foundational method behind systems that can make educated guesses at the relationships between words and create text from scratch.
这篇题为“注意力就是你所需要的”的论文介绍了 Transformer,这是一种软件设计原理,可以帮助人工智能系统识别最重要的训练数据。它成为系统背后的基本方法,可以对单词之间的关系进行有根据的猜测并从头开始创建文本。
At about this time, Rami Sinno was working for Arm Holdings Plc in Austin and coaching his school-age son through a robotics competition. The team built an app that used machine learning algorithms to pore over photos and detect the algae blooms that periodically foul Austin’s lakes in the summer. Impressed by what kids could do with little more than a laptop, Sinno realized a revolution was coming. He joined Amazon in 2019 to help lead its AI chipmaking efforts.
大约在这个时候,Rami Sinno 正在奥斯汀的 Arm Holdings Plc 工作,并指导他学龄的儿子参加机器人比赛。该团队开发了一款应用程序,使用机器学习算法来仔细研究照片并检测夏季定期污染奥斯汀湖泊的藻类繁殖情况。 Sinno 对孩子们只需要一台笔记本电脑就可以做的事情印象深刻,他意识到一场革命即将到来。他于 2019 年加入亚马逊,帮助领导其人工智能芯片制造工作。
The unit’s first chip was designed to power something called inference — when computers trained to recognize patterns in data make a prediction, such as whether a piece of email is spam. That component, called Inferentia, rolled out to Amazon’s data centers by December 2019, and was later used to help the Alexa voice assistant answer commands. Amazon’s second AI chip, Trainium1, was aimed at companies looking to train machine learning models. Engineers also repackaged the chip with components that made it a better fit for inference, as Inferentia2.
该装置的第一款芯片旨在为推理提供动力——经过训练的计算机可以识别数据模式,从而做出预测,例如一封电子邮件是否是垃圾邮件。该组件名为 Inferentia,于 2019 年 12 月在亚马逊数据中心推出,后来用于帮助 Alexa 语音助手应答命令。亚马逊的第二款人工智能芯片 Trainium1 面向希望训练机器学习模型的公司。工程师还用组件重新封装了芯片,使其更适合推理,即 Inferentia2。
Demand for Amazon’s AI chips was slow at first, meaning customers could get access to them immediately rather than waiting weeks for big batches of Nvidia hardware. Japanese firms looking to quickly join the generative AI revolution took advantage of the situation. Electronics maker Ricoh Co., for example, got help converting large language models trained on English-language data to Japanese.
起初,对亚马逊人工智能芯片的需求缓慢,这意味着客户可以立即获得这些芯片,而不必等待数周才能获得大批量的英伟达硬件。希望快速加入生成人工智能革命的日本公司利用了这一形势。例如,电子产品制造商理光公司获得帮助,将用英语数据训练的大型语言模型转换为日语。
Demand has since picked up, according to Gadi Hutt, an early Annapurna employee who works with companies using Amazon chips. “I don’t have any excess capacity of Trainium sitting around waiting for customers,” he says. “It’s all being used.”
安纳布尔纳峰早期员工、与使用亚马逊芯片的公司合作的加迪·赫特 (Gadi Hutt) 表示,此后需求有所回升。 “我没有多余的 Trainium 容量来等待顾客,”他说。 “都被利用了。”
Trainium2 is the company’s third generation of artificial intelligence chip. By industry reckoning, this is a make-or-break moment. Either the third attempt sells in sufficient volume to make the investment worthwhile, or it flops and the company finds a new path. “I have literally never seen a product deviate from the three-generation rule,” says Naveen Rao, a chip industry veteran who oversees AI work at Databricks Inc., a purveyor of data and analytics software.
Trainium2是该公司的第三代人工智能芯片。根据行业的判断,这是一个决定成败的时刻。第三次尝试要么销量足够大,使投资有价值,要么失败,公司找到了新的道路。 “我确实从未见过任何产品偏离三代规则,”负责数据和分析软件供应商 Databricks Inc. 人工智能工作的芯片行业资深人士 Naveen Rao 说道。
Databricks in October agreed to use Trainium as part of a broad agreement with AWS. At the moment, the company’s AI tools primarily run on Nvidia. The plan is to displace some of that work with Trainium, which Amazon has said can offer 30% better performance for the price, according to Rao. “It comes down to sheer economics and availability,” Rao says. “That’s where the battleground is.”
作为与 AWS 达成的广泛协议的一部分,Databricks 于 10 月同意使用 Trainium。目前,该公司的人工智能工具主要在 Nvidia 上运行。 Rao 表示,该计划是用 Trainium 取代部分工作,亚马逊表示,Trainium 的价格可以提供 30% 的性能提升。 “这取决于纯粹的经济性和可用性,”拉奥说。 “那就是战场。”
Trainium1 was comprised of eight chips, nestled side by side in a deep steel box that allows plenty of room for their heat to dissipate. The full package that AWS rents to its customers is made up of two of these arrays. Each case is filled with wires, neatly enclosed in mesh wrapping.
Trainium1 由八个芯片组成,并排放置在一个深钢盒中,为它们提供足够的散热空间。 AWS 租给客户的完整套餐由其中两个阵列组成。每个箱子里都装满了电线,整齐地包裹在网状包装中。
一排人工智能芯片。摄影师:Sergio Flores/彭博社
For Trainium2, which Amazon says has four times the performance and three times the memory of the prior generation, engineers scrapped most of the cables, routing electrical signals instead via printed circuit boards. And Amazon cut the number of chips per box down to two, so that engineers performing maintenance on one unit take down fewer other components. Sinno has come to think of the data center as a giant computer, an approach Nvidia boss Jensen Huang has encouraged the rest of the industry to adopt. “Simplification is critical there, and it also allowed us to go faster for sure,” Sinno says.
亚马逊表示,Trainium2 的性能是上一代的四倍,内存是上一代的三倍,工程师们废弃了大部分电缆,转而通过印刷电路板路由电信号。亚马逊将每个盒子的芯片数量减少到两个,以便工程师在一台设备上进行维护时拆除更少的其他组件。 Sinno 已经将数据中心视为一台巨型计算机,Nvidia 老板 Jensen Huang 鼓励业界其他公司采用这种方法。 Sinno 说:“简化在这里至关重要,而且它也确实让我们能够走得更快。”
Amazon didn’t wait for TSMC to produce a working version of Trainium2 before starting to test how the new design might work. Instead, engineers fixed two prior generation chips onto the board, giving them time to work on the control software and test for electrical interference. It was the semiconductor industry equivalent of building the plane while it’s flying.
亚马逊没有等到台积电生产出 Trainium2 的工作版本,就开始测试新设计的工作原理。相反,工程师将两个上一代芯片安装到板上,让他们有时间开发控制软件并测试电气干扰。在半导体行业中,这相当于在飞行中建造飞机。
Amazon has started shipping Trainium2, which it aims to string together in clusters of up to 100,000 chips, to data centers in Ohio and elsewhere. A broader rollout is coming for Amazon’s main data center hubs.
亚马逊已经开始向俄亥俄州和其他地方的数据中心运送Trainium2,它的目标是将其串成由多达100,000个芯片组成的集群。亚马逊的主要数据中心即将进行更广泛的部署。
负责芯片设计和测试的 Rami Sinno 将数据中心视为一台巨型计算机。摄影师:Sergio Flores/彭博社
The company aims to bring a new chip to market about every 18 months, in part by reducing the number of trips hardware has to make to outside vendors. Across the lab from the drill press sits a set of oscilloscopes Amazon uses to test cards and chips for bum connectors or design flaws. Sinno hints at the work already underway on future editions: In another lab, where earsplitting fans cool test units, four pairs of pipes dangle from the ceiling. They’re capped now but are ready for the day when future AWS chips produce too much heat to be cooled by fans alone.
该公司的目标是大约每 18 个月向市场推出一款新芯片,部分方法是减少硬件与外部供应商之间的往返次数。钻床对面的实验室里有一套示波器,亚马逊用来测试卡和芯片是否存在连接器故障或设计缺陷。 Sinno 暗示了未来版本中已经正在进行的工作:在另一个实验室中,用震耳欲聋的风扇冷却测试单元,四对管道从天花板上垂下来。它们现在已经受到限制,但已经为未来的 AWS 芯片产生太多热量而无法仅靠风扇冷却的那一天做好了准备。
Other companies are pushing the limits, too. Nvidia, which has characterized demand for its chips as “insane,” is pushing to bring a new chip to market every year, a cadence that caused production issues with its upcoming Blackwell product but will put more pressure on the rest of the industry to keep up. Meanwhile, Amazon’s two biggest cloud rivals are accelerating their own chip initiatives.
其他公司也在挑战极限。英伟达将其芯片的需求描述为“疯狂”,每年都在推动将一款新芯片推向市场,这种节奏导致其即将推出的 Blackwell 产品出现生产问题,但也将给该行业的其他公司带来更大的压力,以保持其市场份额。与此同时,亚马逊最大的两个云竞争对手正在加速自己的芯片计划。
Google began building an AI chip about 10 years ago to speed up the machine learning work behind its search products. Later on, the company offered the product to cloud customers, including AI startups like Anthropic, Cohere and Midjourney. The latest edition of the chip is expected to be widely available next year. In April, Google introduced its first central processing unit, a product similar to Amazon’s Graviton. “General purpose compute is a really big opportunity,” says Amin Vahdat, a Google vice president who leads engineering teams working on chips and other infrastructure. The ultimate aim, he says, is getting the AI and general computing chips working together seamlessly.
谷歌大约 10 年前开始构建人工智能芯片,以加速其搜索产品背后的机器学习工作。后来,该公司向云客户提供了该产品,包括 Anthropic、Cohere 和 Midjourney 等人工智能初创公司。该芯片的最新版本预计将于明年广泛上市。 4 月,谷歌推出了其第一个中央处理器,该产品类似于亚马逊的 Graviton。 “通用计算确实是一个巨大的机会,”领导芯片和其他基础设施工程团队的谷歌副总裁 Amin Vahdat 表示。他说,最终目标是让人工智能和通用计算芯片无缝协作。
指定汉密尔顿为亚马逊拥有的多项专利的拼图。摄影师:Chona Kasinger/彭博社
Microsoft got into the data center chip game later than AWS and Google, announcing an AI accelerator called Maia and a CPU named Cobalt only late last year. Like Amazon, the company had realized it could offer customers better performance with hardware tailored to its data centers.
微软比 AWS 和谷歌更晚进入数据中心芯片领域,去年年底才发布了一款名为 Maia 的人工智能加速器和一款名为 Cobalt 的 CPU。与亚马逊一样,该公司也意识到可以通过为其数据中心量身定制的硬件为客户提供更好的性能。
Rani Borkar, a vice president who spent almost three decades at Intel, leads the effort. Earlier this month, her team added two products to Microsoft’s portfolio: a security chip and a data processing unit that speeds up the flow of data between CPUs and graphics processing units, or GPUs. Nvidia sells a similar product. Microsoft has been testing the AI chip internally and just started using it alongside its fleet of Nvidia chips to run the service that lets customers create applications with OpenAI models.
在英特尔工作了近三十年的副总裁拉尼·博卡尔 (Rani Borkar) 领导了这项工作。本月早些时候,她的团队在微软的产品组合中添加了两款产品:一款安全芯片和一款数据处理单元,可加速 CPU 和图形处理单元 (GPU) 之间的数据流。 Nvidia 也销售类似的产品。微软一直在内部测试这款 AI 芯片,并刚刚开始将其与 Nvidia 芯片组一起使用来运行允许客户使用 OpenAI 模型创建应用程序的服务。
While Microsoft’s efforts are considered a couple of generations behind Amazon’s, Borkar says the company is happy with the results so far and is working on updated versions of its chips. “It doesn’t matter where people started,” she says. “My focus is all about: What does the customer need? Because you could be ahead, but if you are building the wrong product that the customer doesn’t want, then the investments in silicon are so massive that I wouldn’t want to be a chapter in that book.”
虽然微软的努力被认为落后亚马逊几代,但博卡表示,该公司对迄今为止的结果感到满意,并正在开发其芯片的更新版本。 “人们从哪里开始并不重要,”她说。 “我的重点是:客户需要什么?因为你可能会领先,但如果你正在构建客户不想要的错误产品,那么对芯片的投资就会如此巨大,以至于我不想成为那本书中的一章。”
Despite their competitive efforts, all three cloud giants sing Nvidia’s praises and jockey for position when new chips, like Blackwell, hit the market.
尽管三家云计算巨头都在努力竞争,但当 Blackwell 等新芯片上市时,这三大云巨头都对 Nvidia 表示赞扬,并争夺自己的地位。
Amazon’s Trainium2 will likely be deemed a success if it can take on more of the company’s internal AI work, along with the occasional project from big AWS customers. That would help free up Amazon’s precious supply of high-end Nvidia chips for specialized AI outfits. For Trainium2 to become an unqualified hit, engineers will have to get the software right — no small feat. Nvidia derives much of its strength from the comprehensiveness of its suite of tools, which let customers get machine-learning projects online with little customization. Amazon’s software, called Neuron SDK, is in its infancy by comparison.
如果亚马逊的 Trainium2 能够承担更多公司内部的人工智能工作,以及来自 AWS 大客户的偶尔项目,那么它很可能会被认为是成功的。这将有助于释放亚马逊为专业人工智能设备提供的宝贵的高端英伟达芯片供应。为了让 Trainium2 成为一款不折不扣的热门产品,工程师们必须正确使用软件——这绝非易事。英伟达的大部分优势来自于其工具套件的全面性,这些工具让客户无需定制即可在线获得机器学习项目。相比之下,亚马逊的软件 Neuron SDK 还处于起步阶段。
Even if companies can port their projects to Amazon without much trouble, checking that the switch-over didn’t break anything can eat up hundreds of hours of engineers’ time, according to an Amazon and chip industry veteran, who requested anonymity to speak freely. An executive at an AWS partner that helps customers with AI projects, who also requested anonymity, says that while Amazon had succeeded in making its general-purpose Graviton chips easy to use, prospective users of the AI hardware still face added complexity.
一位要求匿名的亚马逊和芯片行业资深人士表示,即使公司可以轻松地将他们的项目移植到亚马逊,检查切换是否不会破坏任何东西也可能会占用工程师数百个小时的时间。 。一位为客户提供人工智能项目帮助的 AWS 合作伙伴的一位高管表示,虽然亚马逊已经成功地使其通用 Graviton 芯片变得易于使用,但人工智能硬件的潜在用户仍然面临着更大的复杂性。
“There’s a reason Nvidia dominates,” says Chirag Dekate, a vice president at Gartner Inc. who tracks artificial intelligence technologies. “You don’t have to worry about those details.”
“Nvidia 占据主导地位是有原因的,”跟踪人工智能技术的 Gartner 公司副总裁 Chirag Dekate 说道。 “你不必担心这些细节。”
So Amazon has enlisted help — encouraging big customers and partners to use the chips when they strike up new or renewed deals with AWS. The idea is to get cutting-edge teams to run the silicon ragged and find areas for improvement.
因此,亚马逊寻求帮助——鼓励大客户和合作伙伴在与 AWS 达成新的或续签的交易时使用这些芯片。我们的想法是让最先进的团队运行硅片并找到需要改进的地方。
One of those companies is Databricks, which anticipates spending weeks or months getting things up and running but is willing to put in the effort in the hopes that promised cost savings materialize. Anthropic, the AI startup and OpenAI rival, agreed to use Trainium chips for future development after accepting $4 billion of Amazon’s money last year, though it also uses Nvidia and Google products. On Friday, Anthropic announced another $4 billion infusion from Amazon and deepened the partnership.
Databricks 就是其中之一,该公司预计需要花费数周或数月的时间来启动和运行,但愿意付出努力,希望承诺的成本节约能够实现。人工智能初创公司 Anthropic 是 OpenAI 的竞争对手,去年接受了亚马逊 40 亿美元的资金后,同意使用 Trainium 芯片进行未来开发,尽管它也使用 Nvidia 和谷歌的产品。周五,Anthropic 宣布亚马逊再次注资 40 亿美元,并加深了合作关系。
“We’re particularly impressed by the price-performance of Amazon Trainium chips,” says Tom Brown, Anthropic’s chief compute officer. “We’ve been steadily expanding their use across an increasingly wide range of workloads.”
“Amazon Trainium 芯片的性价比给我们留下了特别深刻的印象,”Anthropic 首席计算官 Tom Brown 说道。 “我们一直在不断扩大其在越来越广泛的工作负载中的使用。”
Hamilton says Anthropic is helping Amazon improve quickly. But he’s clear-eyed about the challenges, saying it’s “mandatory” to create great software that makes it easy for customers to use AWS chips. “If you don’t bridge the complexity gap,” he says, “you’re going to be unsuccessful.”
汉密尔顿表示,Anthropic 正在帮助亚马逊快速改进。但他对挑战很清醒,并表示开发出色的软件以让客户轻松使用 AWS 芯片是“强制性的”。 “如果你不弥合复杂性差距,”他说,“你就不会成功。”
More From Bloomberg 更多来自彭博社
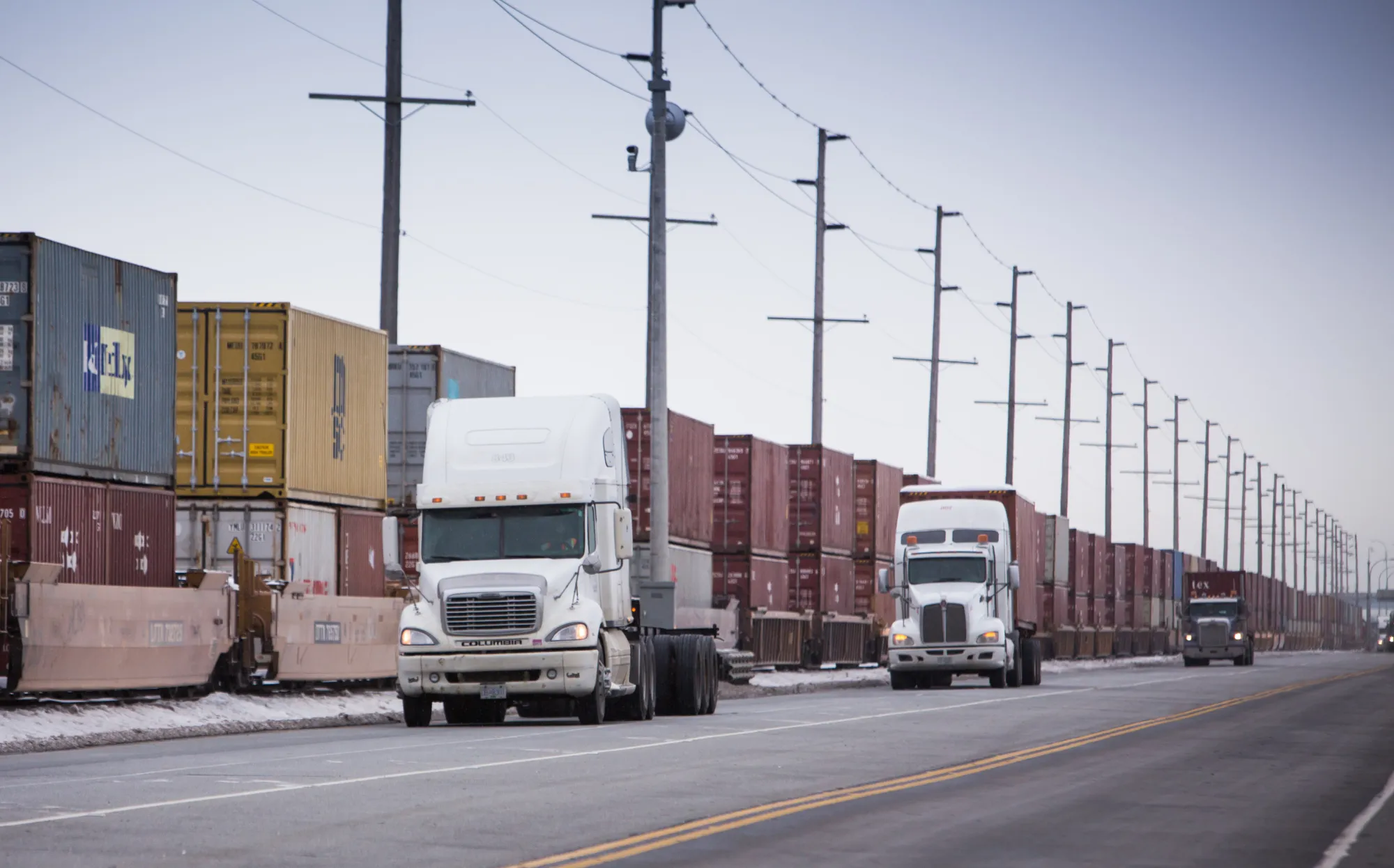
Goldman Sees ‘Significant’ Hit to US From Trump’s Canada Tariffs
高盛认为特朗普的加拿大关税对美国造成“重大”打击
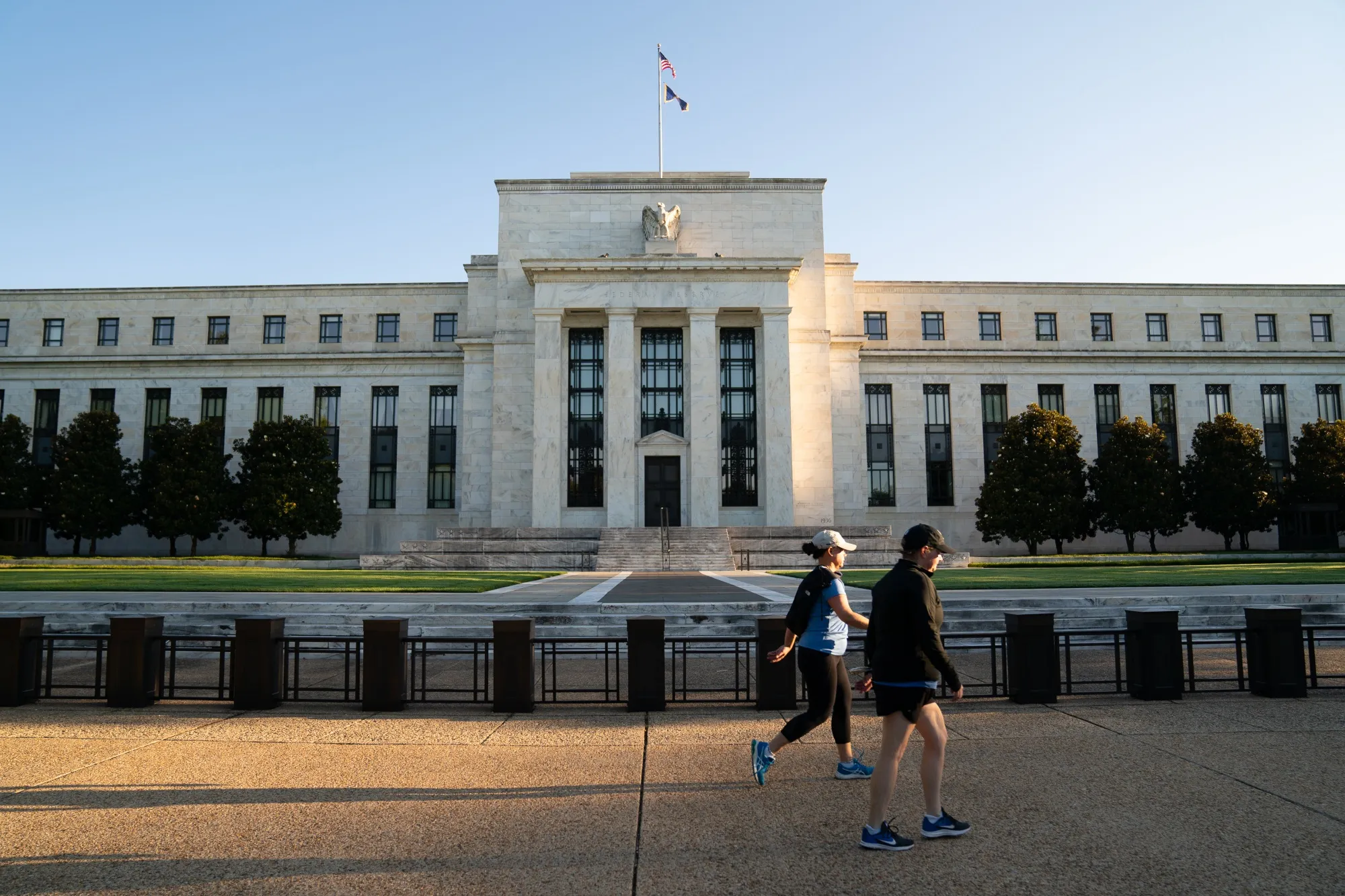
Fed’s Favored Inflation Gauge Picks Up, Backs Cautious Approach
美联储青睐的通胀指标回升,支持采取谨慎态度
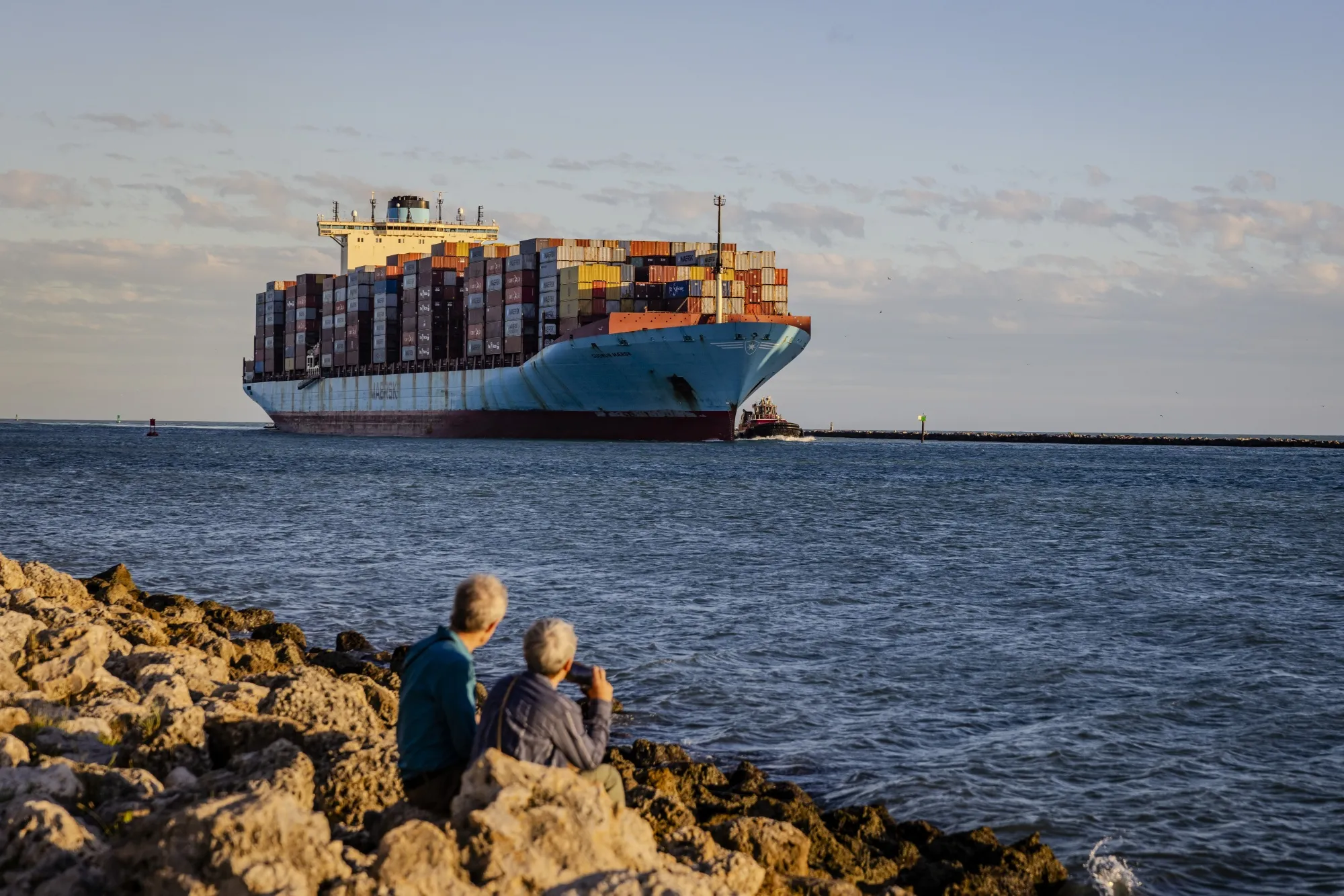
Trump’s Tariff Threat to Top Trading Partners Roils Markets
特朗普对主要贸易伙伴的关税威胁扰乱市场
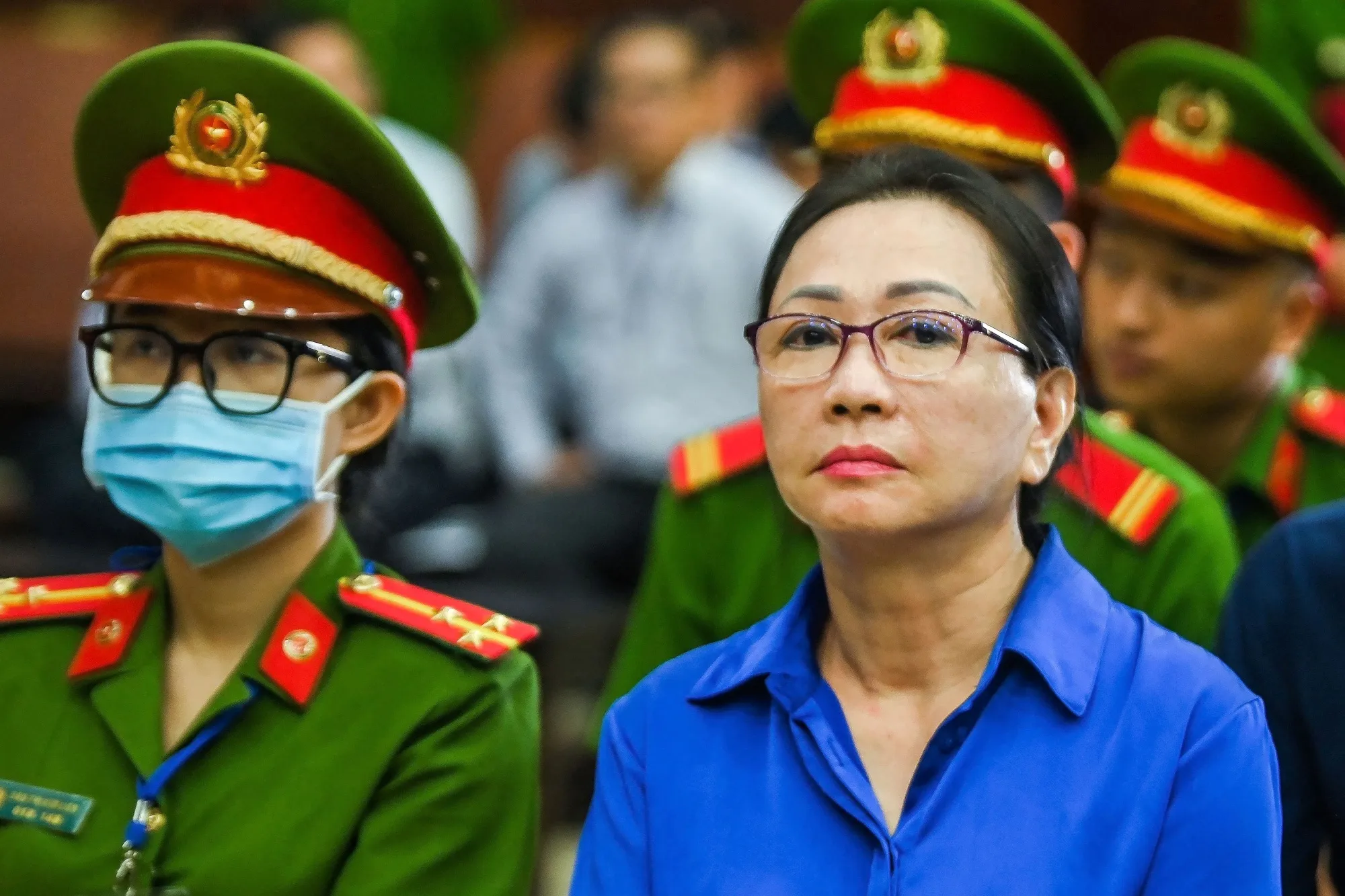
Vietnam Tycoon Told to Repay $11 Billion to Avoid Execution
越南大亨被告知偿还 110 亿美元以避免被处决
Top Reads 热门读物
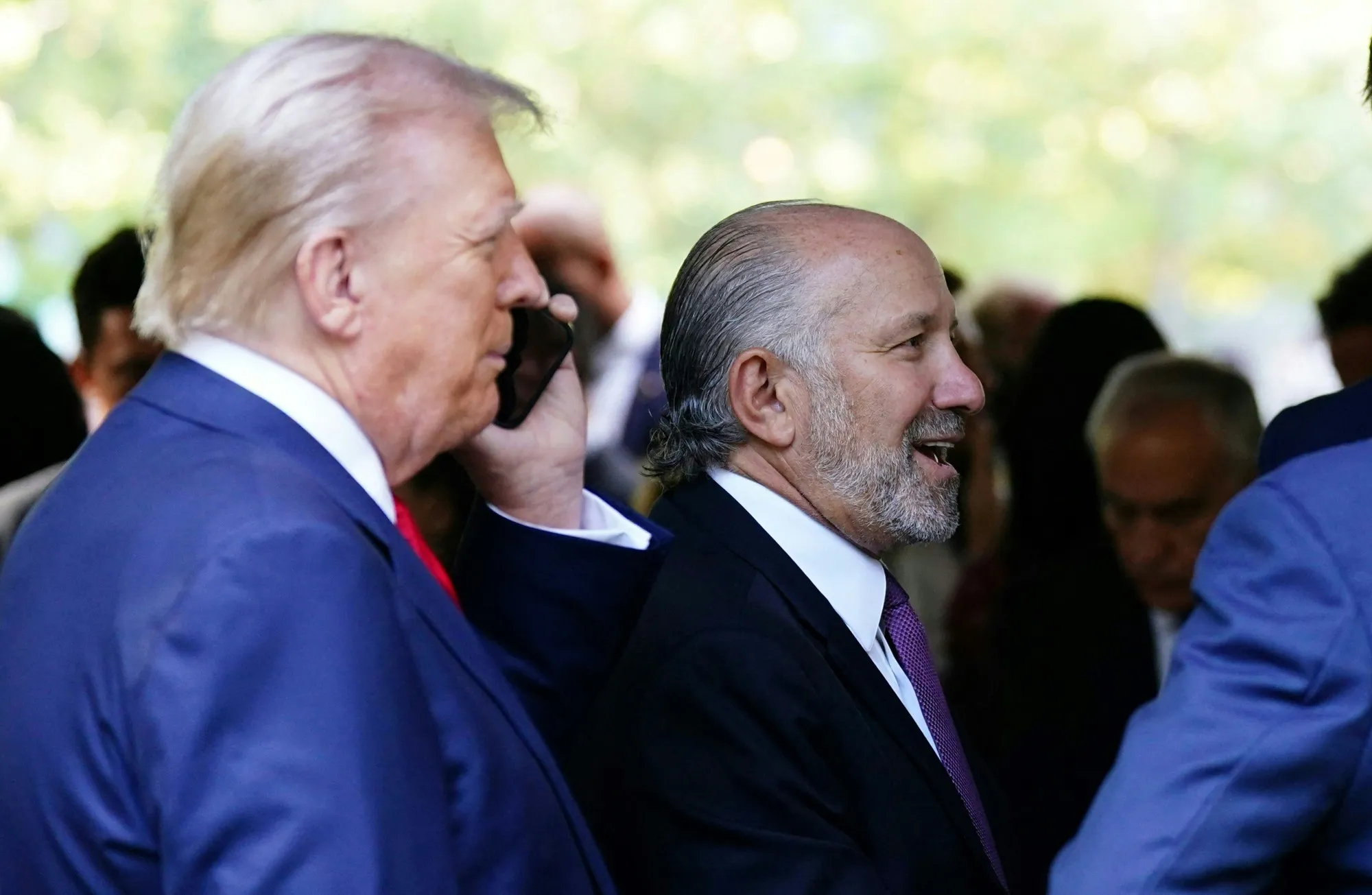
Howard Lutnick, King of Cantor, Gets a Boss of His Own in Donald Trump
坎托之王霍华德·卢特尼克在唐纳德·特朗普身上找到了自己的老板
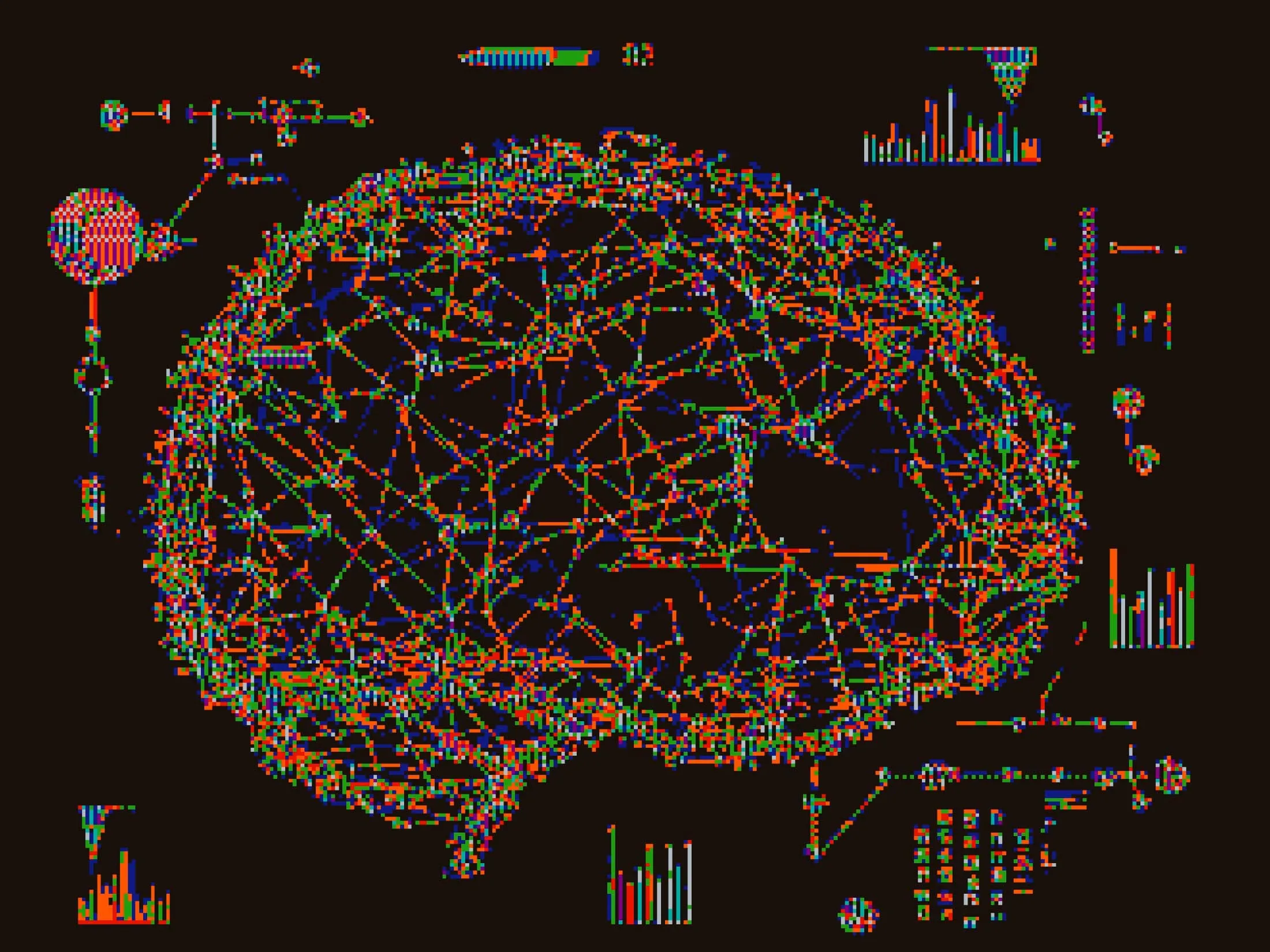
Quant Olympics Seeks to Find Next Generation of Finance Stars
量化奥林匹克寻求寻找下一代金融明星
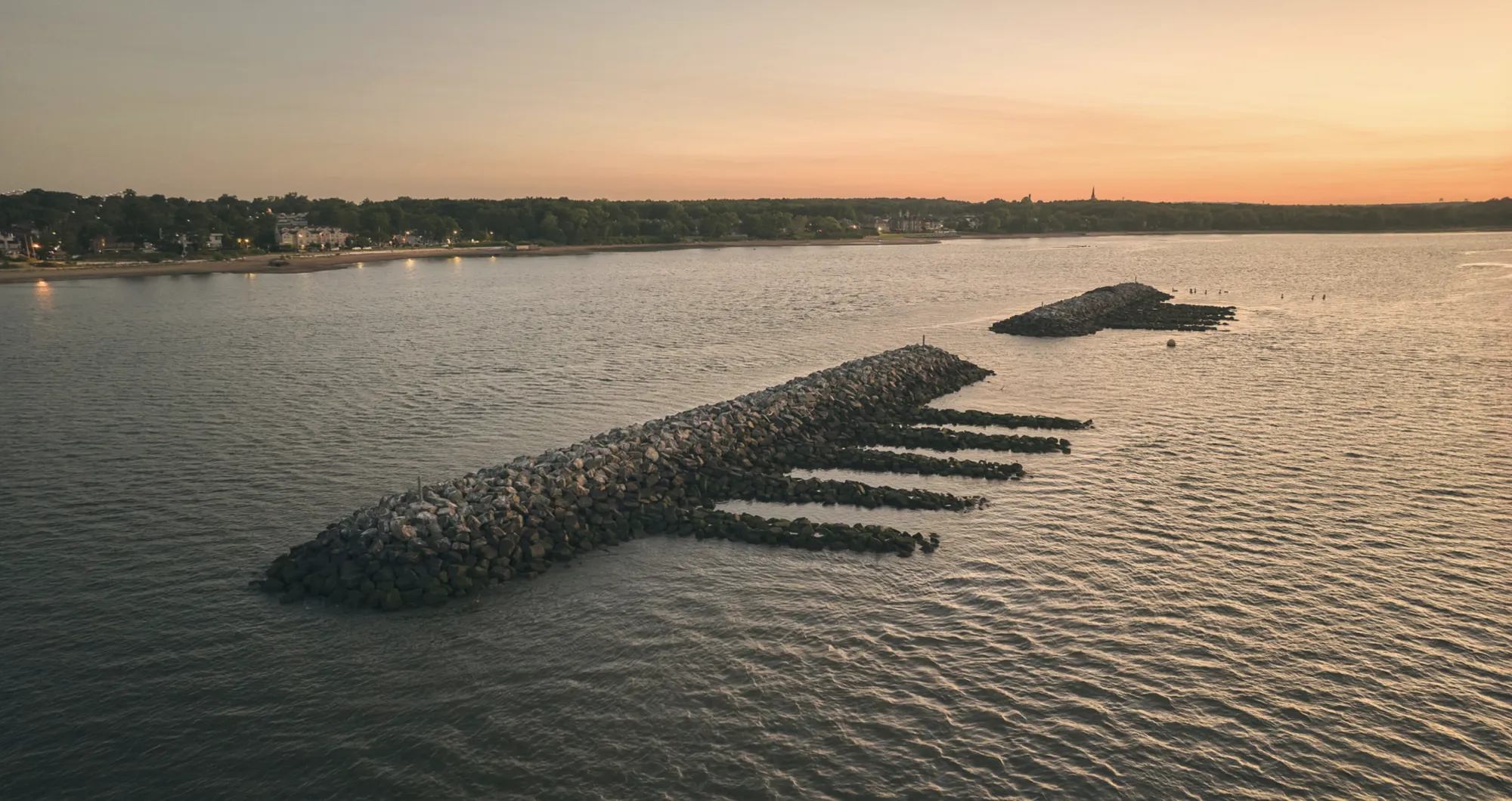
New York City’s ‘Living Breakwaters’ Brace for Stormier Seas
纽约市的“活防波堤”为汹涌的大海做好准备
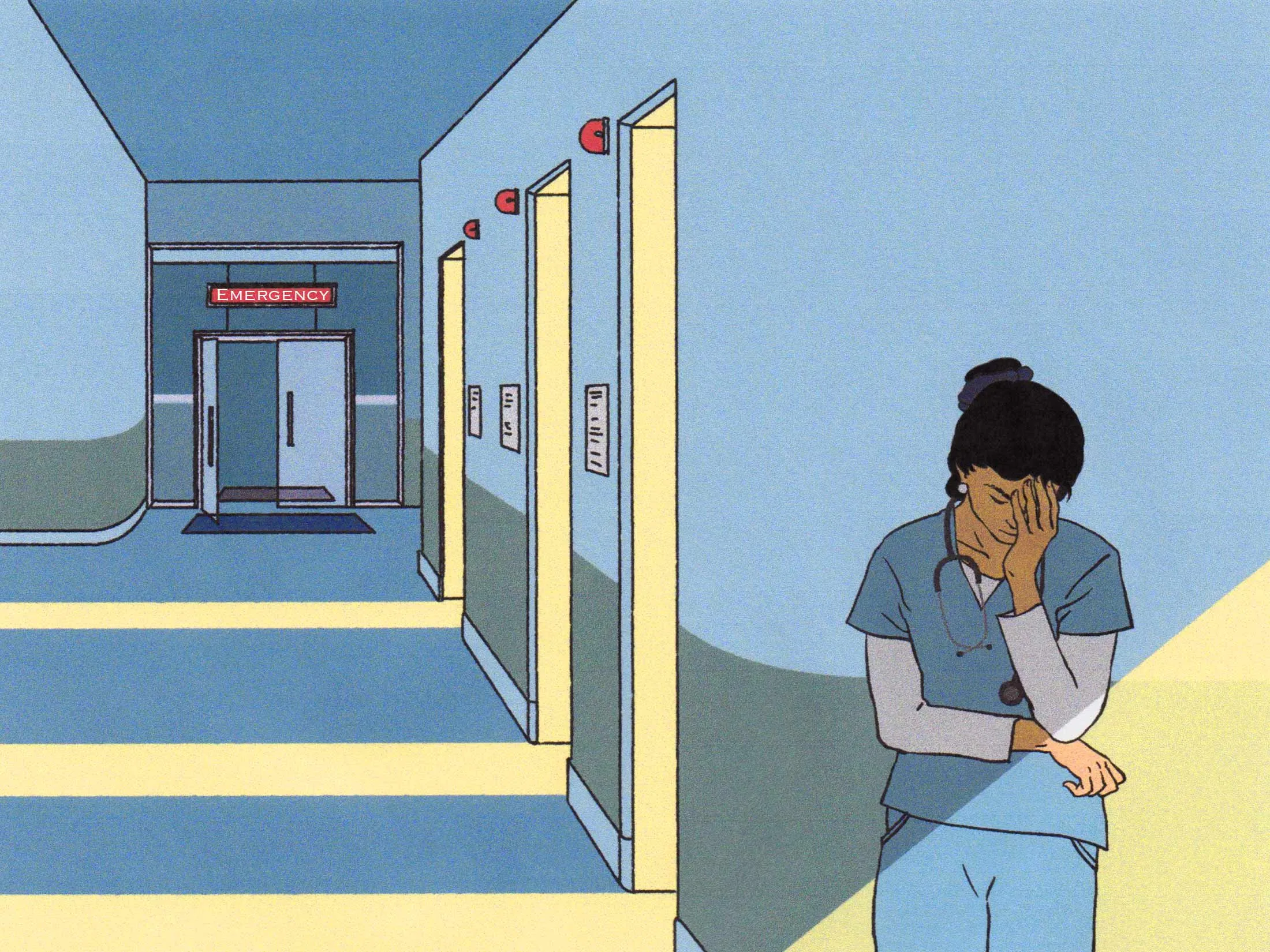
What Happens When US Hospitals Go Big on Nurse Practitioners
当美国医院大量招募执业护士时会发生什么